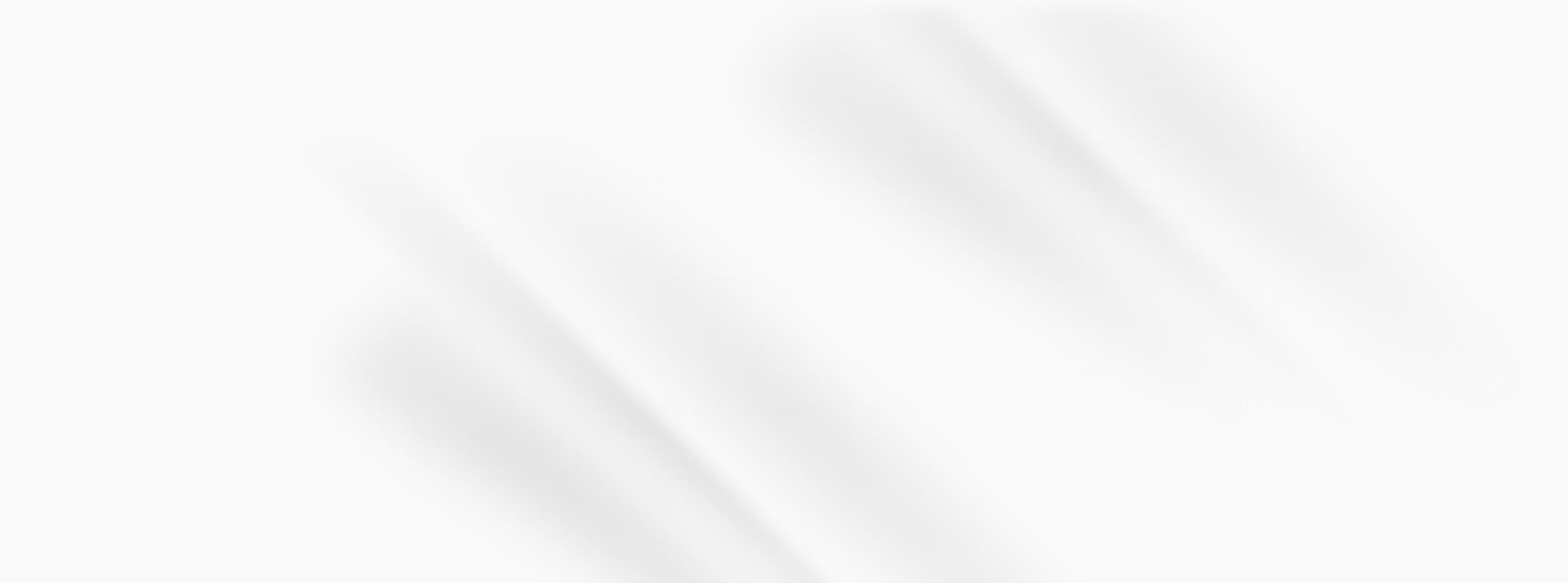
The Ultimate Guide to Bottom-Up Forecasting for B2B Sales Leaders
Feb 17, 2025
Feb 17, 2025
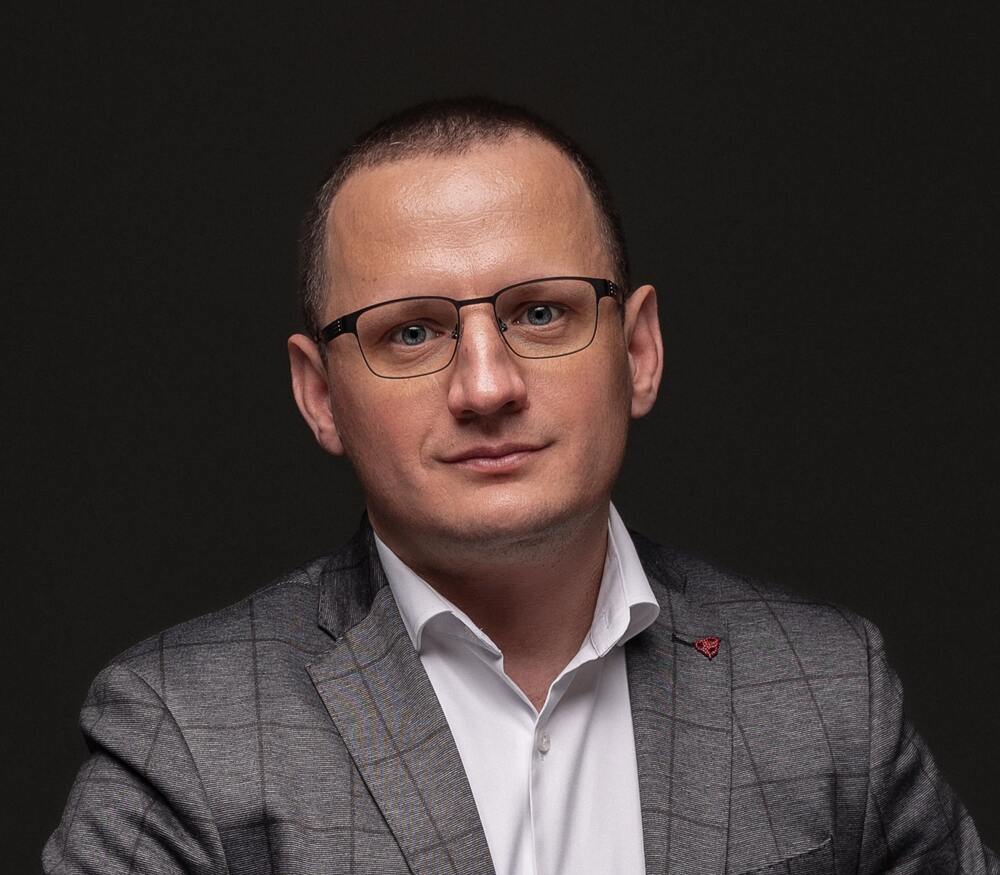
Alex Zlotko
CEO at Forecastio
Last updated
Feb 17, 2025
Reading time
10 min
Share:
Share
Table of Contents
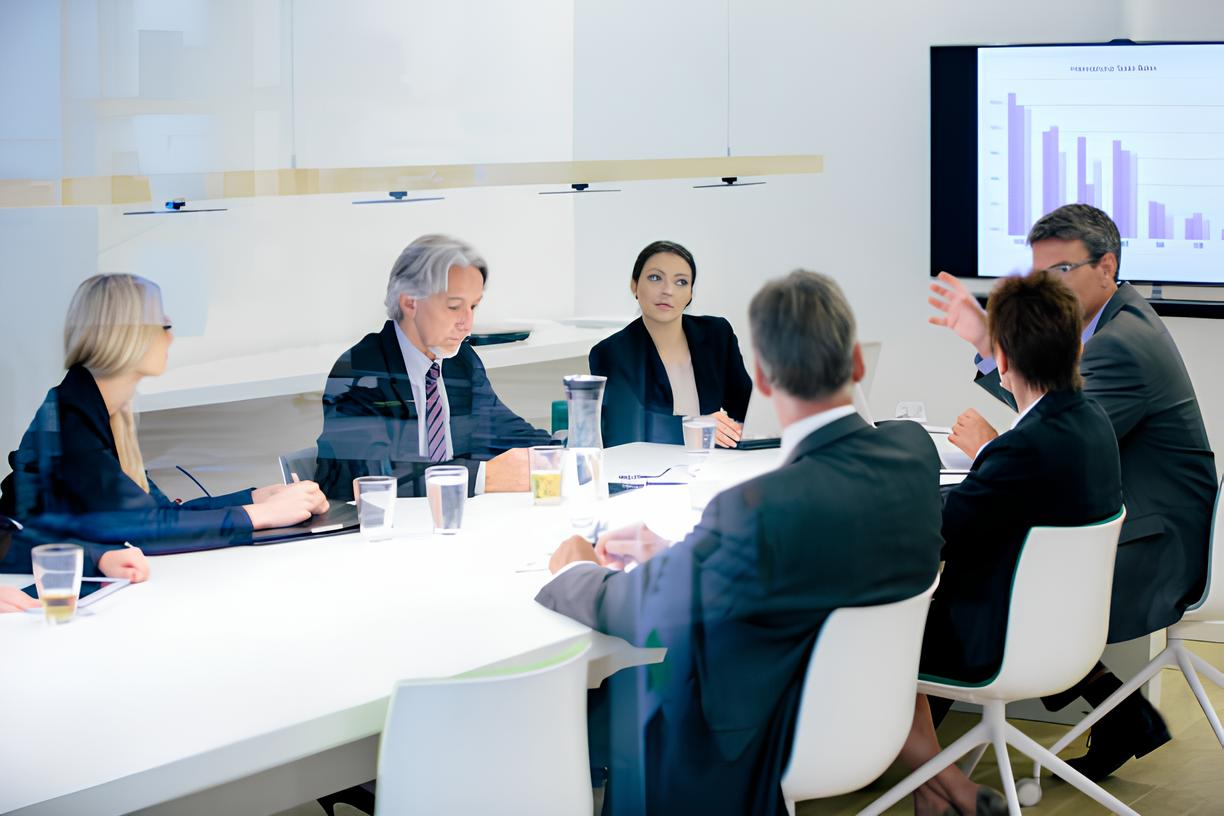
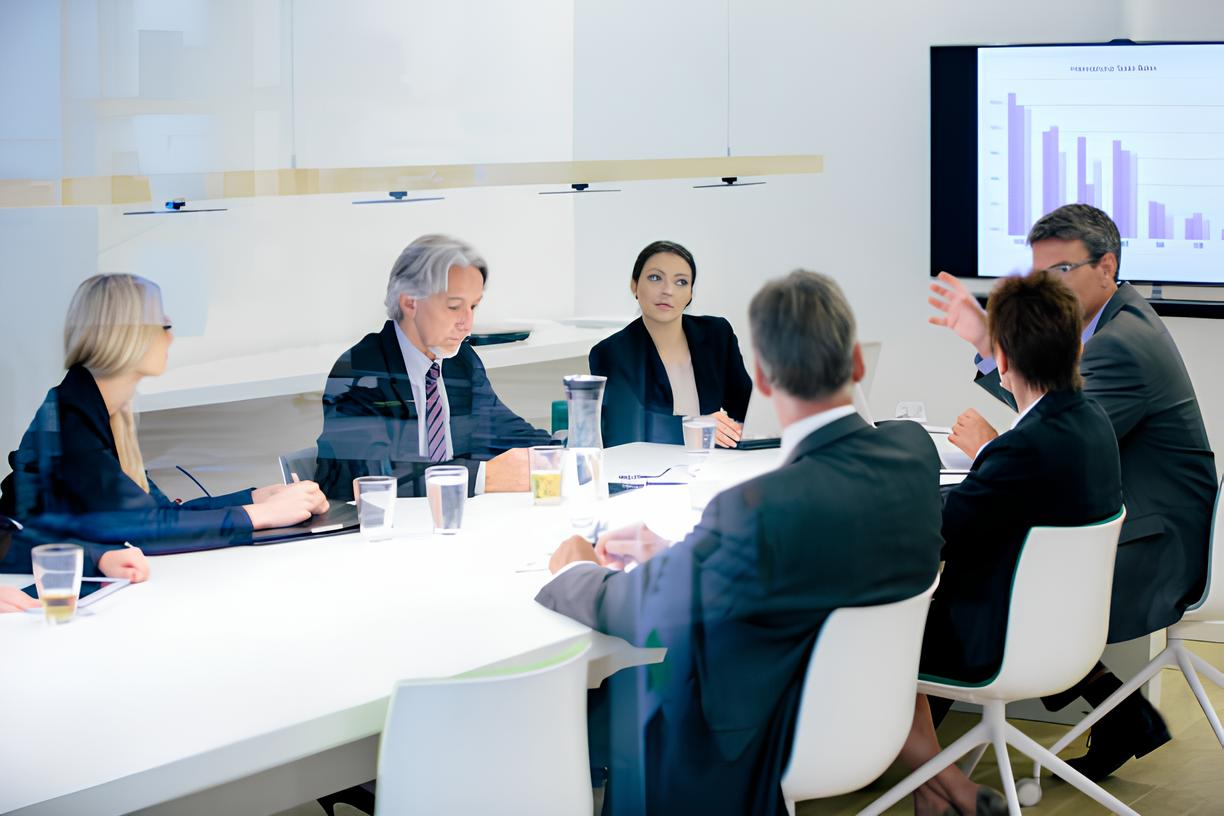
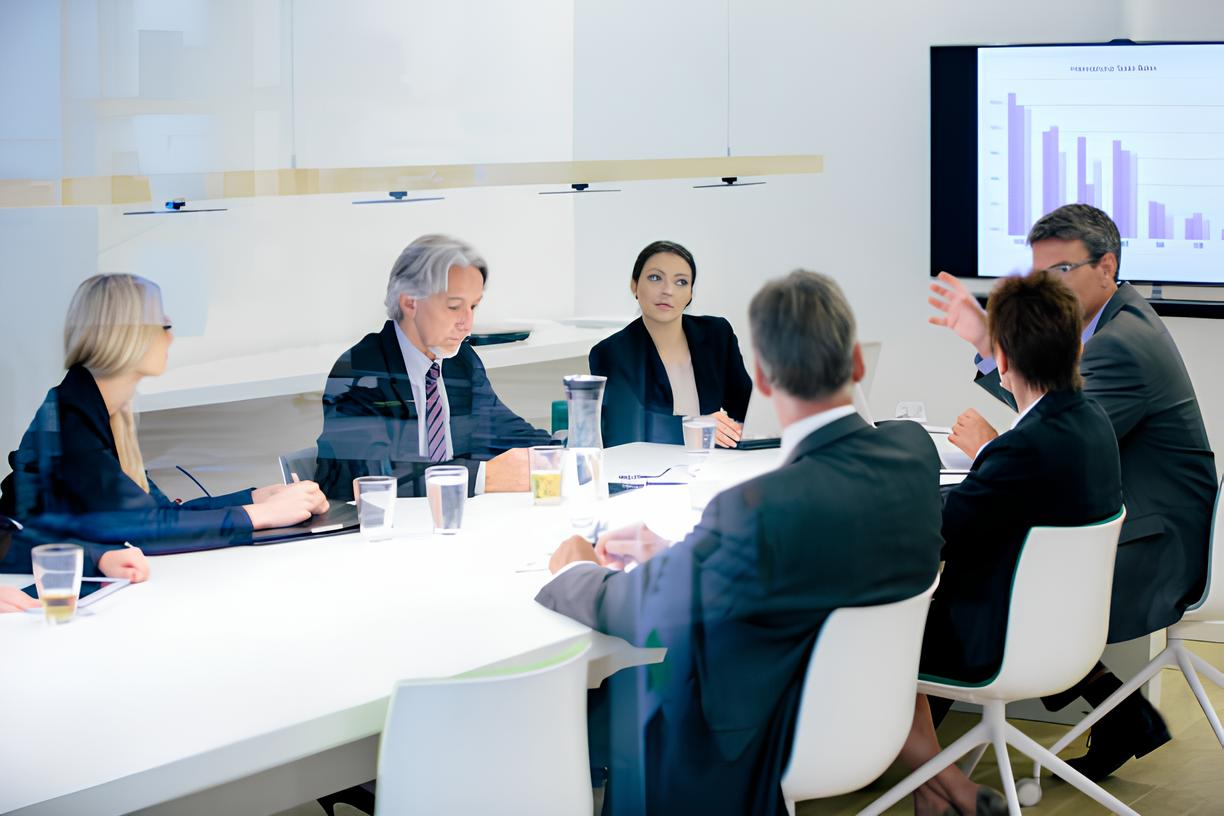
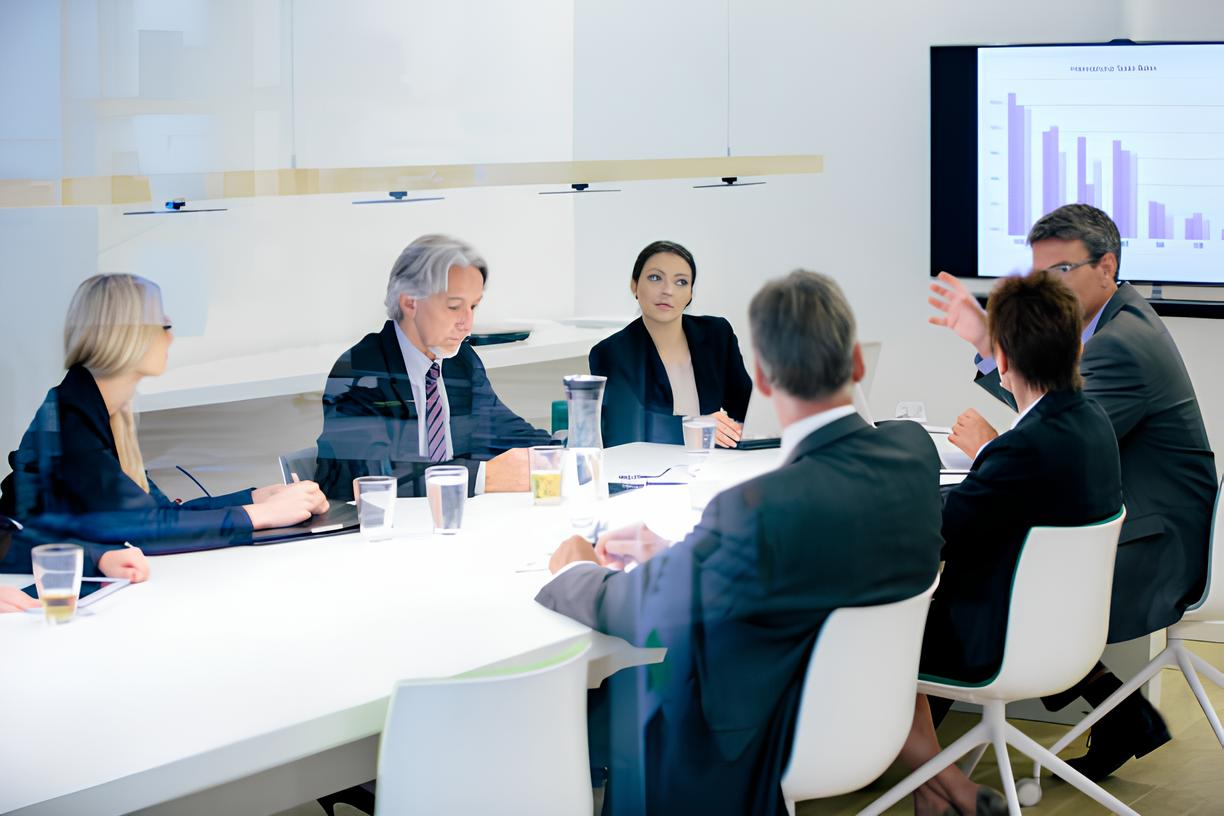
Quick Take
Quick Take
Bottom-up forecasting builds revenue predictions from actual sales data, not market trends, making it more accurate for B2B sales.
Start with individual rep performance: track weekly calls, demos, and closes.
Example: If reps make 50 calls to get 10 demos and close 2 deals at $5,000 each, that's $10,000 per rep weekly. Multiply by your team size for total projections.
Success requires clean CRM data, clear metrics (conversion rates, deal size, sales cycle length), and regular forecast reviews.
Common pitfalls include messy data and skipping reviews. Use AI tools to spot patterns and improve accuracy over time.
Bottom-up forecasting builds revenue predictions from actual sales data, not market trends, making it more accurate for B2B sales.
Start with individual rep performance: track weekly calls, demos, and closes.
Example: If reps make 50 calls to get 10 demos and close 2 deals at $5,000 each, that's $10,000 per rep weekly. Multiply by your team size for total projections.
Success requires clean CRM data, clear metrics (conversion rates, deal size, sales cycle length), and regular forecast reviews.
Common pitfalls include messy data and skipping reviews. Use AI tools to spot patterns and improve accuracy over time.
Introduction
Accurate sales forecasting is one of the most critical aspects of B2B sales management, directly influencing future sales performance, revenue generation, and overall business growth. It enables sales leaders to set achievable revenue projections, allocate resources efficiently, and make data-driven strategic decisions. However, many organizations struggle with forecasting accuracy due to the limitations of traditional forecasting methods.
One commonly used approach is top-down forecasting, which relies heavily on high-level market trends, market research, and broad industry assumptions to estimate total sales. While this method provides a macro-level view, it often lacks precision because it doesn’t consider the detailed data analysis of current sales data, sales channels, or the actual performance of individual sales reps. This is where bottom-up forecasting provides a more reliable alternative.
Unlike the top-down approach, bottom-up forecasting focuses on analyzing historical data, individual sales reps' performance, and specific sales cycles to build a comprehensive and accurate forecast. This bottom-up approach begins at the granular level—using actual sales data from deals, pipelines, and sales teams—before scaling up to company-wide projections. The result is a forecasting process that reflects real, measurable activity and provides a more precise estimation of potential revenue.
In this guide, we will explore bottom-up forecasting in detail, explaining how it differs from top-down forecasting, its methodology, and how to implement it effectively. We will cover essential elements such as the bottom-up forecasting formula, real-world bottom-up forecasting examples, and the advantages and limitations of this bottom-up forecasting method.
Additionally, we will discuss alternative forecasting methods and best practices for improving forecast accuracy in B2B sales teams. Whether you are a sales leader, part of a management team, or a sales rep looking to refine your sales process, this guide will help you master bottom-up sales forecasting for more reliable and data-driven revenue projections.
What is Bottom-Up Forecasting?
Bottom-up forecasting is a sales forecasting method that generates revenue projections by analyzing detailed data at the ground level. Unlike top-down forecasting, which relies on broad market research, high-level assumptions, and macroeconomic indicators, bottom-up forecasting focuses on real, measurable activity within a company’s sales teams. This bottom-up approach starts with individual sales reps, specific deals, or even sales channels, aggregating these insights to create a more accurate forecast of future sales performance and potential revenue.
By leveraging current sales data, ongoing deals in the pipeline, and past sales cycles, companies can develop a highly tailored and precise forecasting process that reflects actual sales productivity. Since this method is rooted in real-time deal activity and historical data, it provides a dynamic, adaptable forecast that adjusts to changing conditions, making it particularly effective for improving forecast accuracy and revenue generation.
Key Characteristics of Bottom-Up Forecasting
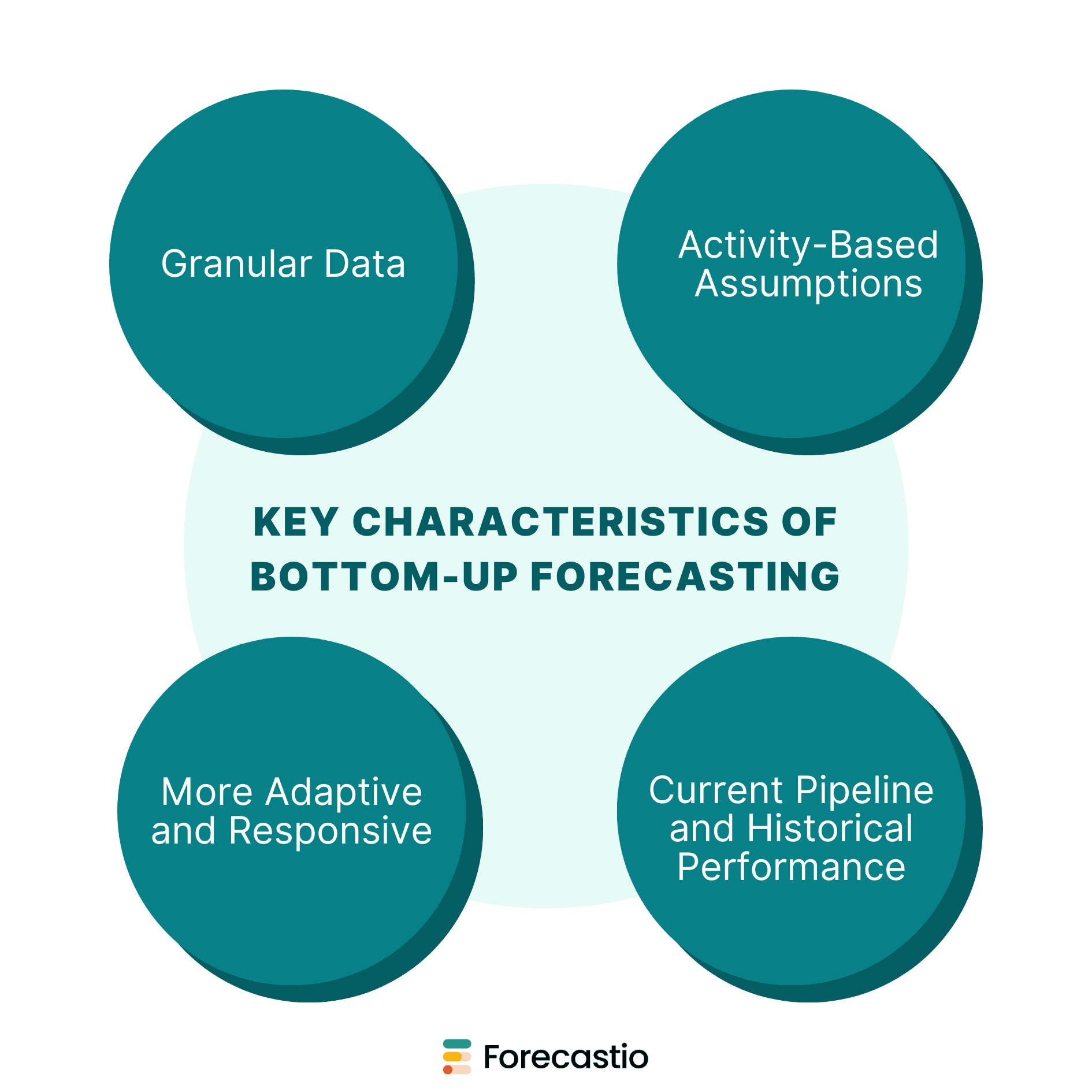
Granular Data: Unlike the top-down approach, which estimates total sales using broad industry figures, bottom-up forecasting relies on detailed data analysis from sales reps' performance, deal pipelines, and sales channels.
Activity-Based Assumptions: A bottom-up forecasting method considers specific sales process activities, such as outbound calls, booked demos, conversion rates, average deal size, and sales velocity, to generate more realistic revenue projections.
Current Pipeline and Historical Performance: A key element of bottom-up sales forecasting is its reliance on both historical data and current sales data from existing deals in the pipeline. By analyzing ongoing sales opportunities, it provides a real-time view of potential revenue, rather than relying solely on past performance.
More Adaptive and Responsive: Since bottom-up models are built on existing market data and current sales data, rather than external assumptions, they are highly adaptable. Companies can quickly adjust their forecasts when market conditions shift, ensuring a more dynamic and responsive forecasting process.
This bottom-up forecasting example demonstrates why many sales leaders prefer this method over top-down forecasting when looking to enhance forecast accuracy, optimize sales productivity, and make better-informed decisions based on real-time sales data.
If you want to get more detailed information about the most effective sales forecasting methods, read this article or download the ebook here.
Bottom-Up vs. Top-Down Forecasting
To understand the advantages of bottom-up forecasting, it’s essential to compare it with top-down forecasting and see how each forecasting method impacts forecast accuracy, adaptability, and data reliability.
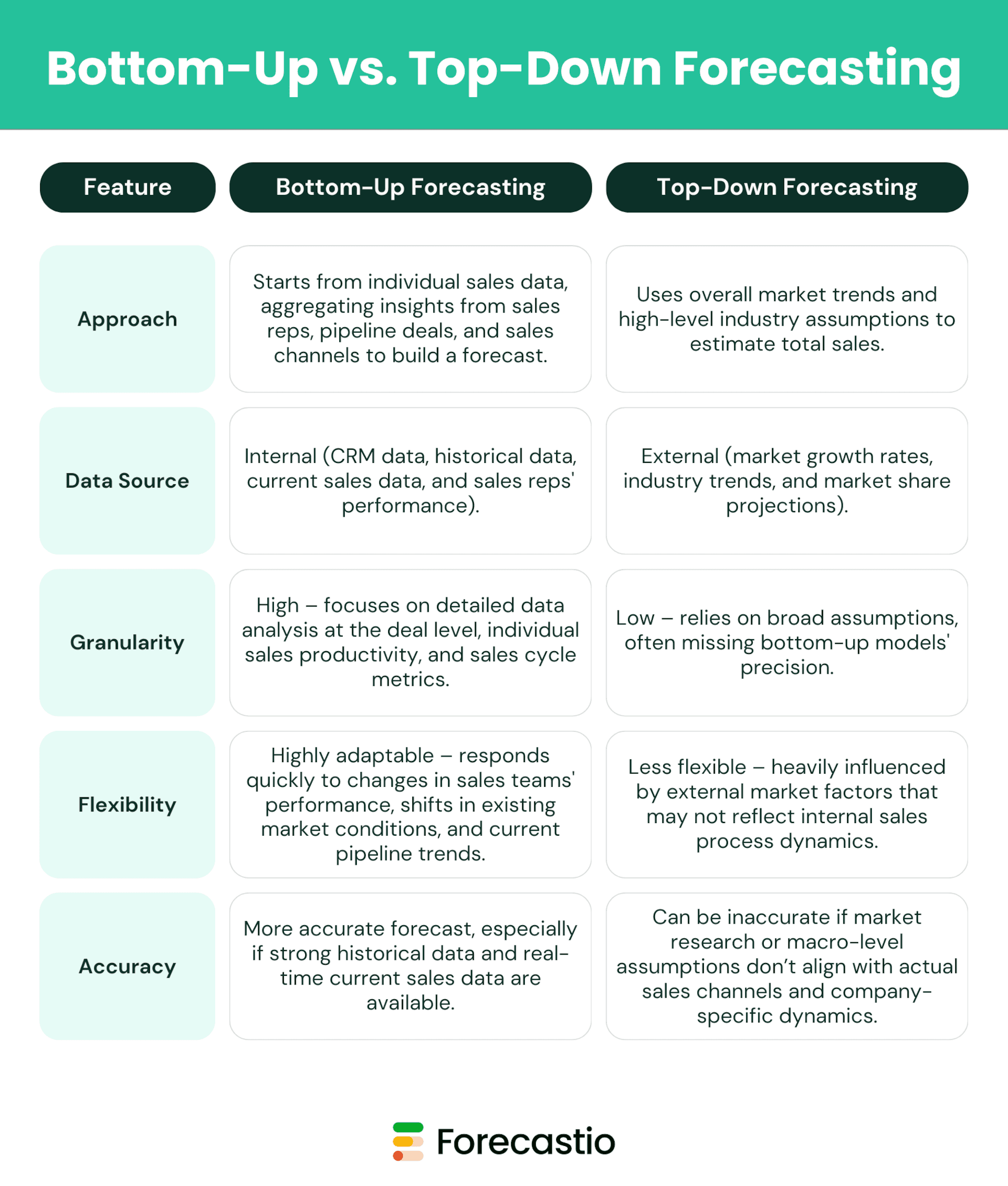
Why Bottom-Up Forecasting Is More Reliable for B2B Sales
For B2B companies, bottom-up forecasting is generally the preferred forecasting process, particularly in dynamic industries where external market share trends may not directly influence future sales performance. Since bottom-up forecasting focuses on real sales data from active pipeline deals, past sales cycles, and sales reps' productivity, it provides a more accurate forecast than the top-down approach, which can often overlook critical internal factors.
By leveraging bottom-up forecasting formulas and real-time bottom-up sales data, sales leaders can create revenue projections that reflect actual sales performance, leading to more precise planning, better sales productivity, and improved revenue generation.
How Bottom-Up Forecasting Works
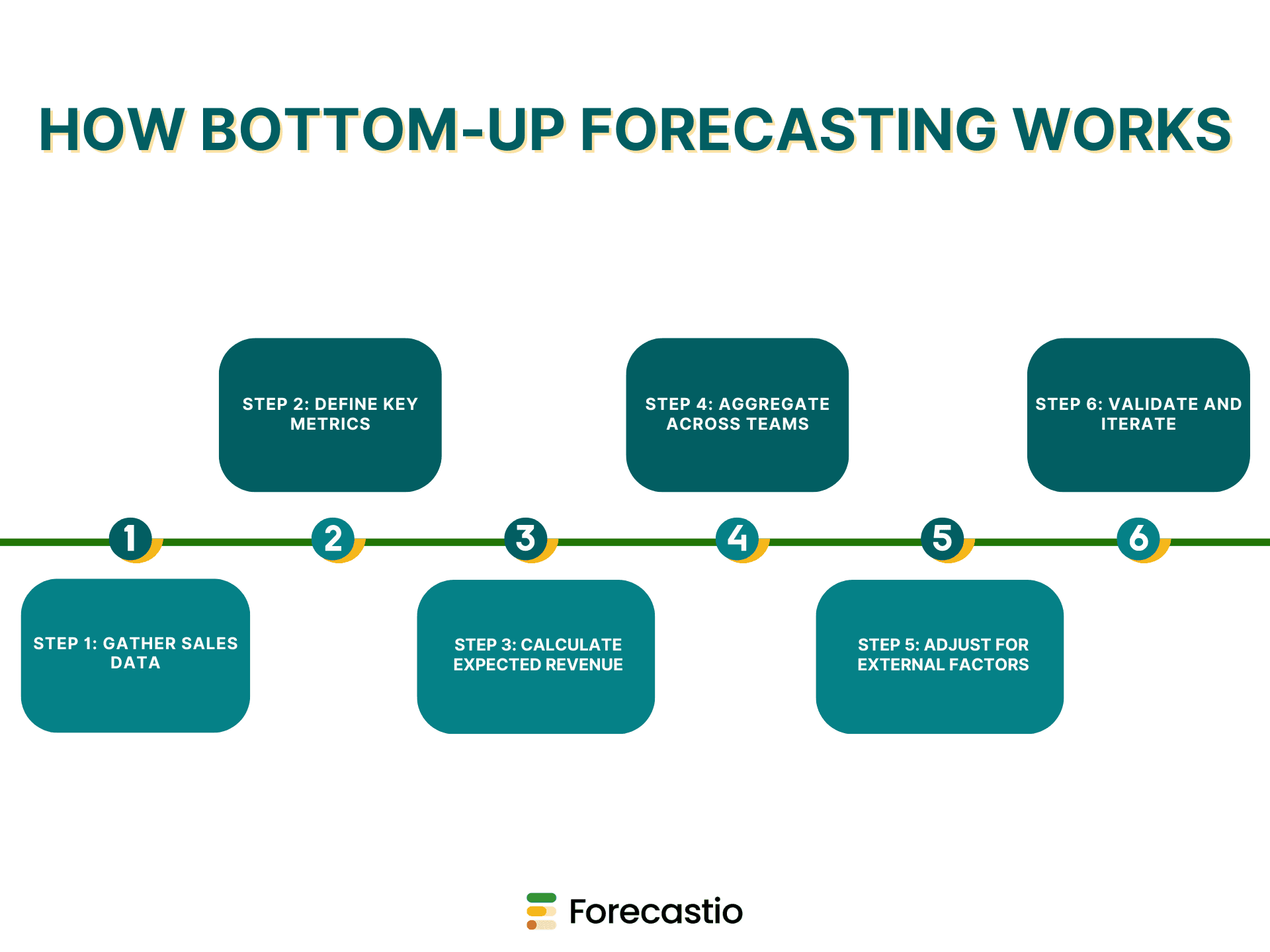
The bottom-up forecasting process follows a structured, data-driven methodology that aggregates insights from individual sales reps, current sales data, and historical data to generate a more accurate forecast. Unlike top-down forecasting, which starts with market research and broad assumptions, bottom-up forecasting focuses on granular, deal-level details to provide reliable revenue projections.
Below is a step-by-step approach to implementing bottom-up sales forecasting effectively:
1. Gather Sales Data
The foundation of bottom-up forecasting is detailed data analysis from sales reps, active deals in the pipeline, and historical data trends. Key data sources include:
Individual rep performance metrics (e.g., number of deals closed, conversion rates, outbound activity, demo-to-close ratio).
Pipeline analysis, including deal velocity, win rates by stage, and overall sales cycle length.
Revenue per account, factoring in past total sales and existing market penetration.
Sales channels and their contribution to revenue generation.
2. Define Key Metrics
To create an accurate forecast, specific sales performance indicators need to be established:
Number of sales reps actively closing deals.
Average quota attainment per rep.
Average deal size based on historical data and current sales data.
Sales cycle length, determining how long it takes for deals to close.
Win rate per stage, helping refine the bottom-up forecasting formula.
3. Calculate Expected Revenue
Once key metrics are identified, the next step is estimating potential revenue:
Determine the number of deals expected to close using historical performance and current pipeline data.
Assign deal probabilities based on pipeline stage (e.g., a deal in the proposal stage might have a 50% chance of closing, while a deal in negotiation might have an 80% probability).
Factor in sales reps’ commitments, as reps often provide their own estimates on which deals they believe will close.
Multiply the adjusted expected deal count by the average deal size to generate preliminary revenue projections.
Adjust calculations based on seasonality, pipeline trends, and variations in sales productivity.
4. Aggregate Across Teams
To transition from individual forecasts to an organizational sales forecast, data is rolled up across multiple levels:
Consolidate individual sales reps' forecasts into team-level estimates.
Scale forecasts across regional teams and company-wide sales projections.
Gather management team insights to refine projections based on market share, industry shifts, and internal strategic goals.
5. Adjust for External Factors
While bottom-up forecasting is primarily based on internal sales data, incorporating external elements improves forecast accuracy:
Consider industry trends, competitive landscape, and market research.
Factor in economic conditions that may impact future sales performance.
Align projections with internal growth expectations and alternative forecasting methods for comparison.
6. Validate and Iterate
The final step in the bottom-up forecasting method involves continuous improvement:
Compare forecasted revenue with actual sales performance.
Identify deviations and refine assumptions based on detailed data analysis.
Adjust the forecasting process as new sales data and pipeline insights become available.
By following this structured approach, sales leaders can develop a bottom-up forecasting model that provides accurate forecasts, enhances sales team performance, and supports more effective revenue generation strategies.
Elevate your forecasting precision by diving into our step-by-step guide to improve sales forecasting accuracy, packed with data-driven strategies and actionable best practices.
Bottom-Up Forecasting Examples
Example 1: Small SaaS Company
A SaaS company with five sales reps selling a single product follows this bottom-up forecasting method to estimate revenue:
Each sales rep makes 50 outreach calls per week.
Their call-to-demo conversion rate is 20% (1 in 5 calls results in a demo).
Their demo-to-close conversion rate is 20% (1 in 5 demos results in a sale).
The average deal size is $5,000.
Revenue Projection Calculation:
Each rep books 10 demos per week (50 outreach calls × 20% demo booking rate).
Each rep closes 2 deals per week (10 demos × 20% close rate).
The weekly revenue per rep is $10,000 (2 deals × $5,000 deal size).
With 5 sales reps, total expected weekly revenue is $50,000 (5 reps × $10,000 per rep).
For a quarter (13 weeks), the company forecasts $650,000 in revenue (50,000 × 13).
By aggregating individual rep forecasts, the company builds an accurate forecast based on actual sales productivity, rather than relying on market-wide assumptions.
Example 2: Large B2B Enterprise (Pipeline-Based Forecasting)
A multinational B2B enterprise selling multiple products across various regions applies a bottom-up forecasting approach based on pipeline data. Instead of relying on cold outreach activity, the forecast is built using deal probabilities and sales reps’ commitments.
Step 1: Forecast at the Sales Rep Level
Each sales rep commits to closing a specific number of deals based on their pipeline data and deal stage probabilities.
Sales rep pipeline at the start of the quarter:
5 deals in the Proposal stage (60% probability of closing).
8 deals in Negotiation (80% probability of closing).
12 deals in Early-stage pipeline (30% probability of closing).
Expected closed deals for the quarter:
Proposal stage: 5 × 60% = 3 closed deals.
Negotiation stage: 8 × 80% = 6 closed deals.
Early-stage pipeline: 12 × 30% = 4 closed deals.
Total expected closed deals: 13 per rep.
Average deal size per rep: $50,000.
Quarterly revenue projection per rep: $650,000 (13 deals × $50,000).
Step 2: Aggregate Across Teams & Products
The company operates in five regions, each with multiple teams selling three product lines.
Team A (10 reps):
Total committed closed deals: 130 (10 reps × 13 deals per rep).
Expected revenue for the team: $6.5M (130 deals × $50,000).
Team B, C, D, and E follow similar calculations, adjusting for deal probabilities, sales cycle variations, and regional factors.
Each product category (e.g., Enterprise Software, Data Security, AI Tools) has its own pipeline, allowing for precise bottom-up sales estimates per product line.
Final Aggregation for Company-Wide Forecast
By summing up forecasts across teams, regions, and product lines, the company produces a highly accurate forecast for the upcoming forecast period. This bottom-up forecasting method ensures that sales leaders base their revenue projections on actual sales data rather than external market research or broad assumptions.
What is Needed to Implement Bottom-Up Forecasting
Implementing bottom-up forecasting requires a structured approach, combining detailed data analysis, standardized processes, and collaboration across sales teams. Unlike top-down forecasting, which relies on market research and high-level estimates, bottom-up forecasting focuses on real sales data to generate an accurate forecast. To ensure a smooth forecasting process, companies need the following key components:
A Reliable CRM System – A robust CRM system is essential for tracking deals in the pipeline, monitoring sales reps' performance, and analyzing historical data. It should provide visibility into pipeline stages, win rates, and total sales, ensuring real-time access to current sales data.
Defined Sales Metrics – Companies must establish clear performance indicators, including conversion rates, quota attainment, average deal size, and sales cycle length. These metrics help create precise revenue projections and improve forecast accuracy.
A Standardized Process – A well-defined bottom-up forecasting method ensures consistency across sales teams. Standardizing how bottom-up models are built—including how sales reps' commitments and deal probabilities are factored in—helps create a reliable and repeatable forecasting process.
Collaboration Between Teams – Sales, marketing, and finance teams must align to ensure accurate revenue generation forecasts. Sales leaders provide bottom-up sales insights, marketing teams contribute market research, and finance teams validate revenue projections against company goals.
Forecasting Software – Advanced forecasting software, such as Forecastio, enhances forecast accuracy by automating bottom-up forecasting formulas, performing time series analysis, and integrating historical data with current sales data. These tools help sales leaders refine forecasts and make data-driven decisions.
By leveraging these elements, companies can develop a bottom-up forecasting approach that improves future sales performance, maximizes sales productivity, and drives better revenue projections.
Pros and Cons of Bottom-Up Forecasting
Pros:
More accurate for companies with detailed historical sales data - When bottom-up forecasting is built on high-quality historical data and current sales data, it delivers accurate forecasts that reflect actual sales productivity rather than broad assumptions.
Allows for granular adjustments – Since bottom-up models are based on individual sales reps' performance and deal-level data, forecasts can be adjusted dynamically to account for changes in pipeline trends, win rates, or quota attainment.
Provides clear insights into individual and team performance – By tracking sales reps' commitments, deal probabilities, and revenue projections, sales leaders gain a deeper understanding of team performance and future sales performance.
Cons:
Requires high-quality sales data – Bottom-up forecasting focuses on detailed data analysis, meaning unreliable or incomplete sales data can reduce forecast accuracy and impact revenue generation.
Can be time-consuming – Collecting and analyzing bottom-up sales data requires significant effort, especially for companies with large sales teams operating in multiple sales channels.
May not account for sudden market shifts as well as top-down forecasting – Since bottom-up forecasting is based primarily on internal sales data, it may lack the broad market research perspective of top-down forecasting, making it less responsive to unexpected market share changes or economic conditions.
Other Sales Forecasting Methods
In addition to bottom-up forecasting and top-down forecasting, companies can leverage alternative forecasting methods to enhance forecast accuracy and adapt to different business needs. Some of the most effective approaches include:
Pipeline-Based Forecasting – Uses current sales data from the deal pipeline, factoring in deal probabilities at each stage to create a data-driven revenue projection. This method ensures forecasts are aligned with existing market conditions and sales cycle dynamics.
Time Series Forecasting – Applies historical data trends and regression analysis to predict future sales performance. This method relies on statistical models, such as moving averages or exponential smoothing, making it useful for businesses with stable sales cycles and consistent historical data patterns.
AI-Based Forecasting – Utilizes machine learning algorithms to analyze complex sales data, identifying hidden patterns in sales productivity, market share, and revenue generation. AI models continuously improve by learning from past performance, enabling sales leaders to make highly accurate forecasts.
Scenario Planning – Creates multiple forecasting models based on varying assumptions about market conditions, sales process changes, or external economic factors. This method allows sales teams to prepare for different business scenarios and adjust their forecasting process accordingly.
By combining these alternative forecasting methods with bottom-up models, companies can enhance their sales forecasting strategy, improve forecast accuracy, and optimize revenue projections.
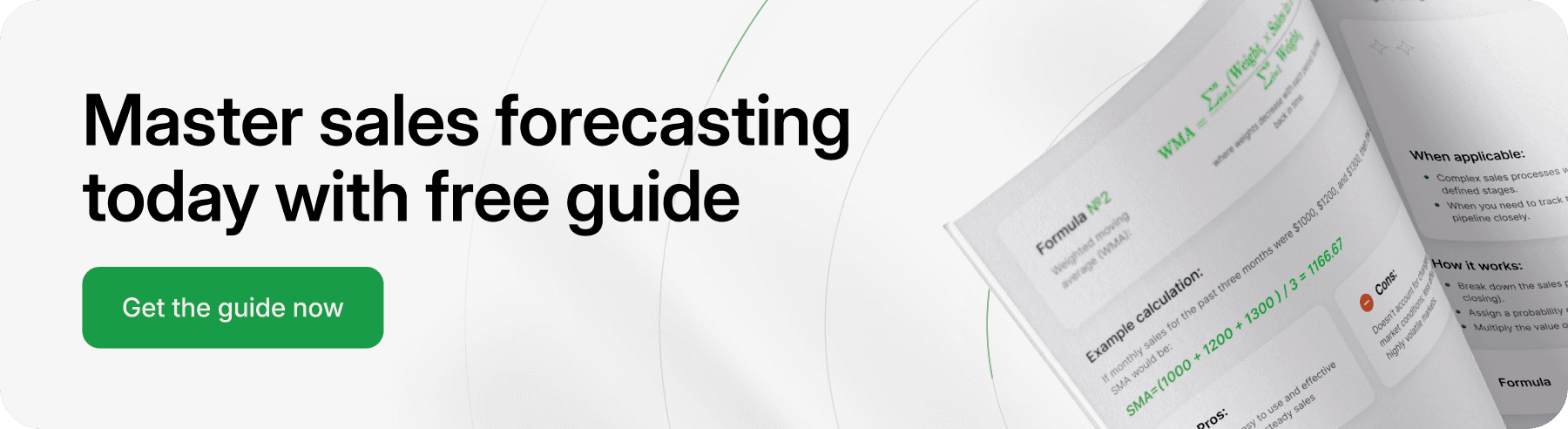
What Is Crucial to Improve Sales Forecasting Accuracy?
Achieving high forecast accuracy requires a combination of structured processes, reliable data, and collaboration across teams. To enhance sales forecasting, companies should focus on the following key areas:
Consistent Data Tracking – Ensure that all sales reps accurately log their activities, including deal updates, conversion rates, and sales cycle progress. Reliable current sales data is essential for improving bottom-up forecasting and making precise revenue projections.
Regular Forecast Reviews – Compare forecasted revenue with actual sales performance to identify gaps, refine assumptions, and improve the forecasting process. Incorporating historical data trends helps enhance forecast accuracy over time.
Cross-Team Collaboration – Align sales, marketing, finance, and operations to create a unified approach to revenue generation. Sales leaders must work closely with other departments to integrate market research, sales productivity insights, and total sales expectations into forecasting models.
Leveraging AI & Automation – Use forecasting software, such as AI-powered sales forecasting tools, to improve predictive accuracy. Machine learning models can analyze bottom-up sales data, recognize patterns, and enhance bottom-up models by accounting for external factors.
Continuous Training – Educate sales teams on best practices for pipeline management, quota attainment, and forecasting methods. Well-trained sales reps contribute to more reliable bottom-up forecasting formulas and ensure that forecasts are based on detailed data analysis rather than guesswork.
By implementing these best practices, companies can strengthen their sales forecasting process, enhance future sales performance, and drive more accurate forecasts for strategic decision-making.
Conclusion
Bottom-up forecasting is one of the most reliable and accurate forecasting methods for predicting sales performance and revenue generation in B2B companies. By leveraging granular data from individual sales reps, deal pipelines, and historical sales trends, businesses can develop highly precise revenue projections, optimize resource allocation, and make more informed, data-driven decisions.
However, for bottom-up forecasting to be truly effective, it requires a solid data foundation, consistent tracking of sales activities, and the use of advanced forecasting tools to automate calculations and enhance forecast accuracy. Without these elements, the reliability of bottom-up sales forecasts can be compromised.
For sales leaders aiming to refine their forecasting process, investing in AI-powered forecasting software, fostering cross-team collaboration, and continuously improving bottom-up models will be crucial. A disciplined approach to data-driven forecasting ensures that businesses stay agile, improve future sales performance, and drive sustained revenue growth in a competitive market.
Introduction
Accurate sales forecasting is one of the most critical aspects of B2B sales management, directly influencing future sales performance, revenue generation, and overall business growth. It enables sales leaders to set achievable revenue projections, allocate resources efficiently, and make data-driven strategic decisions. However, many organizations struggle with forecasting accuracy due to the limitations of traditional forecasting methods.
One commonly used approach is top-down forecasting, which relies heavily on high-level market trends, market research, and broad industry assumptions to estimate total sales. While this method provides a macro-level view, it often lacks precision because it doesn’t consider the detailed data analysis of current sales data, sales channels, or the actual performance of individual sales reps. This is where bottom-up forecasting provides a more reliable alternative.
Unlike the top-down approach, bottom-up forecasting focuses on analyzing historical data, individual sales reps' performance, and specific sales cycles to build a comprehensive and accurate forecast. This bottom-up approach begins at the granular level—using actual sales data from deals, pipelines, and sales teams—before scaling up to company-wide projections. The result is a forecasting process that reflects real, measurable activity and provides a more precise estimation of potential revenue.
In this guide, we will explore bottom-up forecasting in detail, explaining how it differs from top-down forecasting, its methodology, and how to implement it effectively. We will cover essential elements such as the bottom-up forecasting formula, real-world bottom-up forecasting examples, and the advantages and limitations of this bottom-up forecasting method.
Additionally, we will discuss alternative forecasting methods and best practices for improving forecast accuracy in B2B sales teams. Whether you are a sales leader, part of a management team, or a sales rep looking to refine your sales process, this guide will help you master bottom-up sales forecasting for more reliable and data-driven revenue projections.
What is Bottom-Up Forecasting?
Bottom-up forecasting is a sales forecasting method that generates revenue projections by analyzing detailed data at the ground level. Unlike top-down forecasting, which relies on broad market research, high-level assumptions, and macroeconomic indicators, bottom-up forecasting focuses on real, measurable activity within a company’s sales teams. This bottom-up approach starts with individual sales reps, specific deals, or even sales channels, aggregating these insights to create a more accurate forecast of future sales performance and potential revenue.
By leveraging current sales data, ongoing deals in the pipeline, and past sales cycles, companies can develop a highly tailored and precise forecasting process that reflects actual sales productivity. Since this method is rooted in real-time deal activity and historical data, it provides a dynamic, adaptable forecast that adjusts to changing conditions, making it particularly effective for improving forecast accuracy and revenue generation.
Key Characteristics of Bottom-Up Forecasting
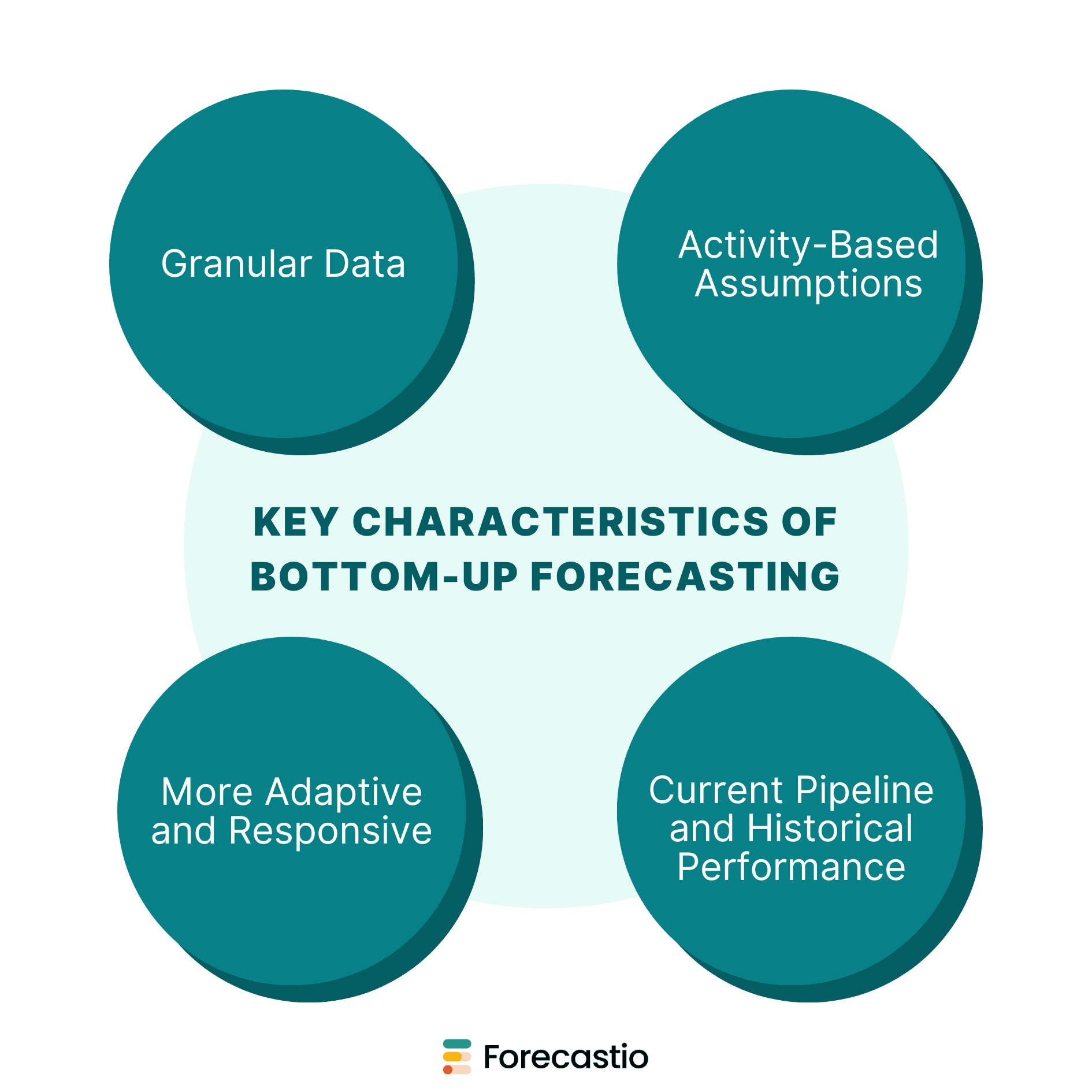
Granular Data: Unlike the top-down approach, which estimates total sales using broad industry figures, bottom-up forecasting relies on detailed data analysis from sales reps' performance, deal pipelines, and sales channels.
Activity-Based Assumptions: A bottom-up forecasting method considers specific sales process activities, such as outbound calls, booked demos, conversion rates, average deal size, and sales velocity, to generate more realistic revenue projections.
Current Pipeline and Historical Performance: A key element of bottom-up sales forecasting is its reliance on both historical data and current sales data from existing deals in the pipeline. By analyzing ongoing sales opportunities, it provides a real-time view of potential revenue, rather than relying solely on past performance.
More Adaptive and Responsive: Since bottom-up models are built on existing market data and current sales data, rather than external assumptions, they are highly adaptable. Companies can quickly adjust their forecasts when market conditions shift, ensuring a more dynamic and responsive forecasting process.
This bottom-up forecasting example demonstrates why many sales leaders prefer this method over top-down forecasting when looking to enhance forecast accuracy, optimize sales productivity, and make better-informed decisions based on real-time sales data.
If you want to get more detailed information about the most effective sales forecasting methods, read this article or download the ebook here.
Bottom-Up vs. Top-Down Forecasting
To understand the advantages of bottom-up forecasting, it’s essential to compare it with top-down forecasting and see how each forecasting method impacts forecast accuracy, adaptability, and data reliability.
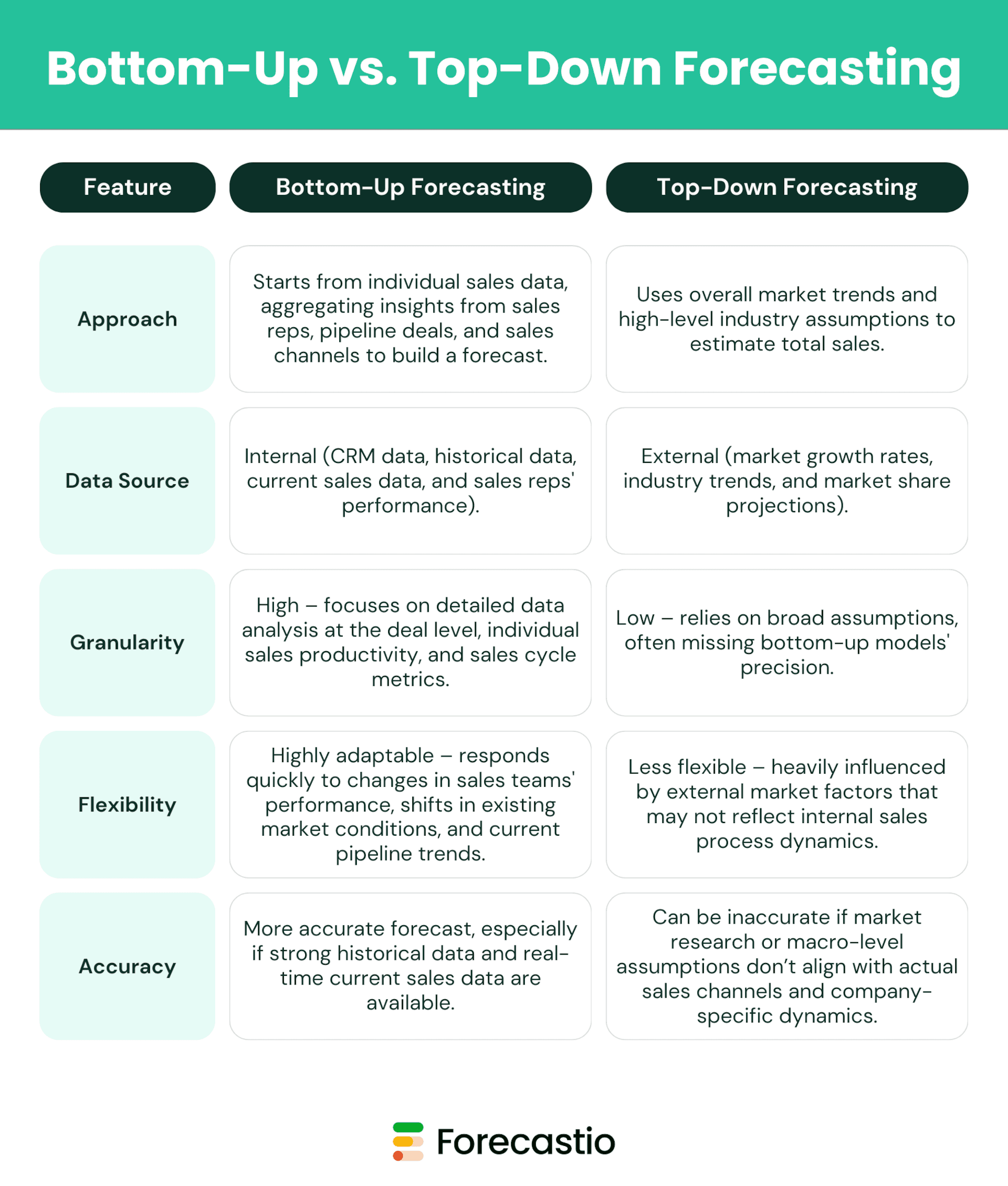
Why Bottom-Up Forecasting Is More Reliable for B2B Sales
For B2B companies, bottom-up forecasting is generally the preferred forecasting process, particularly in dynamic industries where external market share trends may not directly influence future sales performance. Since bottom-up forecasting focuses on real sales data from active pipeline deals, past sales cycles, and sales reps' productivity, it provides a more accurate forecast than the top-down approach, which can often overlook critical internal factors.
By leveraging bottom-up forecasting formulas and real-time bottom-up sales data, sales leaders can create revenue projections that reflect actual sales performance, leading to more precise planning, better sales productivity, and improved revenue generation.
How Bottom-Up Forecasting Works
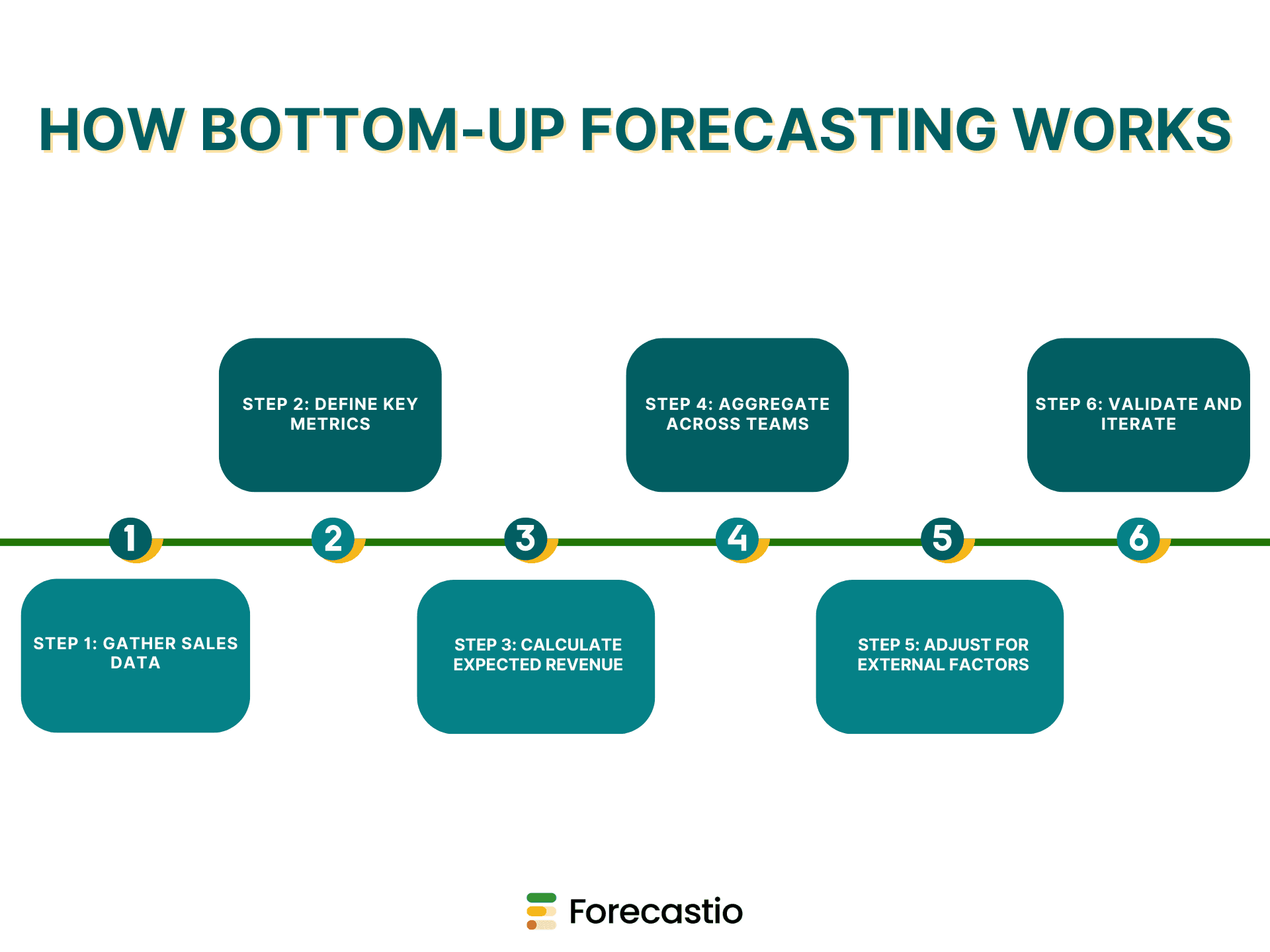
The bottom-up forecasting process follows a structured, data-driven methodology that aggregates insights from individual sales reps, current sales data, and historical data to generate a more accurate forecast. Unlike top-down forecasting, which starts with market research and broad assumptions, bottom-up forecasting focuses on granular, deal-level details to provide reliable revenue projections.
Below is a step-by-step approach to implementing bottom-up sales forecasting effectively:
1. Gather Sales Data
The foundation of bottom-up forecasting is detailed data analysis from sales reps, active deals in the pipeline, and historical data trends. Key data sources include:
Individual rep performance metrics (e.g., number of deals closed, conversion rates, outbound activity, demo-to-close ratio).
Pipeline analysis, including deal velocity, win rates by stage, and overall sales cycle length.
Revenue per account, factoring in past total sales and existing market penetration.
Sales channels and their contribution to revenue generation.
2. Define Key Metrics
To create an accurate forecast, specific sales performance indicators need to be established:
Number of sales reps actively closing deals.
Average quota attainment per rep.
Average deal size based on historical data and current sales data.
Sales cycle length, determining how long it takes for deals to close.
Win rate per stage, helping refine the bottom-up forecasting formula.
3. Calculate Expected Revenue
Once key metrics are identified, the next step is estimating potential revenue:
Determine the number of deals expected to close using historical performance and current pipeline data.
Assign deal probabilities based on pipeline stage (e.g., a deal in the proposal stage might have a 50% chance of closing, while a deal in negotiation might have an 80% probability).
Factor in sales reps’ commitments, as reps often provide their own estimates on which deals they believe will close.
Multiply the adjusted expected deal count by the average deal size to generate preliminary revenue projections.
Adjust calculations based on seasonality, pipeline trends, and variations in sales productivity.
4. Aggregate Across Teams
To transition from individual forecasts to an organizational sales forecast, data is rolled up across multiple levels:
Consolidate individual sales reps' forecasts into team-level estimates.
Scale forecasts across regional teams and company-wide sales projections.
Gather management team insights to refine projections based on market share, industry shifts, and internal strategic goals.
5. Adjust for External Factors
While bottom-up forecasting is primarily based on internal sales data, incorporating external elements improves forecast accuracy:
Consider industry trends, competitive landscape, and market research.
Factor in economic conditions that may impact future sales performance.
Align projections with internal growth expectations and alternative forecasting methods for comparison.
6. Validate and Iterate
The final step in the bottom-up forecasting method involves continuous improvement:
Compare forecasted revenue with actual sales performance.
Identify deviations and refine assumptions based on detailed data analysis.
Adjust the forecasting process as new sales data and pipeline insights become available.
By following this structured approach, sales leaders can develop a bottom-up forecasting model that provides accurate forecasts, enhances sales team performance, and supports more effective revenue generation strategies.
Elevate your forecasting precision by diving into our step-by-step guide to improve sales forecasting accuracy, packed with data-driven strategies and actionable best practices.
Bottom-Up Forecasting Examples
Example 1: Small SaaS Company
A SaaS company with five sales reps selling a single product follows this bottom-up forecasting method to estimate revenue:
Each sales rep makes 50 outreach calls per week.
Their call-to-demo conversion rate is 20% (1 in 5 calls results in a demo).
Their demo-to-close conversion rate is 20% (1 in 5 demos results in a sale).
The average deal size is $5,000.
Revenue Projection Calculation:
Each rep books 10 demos per week (50 outreach calls × 20% demo booking rate).
Each rep closes 2 deals per week (10 demos × 20% close rate).
The weekly revenue per rep is $10,000 (2 deals × $5,000 deal size).
With 5 sales reps, total expected weekly revenue is $50,000 (5 reps × $10,000 per rep).
For a quarter (13 weeks), the company forecasts $650,000 in revenue (50,000 × 13).
By aggregating individual rep forecasts, the company builds an accurate forecast based on actual sales productivity, rather than relying on market-wide assumptions.
Example 2: Large B2B Enterprise (Pipeline-Based Forecasting)
A multinational B2B enterprise selling multiple products across various regions applies a bottom-up forecasting approach based on pipeline data. Instead of relying on cold outreach activity, the forecast is built using deal probabilities and sales reps’ commitments.
Step 1: Forecast at the Sales Rep Level
Each sales rep commits to closing a specific number of deals based on their pipeline data and deal stage probabilities.
Sales rep pipeline at the start of the quarter:
5 deals in the Proposal stage (60% probability of closing).
8 deals in Negotiation (80% probability of closing).
12 deals in Early-stage pipeline (30% probability of closing).
Expected closed deals for the quarter:
Proposal stage: 5 × 60% = 3 closed deals.
Negotiation stage: 8 × 80% = 6 closed deals.
Early-stage pipeline: 12 × 30% = 4 closed deals.
Total expected closed deals: 13 per rep.
Average deal size per rep: $50,000.
Quarterly revenue projection per rep: $650,000 (13 deals × $50,000).
Step 2: Aggregate Across Teams & Products
The company operates in five regions, each with multiple teams selling three product lines.
Team A (10 reps):
Total committed closed deals: 130 (10 reps × 13 deals per rep).
Expected revenue for the team: $6.5M (130 deals × $50,000).
Team B, C, D, and E follow similar calculations, adjusting for deal probabilities, sales cycle variations, and regional factors.
Each product category (e.g., Enterprise Software, Data Security, AI Tools) has its own pipeline, allowing for precise bottom-up sales estimates per product line.
Final Aggregation for Company-Wide Forecast
By summing up forecasts across teams, regions, and product lines, the company produces a highly accurate forecast for the upcoming forecast period. This bottom-up forecasting method ensures that sales leaders base their revenue projections on actual sales data rather than external market research or broad assumptions.
What is Needed to Implement Bottom-Up Forecasting
Implementing bottom-up forecasting requires a structured approach, combining detailed data analysis, standardized processes, and collaboration across sales teams. Unlike top-down forecasting, which relies on market research and high-level estimates, bottom-up forecasting focuses on real sales data to generate an accurate forecast. To ensure a smooth forecasting process, companies need the following key components:
A Reliable CRM System – A robust CRM system is essential for tracking deals in the pipeline, monitoring sales reps' performance, and analyzing historical data. It should provide visibility into pipeline stages, win rates, and total sales, ensuring real-time access to current sales data.
Defined Sales Metrics – Companies must establish clear performance indicators, including conversion rates, quota attainment, average deal size, and sales cycle length. These metrics help create precise revenue projections and improve forecast accuracy.
A Standardized Process – A well-defined bottom-up forecasting method ensures consistency across sales teams. Standardizing how bottom-up models are built—including how sales reps' commitments and deal probabilities are factored in—helps create a reliable and repeatable forecasting process.
Collaboration Between Teams – Sales, marketing, and finance teams must align to ensure accurate revenue generation forecasts. Sales leaders provide bottom-up sales insights, marketing teams contribute market research, and finance teams validate revenue projections against company goals.
Forecasting Software – Advanced forecasting software, such as Forecastio, enhances forecast accuracy by automating bottom-up forecasting formulas, performing time series analysis, and integrating historical data with current sales data. These tools help sales leaders refine forecasts and make data-driven decisions.
By leveraging these elements, companies can develop a bottom-up forecasting approach that improves future sales performance, maximizes sales productivity, and drives better revenue projections.
Pros and Cons of Bottom-Up Forecasting
Pros:
More accurate for companies with detailed historical sales data - When bottom-up forecasting is built on high-quality historical data and current sales data, it delivers accurate forecasts that reflect actual sales productivity rather than broad assumptions.
Allows for granular adjustments – Since bottom-up models are based on individual sales reps' performance and deal-level data, forecasts can be adjusted dynamically to account for changes in pipeline trends, win rates, or quota attainment.
Provides clear insights into individual and team performance – By tracking sales reps' commitments, deal probabilities, and revenue projections, sales leaders gain a deeper understanding of team performance and future sales performance.
Cons:
Requires high-quality sales data – Bottom-up forecasting focuses on detailed data analysis, meaning unreliable or incomplete sales data can reduce forecast accuracy and impact revenue generation.
Can be time-consuming – Collecting and analyzing bottom-up sales data requires significant effort, especially for companies with large sales teams operating in multiple sales channels.
May not account for sudden market shifts as well as top-down forecasting – Since bottom-up forecasting is based primarily on internal sales data, it may lack the broad market research perspective of top-down forecasting, making it less responsive to unexpected market share changes or economic conditions.
Other Sales Forecasting Methods
In addition to bottom-up forecasting and top-down forecasting, companies can leverage alternative forecasting methods to enhance forecast accuracy and adapt to different business needs. Some of the most effective approaches include:
Pipeline-Based Forecasting – Uses current sales data from the deal pipeline, factoring in deal probabilities at each stage to create a data-driven revenue projection. This method ensures forecasts are aligned with existing market conditions and sales cycle dynamics.
Time Series Forecasting – Applies historical data trends and regression analysis to predict future sales performance. This method relies on statistical models, such as moving averages or exponential smoothing, making it useful for businesses with stable sales cycles and consistent historical data patterns.
AI-Based Forecasting – Utilizes machine learning algorithms to analyze complex sales data, identifying hidden patterns in sales productivity, market share, and revenue generation. AI models continuously improve by learning from past performance, enabling sales leaders to make highly accurate forecasts.
Scenario Planning – Creates multiple forecasting models based on varying assumptions about market conditions, sales process changes, or external economic factors. This method allows sales teams to prepare for different business scenarios and adjust their forecasting process accordingly.
By combining these alternative forecasting methods with bottom-up models, companies can enhance their sales forecasting strategy, improve forecast accuracy, and optimize revenue projections.
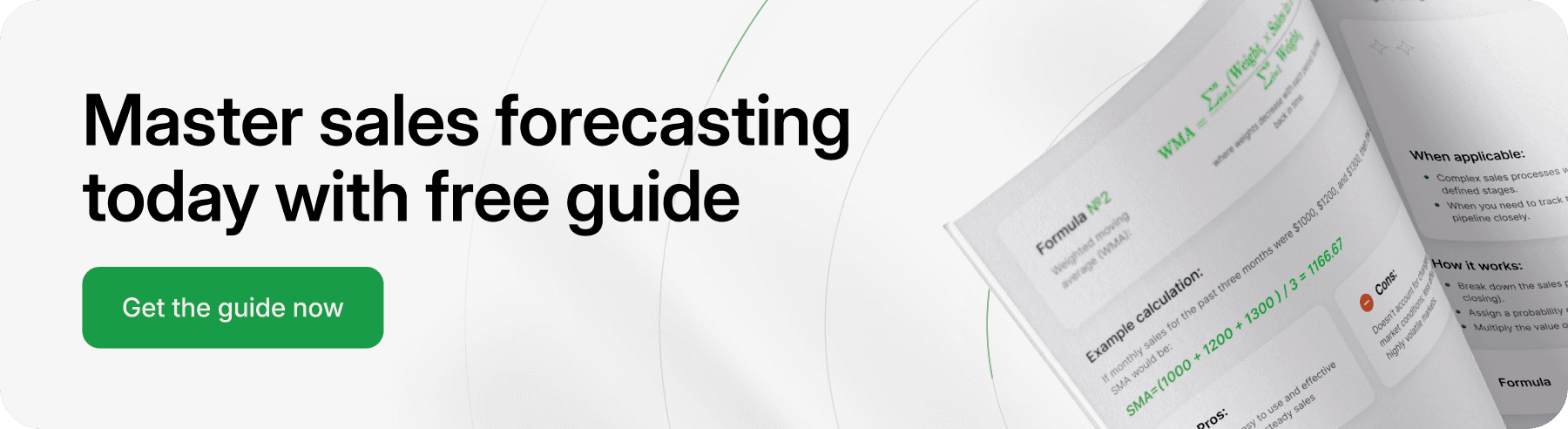
What Is Crucial to Improve Sales Forecasting Accuracy?
Achieving high forecast accuracy requires a combination of structured processes, reliable data, and collaboration across teams. To enhance sales forecasting, companies should focus on the following key areas:
Consistent Data Tracking – Ensure that all sales reps accurately log their activities, including deal updates, conversion rates, and sales cycle progress. Reliable current sales data is essential for improving bottom-up forecasting and making precise revenue projections.
Regular Forecast Reviews – Compare forecasted revenue with actual sales performance to identify gaps, refine assumptions, and improve the forecasting process. Incorporating historical data trends helps enhance forecast accuracy over time.
Cross-Team Collaboration – Align sales, marketing, finance, and operations to create a unified approach to revenue generation. Sales leaders must work closely with other departments to integrate market research, sales productivity insights, and total sales expectations into forecasting models.
Leveraging AI & Automation – Use forecasting software, such as AI-powered sales forecasting tools, to improve predictive accuracy. Machine learning models can analyze bottom-up sales data, recognize patterns, and enhance bottom-up models by accounting for external factors.
Continuous Training – Educate sales teams on best practices for pipeline management, quota attainment, and forecasting methods. Well-trained sales reps contribute to more reliable bottom-up forecasting formulas and ensure that forecasts are based on detailed data analysis rather than guesswork.
By implementing these best practices, companies can strengthen their sales forecasting process, enhance future sales performance, and drive more accurate forecasts for strategic decision-making.
Conclusion
Bottom-up forecasting is one of the most reliable and accurate forecasting methods for predicting sales performance and revenue generation in B2B companies. By leveraging granular data from individual sales reps, deal pipelines, and historical sales trends, businesses can develop highly precise revenue projections, optimize resource allocation, and make more informed, data-driven decisions.
However, for bottom-up forecasting to be truly effective, it requires a solid data foundation, consistent tracking of sales activities, and the use of advanced forecasting tools to automate calculations and enhance forecast accuracy. Without these elements, the reliability of bottom-up sales forecasts can be compromised.
For sales leaders aiming to refine their forecasting process, investing in AI-powered forecasting software, fostering cross-team collaboration, and continuously improving bottom-up models will be crucial. A disciplined approach to data-driven forecasting ensures that businesses stay agile, improve future sales performance, and drive sustained revenue growth in a competitive market.
Introduction
Accurate sales forecasting is one of the most critical aspects of B2B sales management, directly influencing future sales performance, revenue generation, and overall business growth. It enables sales leaders to set achievable revenue projections, allocate resources efficiently, and make data-driven strategic decisions. However, many organizations struggle with forecasting accuracy due to the limitations of traditional forecasting methods.
One commonly used approach is top-down forecasting, which relies heavily on high-level market trends, market research, and broad industry assumptions to estimate total sales. While this method provides a macro-level view, it often lacks precision because it doesn’t consider the detailed data analysis of current sales data, sales channels, or the actual performance of individual sales reps. This is where bottom-up forecasting provides a more reliable alternative.
Unlike the top-down approach, bottom-up forecasting focuses on analyzing historical data, individual sales reps' performance, and specific sales cycles to build a comprehensive and accurate forecast. This bottom-up approach begins at the granular level—using actual sales data from deals, pipelines, and sales teams—before scaling up to company-wide projections. The result is a forecasting process that reflects real, measurable activity and provides a more precise estimation of potential revenue.
In this guide, we will explore bottom-up forecasting in detail, explaining how it differs from top-down forecasting, its methodology, and how to implement it effectively. We will cover essential elements such as the bottom-up forecasting formula, real-world bottom-up forecasting examples, and the advantages and limitations of this bottom-up forecasting method.
Additionally, we will discuss alternative forecasting methods and best practices for improving forecast accuracy in B2B sales teams. Whether you are a sales leader, part of a management team, or a sales rep looking to refine your sales process, this guide will help you master bottom-up sales forecasting for more reliable and data-driven revenue projections.
What is Bottom-Up Forecasting?
Bottom-up forecasting is a sales forecasting method that generates revenue projections by analyzing detailed data at the ground level. Unlike top-down forecasting, which relies on broad market research, high-level assumptions, and macroeconomic indicators, bottom-up forecasting focuses on real, measurable activity within a company’s sales teams. This bottom-up approach starts with individual sales reps, specific deals, or even sales channels, aggregating these insights to create a more accurate forecast of future sales performance and potential revenue.
By leveraging current sales data, ongoing deals in the pipeline, and past sales cycles, companies can develop a highly tailored and precise forecasting process that reflects actual sales productivity. Since this method is rooted in real-time deal activity and historical data, it provides a dynamic, adaptable forecast that adjusts to changing conditions, making it particularly effective for improving forecast accuracy and revenue generation.
Key Characteristics of Bottom-Up Forecasting
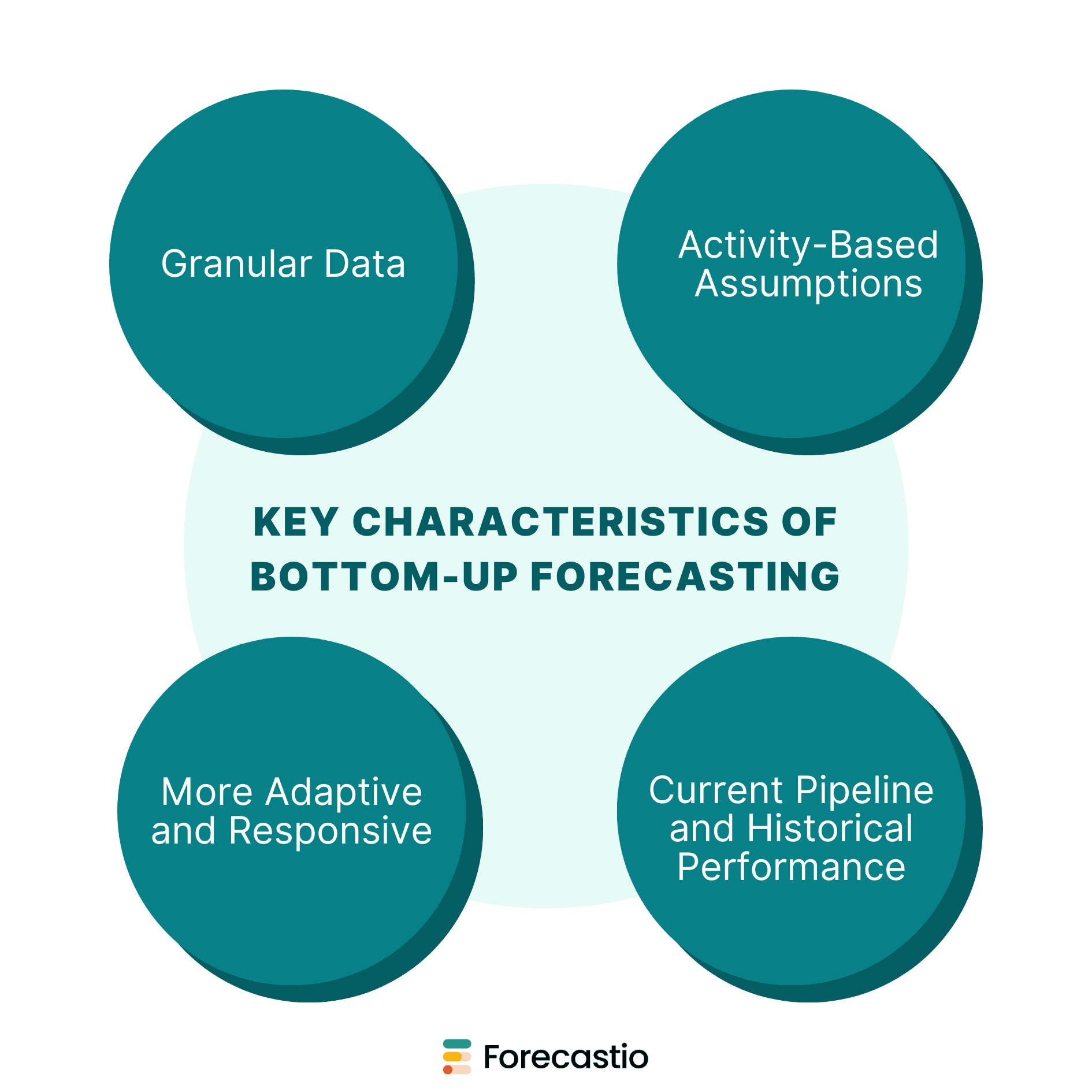
Granular Data: Unlike the top-down approach, which estimates total sales using broad industry figures, bottom-up forecasting relies on detailed data analysis from sales reps' performance, deal pipelines, and sales channels.
Activity-Based Assumptions: A bottom-up forecasting method considers specific sales process activities, such as outbound calls, booked demos, conversion rates, average deal size, and sales velocity, to generate more realistic revenue projections.
Current Pipeline and Historical Performance: A key element of bottom-up sales forecasting is its reliance on both historical data and current sales data from existing deals in the pipeline. By analyzing ongoing sales opportunities, it provides a real-time view of potential revenue, rather than relying solely on past performance.
More Adaptive and Responsive: Since bottom-up models are built on existing market data and current sales data, rather than external assumptions, they are highly adaptable. Companies can quickly adjust their forecasts when market conditions shift, ensuring a more dynamic and responsive forecasting process.
This bottom-up forecasting example demonstrates why many sales leaders prefer this method over top-down forecasting when looking to enhance forecast accuracy, optimize sales productivity, and make better-informed decisions based on real-time sales data.
If you want to get more detailed information about the most effective sales forecasting methods, read this article or download the ebook here.
Bottom-Up vs. Top-Down Forecasting
To understand the advantages of bottom-up forecasting, it’s essential to compare it with top-down forecasting and see how each forecasting method impacts forecast accuracy, adaptability, and data reliability.
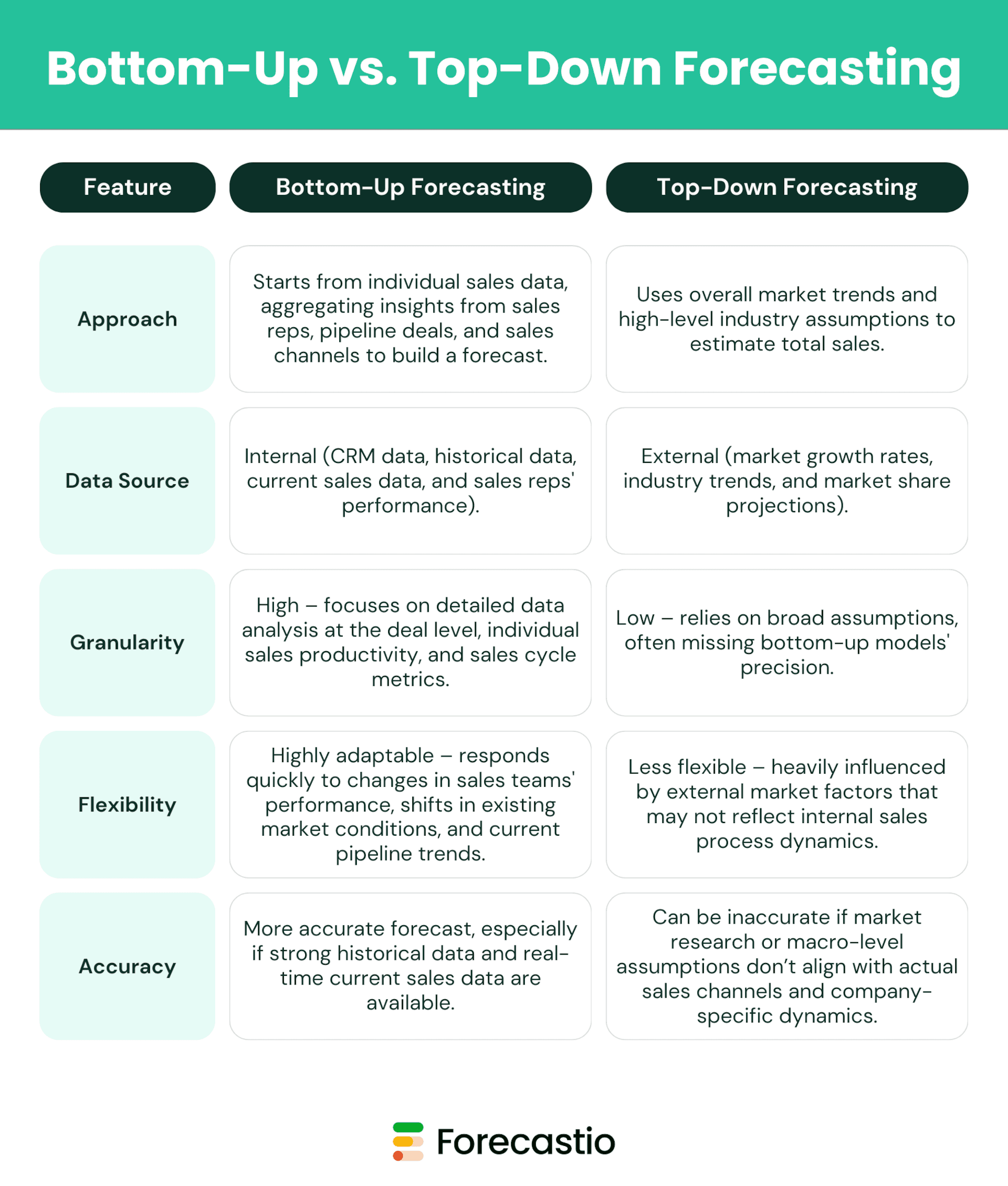
Why Bottom-Up Forecasting Is More Reliable for B2B Sales
For B2B companies, bottom-up forecasting is generally the preferred forecasting process, particularly in dynamic industries where external market share trends may not directly influence future sales performance. Since bottom-up forecasting focuses on real sales data from active pipeline deals, past sales cycles, and sales reps' productivity, it provides a more accurate forecast than the top-down approach, which can often overlook critical internal factors.
By leveraging bottom-up forecasting formulas and real-time bottom-up sales data, sales leaders can create revenue projections that reflect actual sales performance, leading to more precise planning, better sales productivity, and improved revenue generation.
How Bottom-Up Forecasting Works
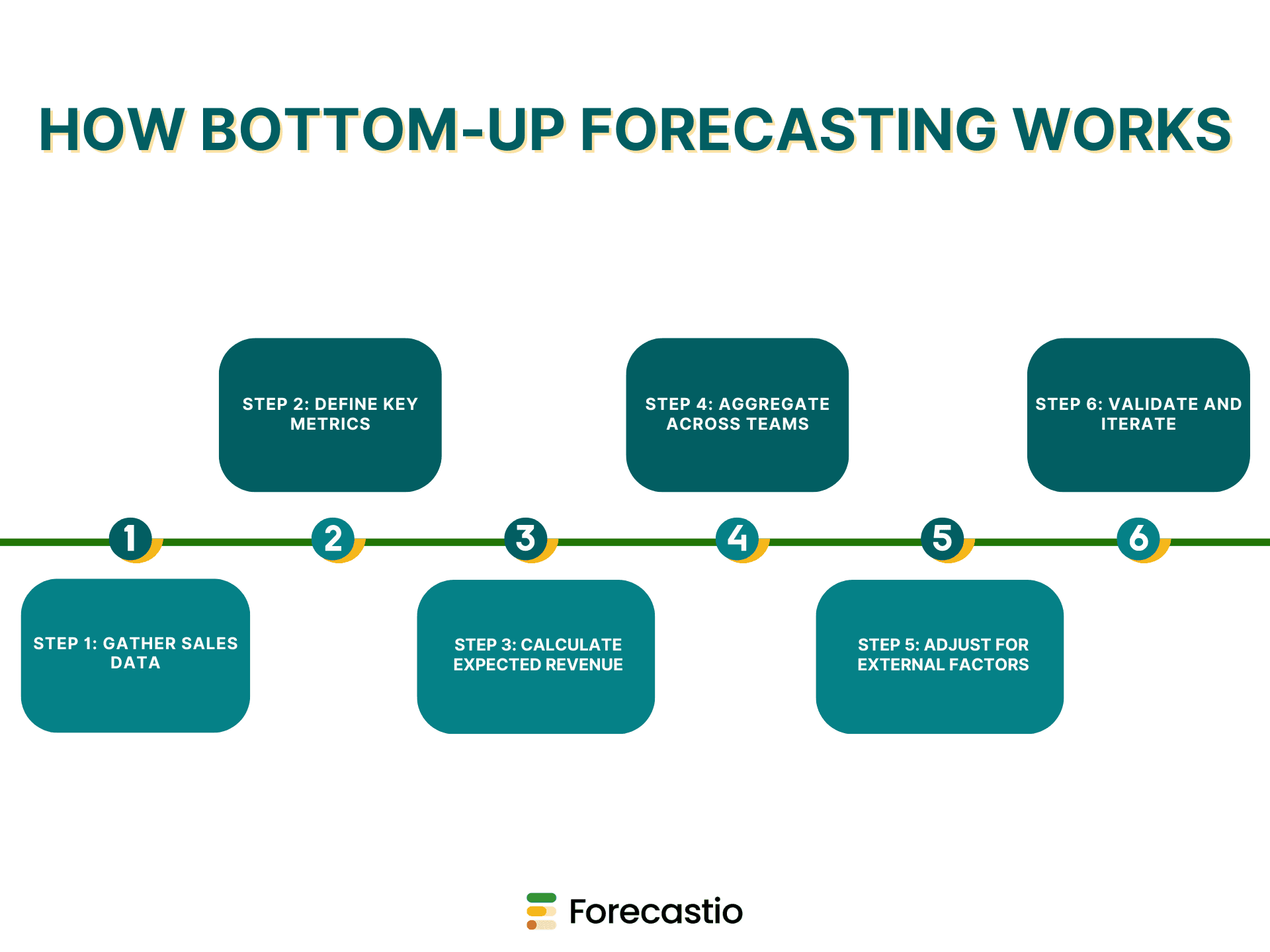
The bottom-up forecasting process follows a structured, data-driven methodology that aggregates insights from individual sales reps, current sales data, and historical data to generate a more accurate forecast. Unlike top-down forecasting, which starts with market research and broad assumptions, bottom-up forecasting focuses on granular, deal-level details to provide reliable revenue projections.
Below is a step-by-step approach to implementing bottom-up sales forecasting effectively:
1. Gather Sales Data
The foundation of bottom-up forecasting is detailed data analysis from sales reps, active deals in the pipeline, and historical data trends. Key data sources include:
Individual rep performance metrics (e.g., number of deals closed, conversion rates, outbound activity, demo-to-close ratio).
Pipeline analysis, including deal velocity, win rates by stage, and overall sales cycle length.
Revenue per account, factoring in past total sales and existing market penetration.
Sales channels and their contribution to revenue generation.
2. Define Key Metrics
To create an accurate forecast, specific sales performance indicators need to be established:
Number of sales reps actively closing deals.
Average quota attainment per rep.
Average deal size based on historical data and current sales data.
Sales cycle length, determining how long it takes for deals to close.
Win rate per stage, helping refine the bottom-up forecasting formula.
3. Calculate Expected Revenue
Once key metrics are identified, the next step is estimating potential revenue:
Determine the number of deals expected to close using historical performance and current pipeline data.
Assign deal probabilities based on pipeline stage (e.g., a deal in the proposal stage might have a 50% chance of closing, while a deal in negotiation might have an 80% probability).
Factor in sales reps’ commitments, as reps often provide their own estimates on which deals they believe will close.
Multiply the adjusted expected deal count by the average deal size to generate preliminary revenue projections.
Adjust calculations based on seasonality, pipeline trends, and variations in sales productivity.
4. Aggregate Across Teams
To transition from individual forecasts to an organizational sales forecast, data is rolled up across multiple levels:
Consolidate individual sales reps' forecasts into team-level estimates.
Scale forecasts across regional teams and company-wide sales projections.
Gather management team insights to refine projections based on market share, industry shifts, and internal strategic goals.
5. Adjust for External Factors
While bottom-up forecasting is primarily based on internal sales data, incorporating external elements improves forecast accuracy:
Consider industry trends, competitive landscape, and market research.
Factor in economic conditions that may impact future sales performance.
Align projections with internal growth expectations and alternative forecasting methods for comparison.
6. Validate and Iterate
The final step in the bottom-up forecasting method involves continuous improvement:
Compare forecasted revenue with actual sales performance.
Identify deviations and refine assumptions based on detailed data analysis.
Adjust the forecasting process as new sales data and pipeline insights become available.
By following this structured approach, sales leaders can develop a bottom-up forecasting model that provides accurate forecasts, enhances sales team performance, and supports more effective revenue generation strategies.
Elevate your forecasting precision by diving into our step-by-step guide to improve sales forecasting accuracy, packed with data-driven strategies and actionable best practices.
Bottom-Up Forecasting Examples
Example 1: Small SaaS Company
A SaaS company with five sales reps selling a single product follows this bottom-up forecasting method to estimate revenue:
Each sales rep makes 50 outreach calls per week.
Their call-to-demo conversion rate is 20% (1 in 5 calls results in a demo).
Their demo-to-close conversion rate is 20% (1 in 5 demos results in a sale).
The average deal size is $5,000.
Revenue Projection Calculation:
Each rep books 10 demos per week (50 outreach calls × 20% demo booking rate).
Each rep closes 2 deals per week (10 demos × 20% close rate).
The weekly revenue per rep is $10,000 (2 deals × $5,000 deal size).
With 5 sales reps, total expected weekly revenue is $50,000 (5 reps × $10,000 per rep).
For a quarter (13 weeks), the company forecasts $650,000 in revenue (50,000 × 13).
By aggregating individual rep forecasts, the company builds an accurate forecast based on actual sales productivity, rather than relying on market-wide assumptions.
Example 2: Large B2B Enterprise (Pipeline-Based Forecasting)
A multinational B2B enterprise selling multiple products across various regions applies a bottom-up forecasting approach based on pipeline data. Instead of relying on cold outreach activity, the forecast is built using deal probabilities and sales reps’ commitments.
Step 1: Forecast at the Sales Rep Level
Each sales rep commits to closing a specific number of deals based on their pipeline data and deal stage probabilities.
Sales rep pipeline at the start of the quarter:
5 deals in the Proposal stage (60% probability of closing).
8 deals in Negotiation (80% probability of closing).
12 deals in Early-stage pipeline (30% probability of closing).
Expected closed deals for the quarter:
Proposal stage: 5 × 60% = 3 closed deals.
Negotiation stage: 8 × 80% = 6 closed deals.
Early-stage pipeline: 12 × 30% = 4 closed deals.
Total expected closed deals: 13 per rep.
Average deal size per rep: $50,000.
Quarterly revenue projection per rep: $650,000 (13 deals × $50,000).
Step 2: Aggregate Across Teams & Products
The company operates in five regions, each with multiple teams selling three product lines.
Team A (10 reps):
Total committed closed deals: 130 (10 reps × 13 deals per rep).
Expected revenue for the team: $6.5M (130 deals × $50,000).
Team B, C, D, and E follow similar calculations, adjusting for deal probabilities, sales cycle variations, and regional factors.
Each product category (e.g., Enterprise Software, Data Security, AI Tools) has its own pipeline, allowing for precise bottom-up sales estimates per product line.
Final Aggregation for Company-Wide Forecast
By summing up forecasts across teams, regions, and product lines, the company produces a highly accurate forecast for the upcoming forecast period. This bottom-up forecasting method ensures that sales leaders base their revenue projections on actual sales data rather than external market research or broad assumptions.
What is Needed to Implement Bottom-Up Forecasting
Implementing bottom-up forecasting requires a structured approach, combining detailed data analysis, standardized processes, and collaboration across sales teams. Unlike top-down forecasting, which relies on market research and high-level estimates, bottom-up forecasting focuses on real sales data to generate an accurate forecast. To ensure a smooth forecasting process, companies need the following key components:
A Reliable CRM System – A robust CRM system is essential for tracking deals in the pipeline, monitoring sales reps' performance, and analyzing historical data. It should provide visibility into pipeline stages, win rates, and total sales, ensuring real-time access to current sales data.
Defined Sales Metrics – Companies must establish clear performance indicators, including conversion rates, quota attainment, average deal size, and sales cycle length. These metrics help create precise revenue projections and improve forecast accuracy.
A Standardized Process – A well-defined bottom-up forecasting method ensures consistency across sales teams. Standardizing how bottom-up models are built—including how sales reps' commitments and deal probabilities are factored in—helps create a reliable and repeatable forecasting process.
Collaboration Between Teams – Sales, marketing, and finance teams must align to ensure accurate revenue generation forecasts. Sales leaders provide bottom-up sales insights, marketing teams contribute market research, and finance teams validate revenue projections against company goals.
Forecasting Software – Advanced forecasting software, such as Forecastio, enhances forecast accuracy by automating bottom-up forecasting formulas, performing time series analysis, and integrating historical data with current sales data. These tools help sales leaders refine forecasts and make data-driven decisions.
By leveraging these elements, companies can develop a bottom-up forecasting approach that improves future sales performance, maximizes sales productivity, and drives better revenue projections.
Pros and Cons of Bottom-Up Forecasting
Pros:
More accurate for companies with detailed historical sales data - When bottom-up forecasting is built on high-quality historical data and current sales data, it delivers accurate forecasts that reflect actual sales productivity rather than broad assumptions.
Allows for granular adjustments – Since bottom-up models are based on individual sales reps' performance and deal-level data, forecasts can be adjusted dynamically to account for changes in pipeline trends, win rates, or quota attainment.
Provides clear insights into individual and team performance – By tracking sales reps' commitments, deal probabilities, and revenue projections, sales leaders gain a deeper understanding of team performance and future sales performance.
Cons:
Requires high-quality sales data – Bottom-up forecasting focuses on detailed data analysis, meaning unreliable or incomplete sales data can reduce forecast accuracy and impact revenue generation.
Can be time-consuming – Collecting and analyzing bottom-up sales data requires significant effort, especially for companies with large sales teams operating in multiple sales channels.
May not account for sudden market shifts as well as top-down forecasting – Since bottom-up forecasting is based primarily on internal sales data, it may lack the broad market research perspective of top-down forecasting, making it less responsive to unexpected market share changes or economic conditions.
Other Sales Forecasting Methods
In addition to bottom-up forecasting and top-down forecasting, companies can leverage alternative forecasting methods to enhance forecast accuracy and adapt to different business needs. Some of the most effective approaches include:
Pipeline-Based Forecasting – Uses current sales data from the deal pipeline, factoring in deal probabilities at each stage to create a data-driven revenue projection. This method ensures forecasts are aligned with existing market conditions and sales cycle dynamics.
Time Series Forecasting – Applies historical data trends and regression analysis to predict future sales performance. This method relies on statistical models, such as moving averages or exponential smoothing, making it useful for businesses with stable sales cycles and consistent historical data patterns.
AI-Based Forecasting – Utilizes machine learning algorithms to analyze complex sales data, identifying hidden patterns in sales productivity, market share, and revenue generation. AI models continuously improve by learning from past performance, enabling sales leaders to make highly accurate forecasts.
Scenario Planning – Creates multiple forecasting models based on varying assumptions about market conditions, sales process changes, or external economic factors. This method allows sales teams to prepare for different business scenarios and adjust their forecasting process accordingly.
By combining these alternative forecasting methods with bottom-up models, companies can enhance their sales forecasting strategy, improve forecast accuracy, and optimize revenue projections.
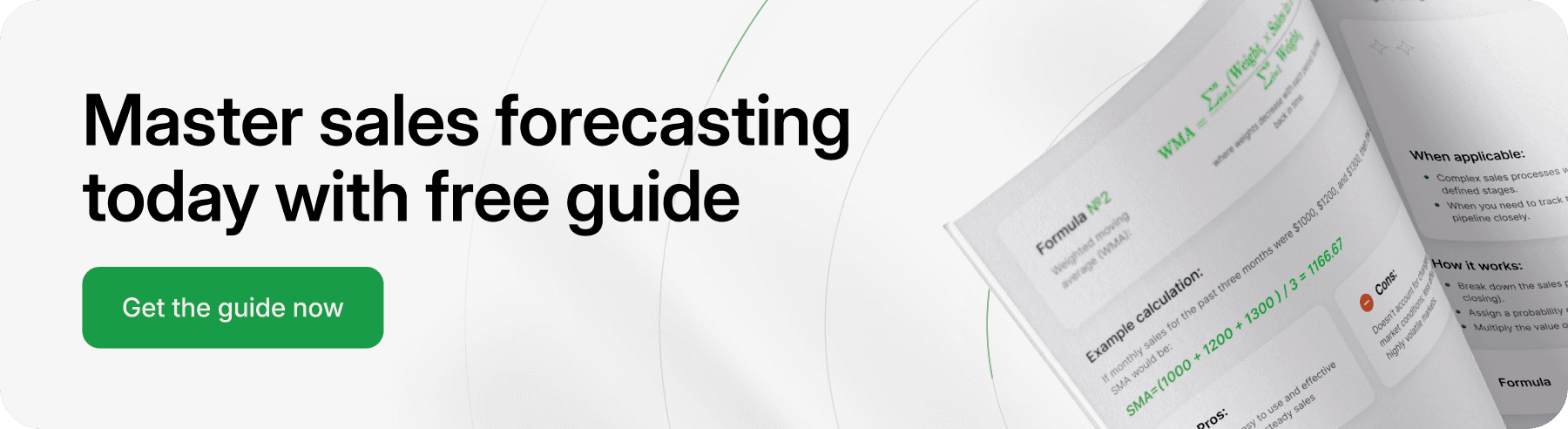
What Is Crucial to Improve Sales Forecasting Accuracy?
Achieving high forecast accuracy requires a combination of structured processes, reliable data, and collaboration across teams. To enhance sales forecasting, companies should focus on the following key areas:
Consistent Data Tracking – Ensure that all sales reps accurately log their activities, including deal updates, conversion rates, and sales cycle progress. Reliable current sales data is essential for improving bottom-up forecasting and making precise revenue projections.
Regular Forecast Reviews – Compare forecasted revenue with actual sales performance to identify gaps, refine assumptions, and improve the forecasting process. Incorporating historical data trends helps enhance forecast accuracy over time.
Cross-Team Collaboration – Align sales, marketing, finance, and operations to create a unified approach to revenue generation. Sales leaders must work closely with other departments to integrate market research, sales productivity insights, and total sales expectations into forecasting models.
Leveraging AI & Automation – Use forecasting software, such as AI-powered sales forecasting tools, to improve predictive accuracy. Machine learning models can analyze bottom-up sales data, recognize patterns, and enhance bottom-up models by accounting for external factors.
Continuous Training – Educate sales teams on best practices for pipeline management, quota attainment, and forecasting methods. Well-trained sales reps contribute to more reliable bottom-up forecasting formulas and ensure that forecasts are based on detailed data analysis rather than guesswork.
By implementing these best practices, companies can strengthen their sales forecasting process, enhance future sales performance, and drive more accurate forecasts for strategic decision-making.
Conclusion
Bottom-up forecasting is one of the most reliable and accurate forecasting methods for predicting sales performance and revenue generation in B2B companies. By leveraging granular data from individual sales reps, deal pipelines, and historical sales trends, businesses can develop highly precise revenue projections, optimize resource allocation, and make more informed, data-driven decisions.
However, for bottom-up forecasting to be truly effective, it requires a solid data foundation, consistent tracking of sales activities, and the use of advanced forecasting tools to automate calculations and enhance forecast accuracy. Without these elements, the reliability of bottom-up sales forecasts can be compromised.
For sales leaders aiming to refine their forecasting process, investing in AI-powered forecasting software, fostering cross-team collaboration, and continuously improving bottom-up models will be crucial. A disciplined approach to data-driven forecasting ensures that businesses stay agile, improve future sales performance, and drive sustained revenue growth in a competitive market.
Introduction
Accurate sales forecasting is one of the most critical aspects of B2B sales management, directly influencing future sales performance, revenue generation, and overall business growth. It enables sales leaders to set achievable revenue projections, allocate resources efficiently, and make data-driven strategic decisions. However, many organizations struggle with forecasting accuracy due to the limitations of traditional forecasting methods.
One commonly used approach is top-down forecasting, which relies heavily on high-level market trends, market research, and broad industry assumptions to estimate total sales. While this method provides a macro-level view, it often lacks precision because it doesn’t consider the detailed data analysis of current sales data, sales channels, or the actual performance of individual sales reps. This is where bottom-up forecasting provides a more reliable alternative.
Unlike the top-down approach, bottom-up forecasting focuses on analyzing historical data, individual sales reps' performance, and specific sales cycles to build a comprehensive and accurate forecast. This bottom-up approach begins at the granular level—using actual sales data from deals, pipelines, and sales teams—before scaling up to company-wide projections. The result is a forecasting process that reflects real, measurable activity and provides a more precise estimation of potential revenue.
In this guide, we will explore bottom-up forecasting in detail, explaining how it differs from top-down forecasting, its methodology, and how to implement it effectively. We will cover essential elements such as the bottom-up forecasting formula, real-world bottom-up forecasting examples, and the advantages and limitations of this bottom-up forecasting method.
Additionally, we will discuss alternative forecasting methods and best practices for improving forecast accuracy in B2B sales teams. Whether you are a sales leader, part of a management team, or a sales rep looking to refine your sales process, this guide will help you master bottom-up sales forecasting for more reliable and data-driven revenue projections.
What is Bottom-Up Forecasting?
Bottom-up forecasting is a sales forecasting method that generates revenue projections by analyzing detailed data at the ground level. Unlike top-down forecasting, which relies on broad market research, high-level assumptions, and macroeconomic indicators, bottom-up forecasting focuses on real, measurable activity within a company’s sales teams. This bottom-up approach starts with individual sales reps, specific deals, or even sales channels, aggregating these insights to create a more accurate forecast of future sales performance and potential revenue.
By leveraging current sales data, ongoing deals in the pipeline, and past sales cycles, companies can develop a highly tailored and precise forecasting process that reflects actual sales productivity. Since this method is rooted in real-time deal activity and historical data, it provides a dynamic, adaptable forecast that adjusts to changing conditions, making it particularly effective for improving forecast accuracy and revenue generation.
Key Characteristics of Bottom-Up Forecasting
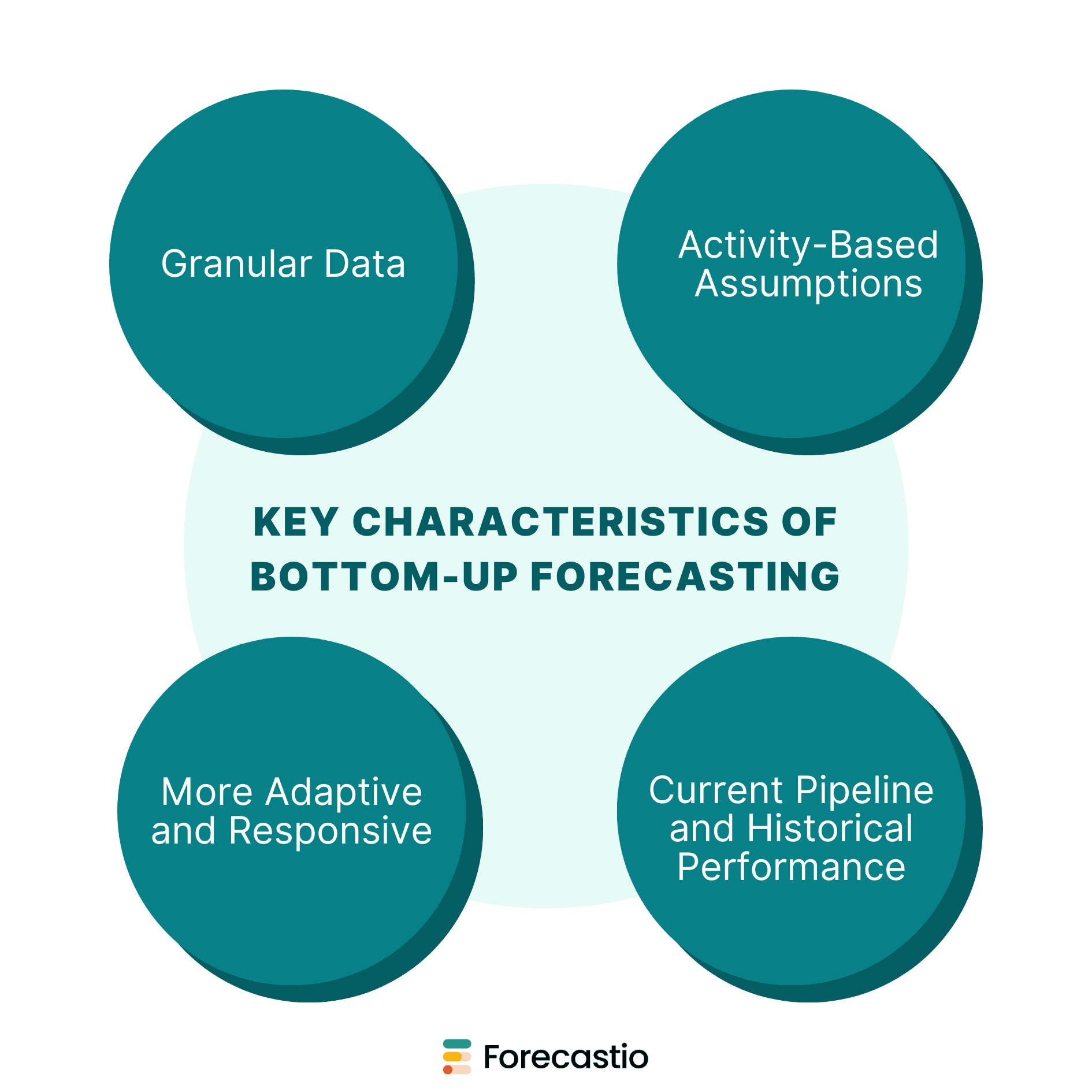
Granular Data: Unlike the top-down approach, which estimates total sales using broad industry figures, bottom-up forecasting relies on detailed data analysis from sales reps' performance, deal pipelines, and sales channels.
Activity-Based Assumptions: A bottom-up forecasting method considers specific sales process activities, such as outbound calls, booked demos, conversion rates, average deal size, and sales velocity, to generate more realistic revenue projections.
Current Pipeline and Historical Performance: A key element of bottom-up sales forecasting is its reliance on both historical data and current sales data from existing deals in the pipeline. By analyzing ongoing sales opportunities, it provides a real-time view of potential revenue, rather than relying solely on past performance.
More Adaptive and Responsive: Since bottom-up models are built on existing market data and current sales data, rather than external assumptions, they are highly adaptable. Companies can quickly adjust their forecasts when market conditions shift, ensuring a more dynamic and responsive forecasting process.
This bottom-up forecasting example demonstrates why many sales leaders prefer this method over top-down forecasting when looking to enhance forecast accuracy, optimize sales productivity, and make better-informed decisions based on real-time sales data.
If you want to get more detailed information about the most effective sales forecasting methods, read this article or download the ebook here.
Bottom-Up vs. Top-Down Forecasting
To understand the advantages of bottom-up forecasting, it’s essential to compare it with top-down forecasting and see how each forecasting method impacts forecast accuracy, adaptability, and data reliability.
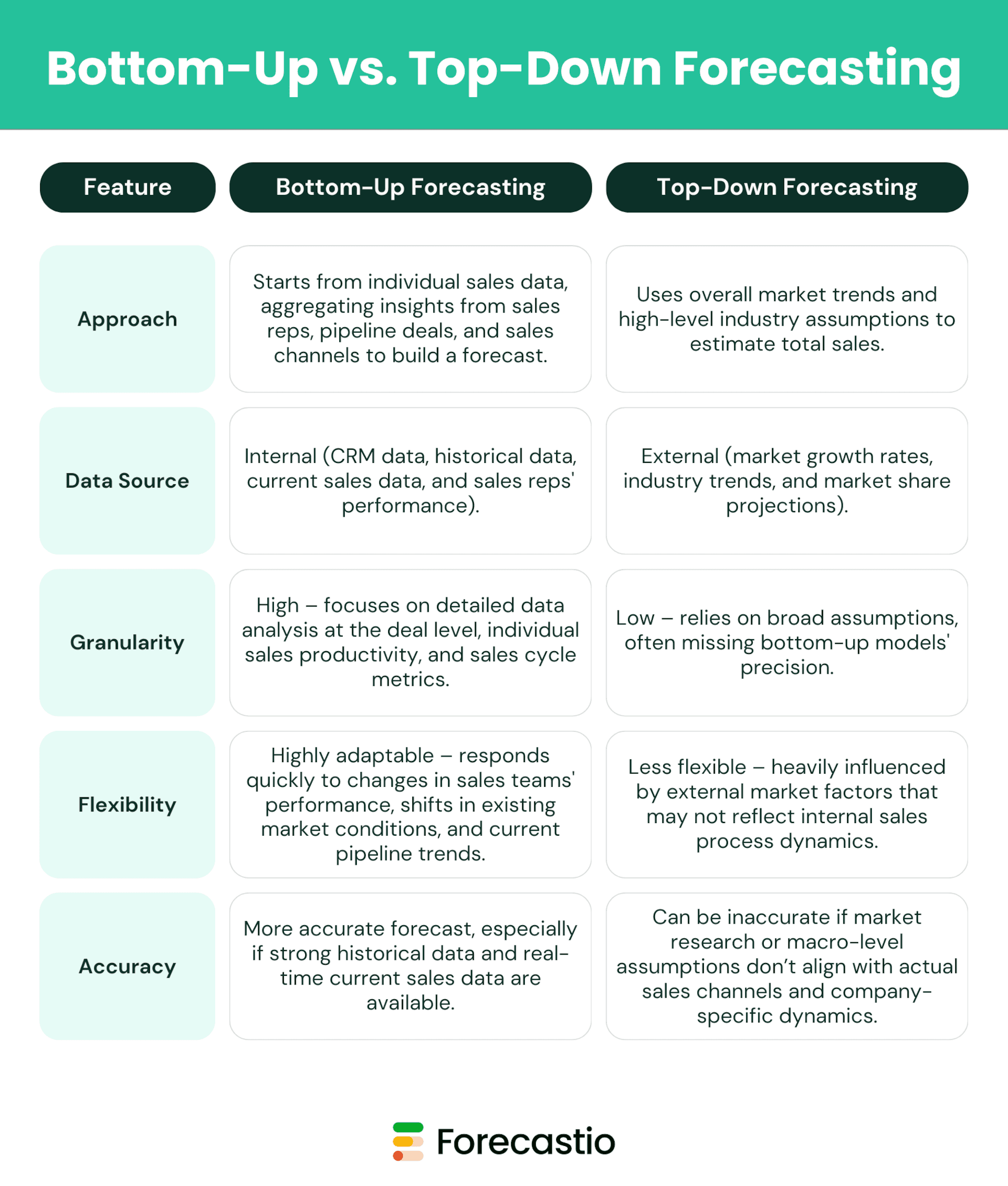
Why Bottom-Up Forecasting Is More Reliable for B2B Sales
For B2B companies, bottom-up forecasting is generally the preferred forecasting process, particularly in dynamic industries where external market share trends may not directly influence future sales performance. Since bottom-up forecasting focuses on real sales data from active pipeline deals, past sales cycles, and sales reps' productivity, it provides a more accurate forecast than the top-down approach, which can often overlook critical internal factors.
By leveraging bottom-up forecasting formulas and real-time bottom-up sales data, sales leaders can create revenue projections that reflect actual sales performance, leading to more precise planning, better sales productivity, and improved revenue generation.
How Bottom-Up Forecasting Works
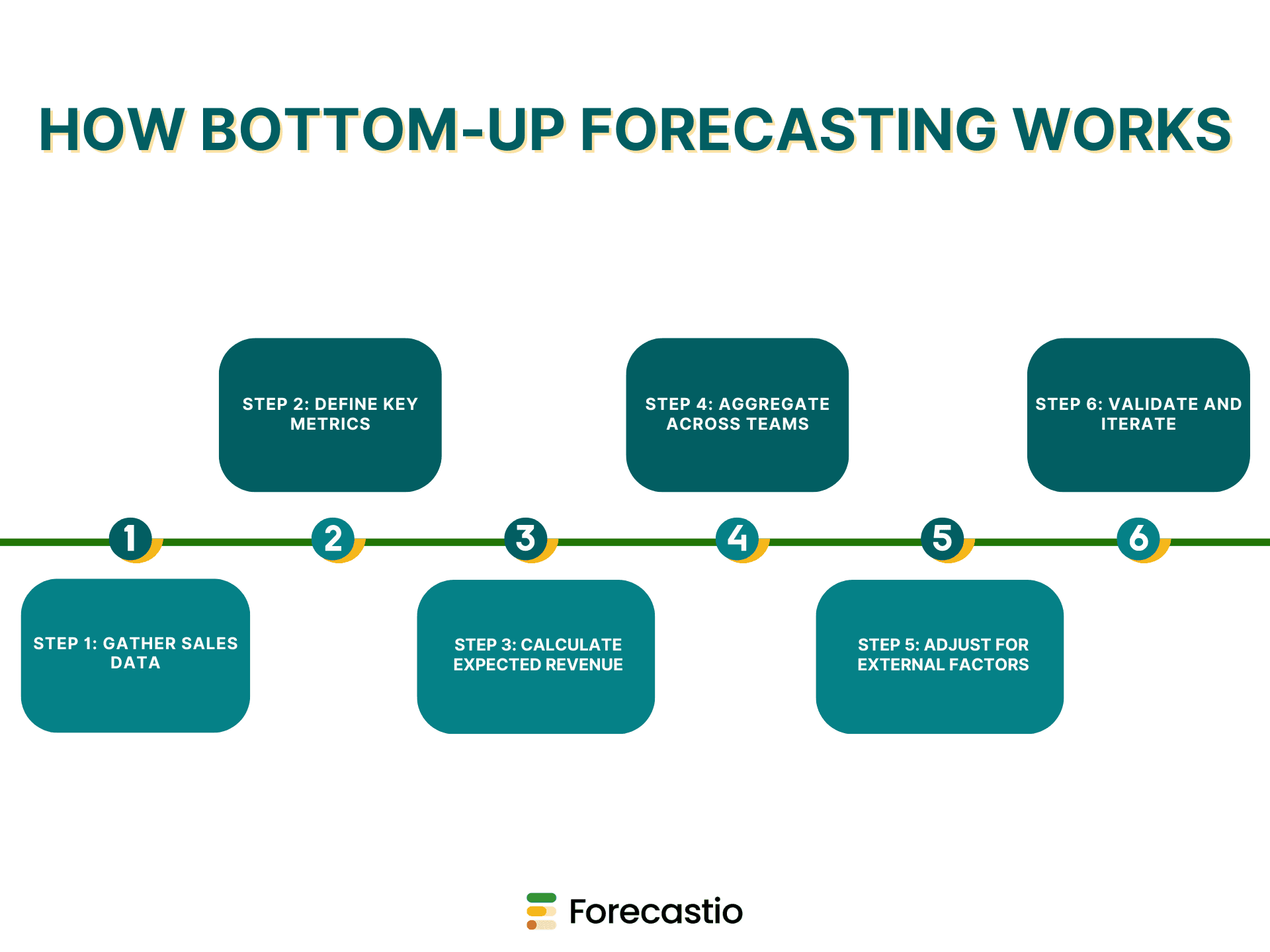
The bottom-up forecasting process follows a structured, data-driven methodology that aggregates insights from individual sales reps, current sales data, and historical data to generate a more accurate forecast. Unlike top-down forecasting, which starts with market research and broad assumptions, bottom-up forecasting focuses on granular, deal-level details to provide reliable revenue projections.
Below is a step-by-step approach to implementing bottom-up sales forecasting effectively:
1. Gather Sales Data
The foundation of bottom-up forecasting is detailed data analysis from sales reps, active deals in the pipeline, and historical data trends. Key data sources include:
Individual rep performance metrics (e.g., number of deals closed, conversion rates, outbound activity, demo-to-close ratio).
Pipeline analysis, including deal velocity, win rates by stage, and overall sales cycle length.
Revenue per account, factoring in past total sales and existing market penetration.
Sales channels and their contribution to revenue generation.
2. Define Key Metrics
To create an accurate forecast, specific sales performance indicators need to be established:
Number of sales reps actively closing deals.
Average quota attainment per rep.
Average deal size based on historical data and current sales data.
Sales cycle length, determining how long it takes for deals to close.
Win rate per stage, helping refine the bottom-up forecasting formula.
3. Calculate Expected Revenue
Once key metrics are identified, the next step is estimating potential revenue:
Determine the number of deals expected to close using historical performance and current pipeline data.
Assign deal probabilities based on pipeline stage (e.g., a deal in the proposal stage might have a 50% chance of closing, while a deal in negotiation might have an 80% probability).
Factor in sales reps’ commitments, as reps often provide their own estimates on which deals they believe will close.
Multiply the adjusted expected deal count by the average deal size to generate preliminary revenue projections.
Adjust calculations based on seasonality, pipeline trends, and variations in sales productivity.
4. Aggregate Across Teams
To transition from individual forecasts to an organizational sales forecast, data is rolled up across multiple levels:
Consolidate individual sales reps' forecasts into team-level estimates.
Scale forecasts across regional teams and company-wide sales projections.
Gather management team insights to refine projections based on market share, industry shifts, and internal strategic goals.
5. Adjust for External Factors
While bottom-up forecasting is primarily based on internal sales data, incorporating external elements improves forecast accuracy:
Consider industry trends, competitive landscape, and market research.
Factor in economic conditions that may impact future sales performance.
Align projections with internal growth expectations and alternative forecasting methods for comparison.
6. Validate and Iterate
The final step in the bottom-up forecasting method involves continuous improvement:
Compare forecasted revenue with actual sales performance.
Identify deviations and refine assumptions based on detailed data analysis.
Adjust the forecasting process as new sales data and pipeline insights become available.
By following this structured approach, sales leaders can develop a bottom-up forecasting model that provides accurate forecasts, enhances sales team performance, and supports more effective revenue generation strategies.
Elevate your forecasting precision by diving into our step-by-step guide to improve sales forecasting accuracy, packed with data-driven strategies and actionable best practices.
Bottom-Up Forecasting Examples
Example 1: Small SaaS Company
A SaaS company with five sales reps selling a single product follows this bottom-up forecasting method to estimate revenue:
Each sales rep makes 50 outreach calls per week.
Their call-to-demo conversion rate is 20% (1 in 5 calls results in a demo).
Their demo-to-close conversion rate is 20% (1 in 5 demos results in a sale).
The average deal size is $5,000.
Revenue Projection Calculation:
Each rep books 10 demos per week (50 outreach calls × 20% demo booking rate).
Each rep closes 2 deals per week (10 demos × 20% close rate).
The weekly revenue per rep is $10,000 (2 deals × $5,000 deal size).
With 5 sales reps, total expected weekly revenue is $50,000 (5 reps × $10,000 per rep).
For a quarter (13 weeks), the company forecasts $650,000 in revenue (50,000 × 13).
By aggregating individual rep forecasts, the company builds an accurate forecast based on actual sales productivity, rather than relying on market-wide assumptions.
Example 2: Large B2B Enterprise (Pipeline-Based Forecasting)
A multinational B2B enterprise selling multiple products across various regions applies a bottom-up forecasting approach based on pipeline data. Instead of relying on cold outreach activity, the forecast is built using deal probabilities and sales reps’ commitments.
Step 1: Forecast at the Sales Rep Level
Each sales rep commits to closing a specific number of deals based on their pipeline data and deal stage probabilities.
Sales rep pipeline at the start of the quarter:
5 deals in the Proposal stage (60% probability of closing).
8 deals in Negotiation (80% probability of closing).
12 deals in Early-stage pipeline (30% probability of closing).
Expected closed deals for the quarter:
Proposal stage: 5 × 60% = 3 closed deals.
Negotiation stage: 8 × 80% = 6 closed deals.
Early-stage pipeline: 12 × 30% = 4 closed deals.
Total expected closed deals: 13 per rep.
Average deal size per rep: $50,000.
Quarterly revenue projection per rep: $650,000 (13 deals × $50,000).
Step 2: Aggregate Across Teams & Products
The company operates in five regions, each with multiple teams selling three product lines.
Team A (10 reps):
Total committed closed deals: 130 (10 reps × 13 deals per rep).
Expected revenue for the team: $6.5M (130 deals × $50,000).
Team B, C, D, and E follow similar calculations, adjusting for deal probabilities, sales cycle variations, and regional factors.
Each product category (e.g., Enterprise Software, Data Security, AI Tools) has its own pipeline, allowing for precise bottom-up sales estimates per product line.
Final Aggregation for Company-Wide Forecast
By summing up forecasts across teams, regions, and product lines, the company produces a highly accurate forecast for the upcoming forecast period. This bottom-up forecasting method ensures that sales leaders base their revenue projections on actual sales data rather than external market research or broad assumptions.
What is Needed to Implement Bottom-Up Forecasting
Implementing bottom-up forecasting requires a structured approach, combining detailed data analysis, standardized processes, and collaboration across sales teams. Unlike top-down forecasting, which relies on market research and high-level estimates, bottom-up forecasting focuses on real sales data to generate an accurate forecast. To ensure a smooth forecasting process, companies need the following key components:
A Reliable CRM System – A robust CRM system is essential for tracking deals in the pipeline, monitoring sales reps' performance, and analyzing historical data. It should provide visibility into pipeline stages, win rates, and total sales, ensuring real-time access to current sales data.
Defined Sales Metrics – Companies must establish clear performance indicators, including conversion rates, quota attainment, average deal size, and sales cycle length. These metrics help create precise revenue projections and improve forecast accuracy.
A Standardized Process – A well-defined bottom-up forecasting method ensures consistency across sales teams. Standardizing how bottom-up models are built—including how sales reps' commitments and deal probabilities are factored in—helps create a reliable and repeatable forecasting process.
Collaboration Between Teams – Sales, marketing, and finance teams must align to ensure accurate revenue generation forecasts. Sales leaders provide bottom-up sales insights, marketing teams contribute market research, and finance teams validate revenue projections against company goals.
Forecasting Software – Advanced forecasting software, such as Forecastio, enhances forecast accuracy by automating bottom-up forecasting formulas, performing time series analysis, and integrating historical data with current sales data. These tools help sales leaders refine forecasts and make data-driven decisions.
By leveraging these elements, companies can develop a bottom-up forecasting approach that improves future sales performance, maximizes sales productivity, and drives better revenue projections.
Pros and Cons of Bottom-Up Forecasting
Pros:
More accurate for companies with detailed historical sales data - When bottom-up forecasting is built on high-quality historical data and current sales data, it delivers accurate forecasts that reflect actual sales productivity rather than broad assumptions.
Allows for granular adjustments – Since bottom-up models are based on individual sales reps' performance and deal-level data, forecasts can be adjusted dynamically to account for changes in pipeline trends, win rates, or quota attainment.
Provides clear insights into individual and team performance – By tracking sales reps' commitments, deal probabilities, and revenue projections, sales leaders gain a deeper understanding of team performance and future sales performance.
Cons:
Requires high-quality sales data – Bottom-up forecasting focuses on detailed data analysis, meaning unreliable or incomplete sales data can reduce forecast accuracy and impact revenue generation.
Can be time-consuming – Collecting and analyzing bottom-up sales data requires significant effort, especially for companies with large sales teams operating in multiple sales channels.
May not account for sudden market shifts as well as top-down forecasting – Since bottom-up forecasting is based primarily on internal sales data, it may lack the broad market research perspective of top-down forecasting, making it less responsive to unexpected market share changes or economic conditions.
Other Sales Forecasting Methods
In addition to bottom-up forecasting and top-down forecasting, companies can leverage alternative forecasting methods to enhance forecast accuracy and adapt to different business needs. Some of the most effective approaches include:
Pipeline-Based Forecasting – Uses current sales data from the deal pipeline, factoring in deal probabilities at each stage to create a data-driven revenue projection. This method ensures forecasts are aligned with existing market conditions and sales cycle dynamics.
Time Series Forecasting – Applies historical data trends and regression analysis to predict future sales performance. This method relies on statistical models, such as moving averages or exponential smoothing, making it useful for businesses with stable sales cycles and consistent historical data patterns.
AI-Based Forecasting – Utilizes machine learning algorithms to analyze complex sales data, identifying hidden patterns in sales productivity, market share, and revenue generation. AI models continuously improve by learning from past performance, enabling sales leaders to make highly accurate forecasts.
Scenario Planning – Creates multiple forecasting models based on varying assumptions about market conditions, sales process changes, or external economic factors. This method allows sales teams to prepare for different business scenarios and adjust their forecasting process accordingly.
By combining these alternative forecasting methods with bottom-up models, companies can enhance their sales forecasting strategy, improve forecast accuracy, and optimize revenue projections.
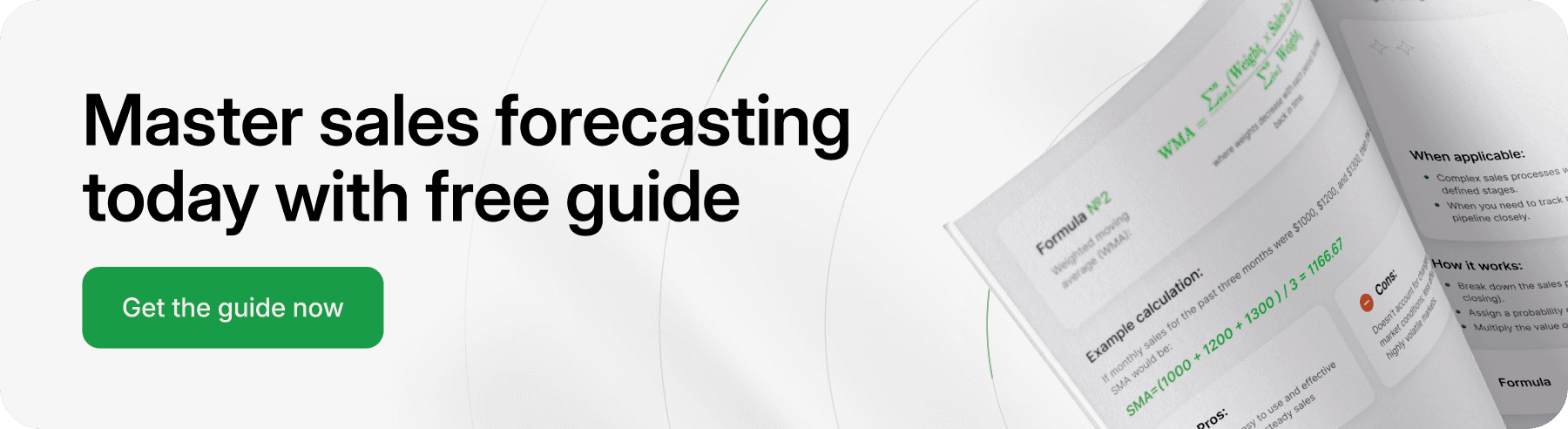
What Is Crucial to Improve Sales Forecasting Accuracy?
Achieving high forecast accuracy requires a combination of structured processes, reliable data, and collaboration across teams. To enhance sales forecasting, companies should focus on the following key areas:
Consistent Data Tracking – Ensure that all sales reps accurately log their activities, including deal updates, conversion rates, and sales cycle progress. Reliable current sales data is essential for improving bottom-up forecasting and making precise revenue projections.
Regular Forecast Reviews – Compare forecasted revenue with actual sales performance to identify gaps, refine assumptions, and improve the forecasting process. Incorporating historical data trends helps enhance forecast accuracy over time.
Cross-Team Collaboration – Align sales, marketing, finance, and operations to create a unified approach to revenue generation. Sales leaders must work closely with other departments to integrate market research, sales productivity insights, and total sales expectations into forecasting models.
Leveraging AI & Automation – Use forecasting software, such as AI-powered sales forecasting tools, to improve predictive accuracy. Machine learning models can analyze bottom-up sales data, recognize patterns, and enhance bottom-up models by accounting for external factors.
Continuous Training – Educate sales teams on best practices for pipeline management, quota attainment, and forecasting methods. Well-trained sales reps contribute to more reliable bottom-up forecasting formulas and ensure that forecasts are based on detailed data analysis rather than guesswork.
By implementing these best practices, companies can strengthen their sales forecasting process, enhance future sales performance, and drive more accurate forecasts for strategic decision-making.
Conclusion
Bottom-up forecasting is one of the most reliable and accurate forecasting methods for predicting sales performance and revenue generation in B2B companies. By leveraging granular data from individual sales reps, deal pipelines, and historical sales trends, businesses can develop highly precise revenue projections, optimize resource allocation, and make more informed, data-driven decisions.
However, for bottom-up forecasting to be truly effective, it requires a solid data foundation, consistent tracking of sales activities, and the use of advanced forecasting tools to automate calculations and enhance forecast accuracy. Without these elements, the reliability of bottom-up sales forecasts can be compromised.
For sales leaders aiming to refine their forecasting process, investing in AI-powered forecasting software, fostering cross-team collaboration, and continuously improving bottom-up models will be crucial. A disciplined approach to data-driven forecasting ensures that businesses stay agile, improve future sales performance, and drive sustained revenue growth in a competitive market.
Share:
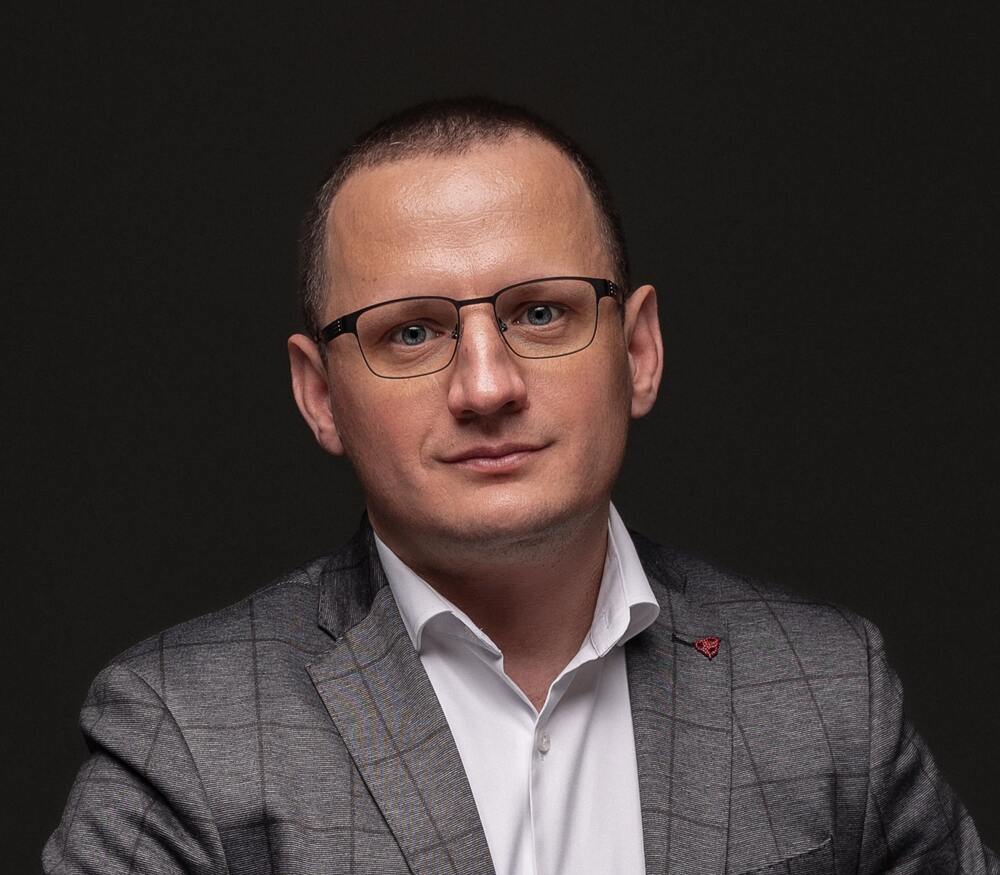
Alex is the CEO at Forecastio, bringing over 15 years of experience as a seasoned B2B sales expert and leader in the tech industry. His expertise lies in streamlining sales operations, developing robust go-to-market strategies, enhancing sales planning and forecasting, and refining sales processes.
Alex is the CEO at Forecastio, bringing over 15 years of experience as a seasoned B2B sales expert and leader in the tech industry. His expertise lies in streamlining sales operations, developing robust go-to-market strategies, enhancing sales planning and forecasting, and refining sales processes.
Related articles
Pipeline Management
Feb 17, 2025
9 min
Pipeline Management
Feb 17, 2025
9 min
Sales Performance
Feb 17, 2025
9 min
Sales Performance
Feb 17, 2025
9 min
Feb 17, 2025
9 min
Feb 17, 2025
9 min
Pipeline Management
Feb 17, 2025
9 min
Sales Performance
Feb 17, 2025
9 min
Pipeline Management
Feb 17, 2025
9 min
Sales Performance
Feb 17, 2025
9 min
Sales Planning
Sales Forecasting
Sales Performance Insights
Sales Planning
Sales Forecasting
Sales Performance Insights
Sales Planning
Sales Forecasting
Sales Performance Insights
© 2025 Forecastio, All rights reserved.
Sales Planning
Sales Forecasting
Sales Performance Insights
Sales Planning
Sales Forecasting
Sales Performance Insights
Sales Planning
Sales Forecasting
Sales Performance Insights
© 2025 Forecastio, All rights reserved.
Sales Planning
Sales Forecasting
Sales Performance Insights
Sales Planning
Sales Forecasting
Sales Performance Insights
Sales Planning
Sales Forecasting
Sales Performance Insights
© 2025 Forecastio, All rights reserved.
Sales Planning
Sales Forecasting
Sales Performance Insights
Sales Planning
Sales Forecasting
Sales Performance Insights
Sales Planning
Sales Forecasting
Sales Performance Insights
© 2025 Forecastio, All rights reserved.