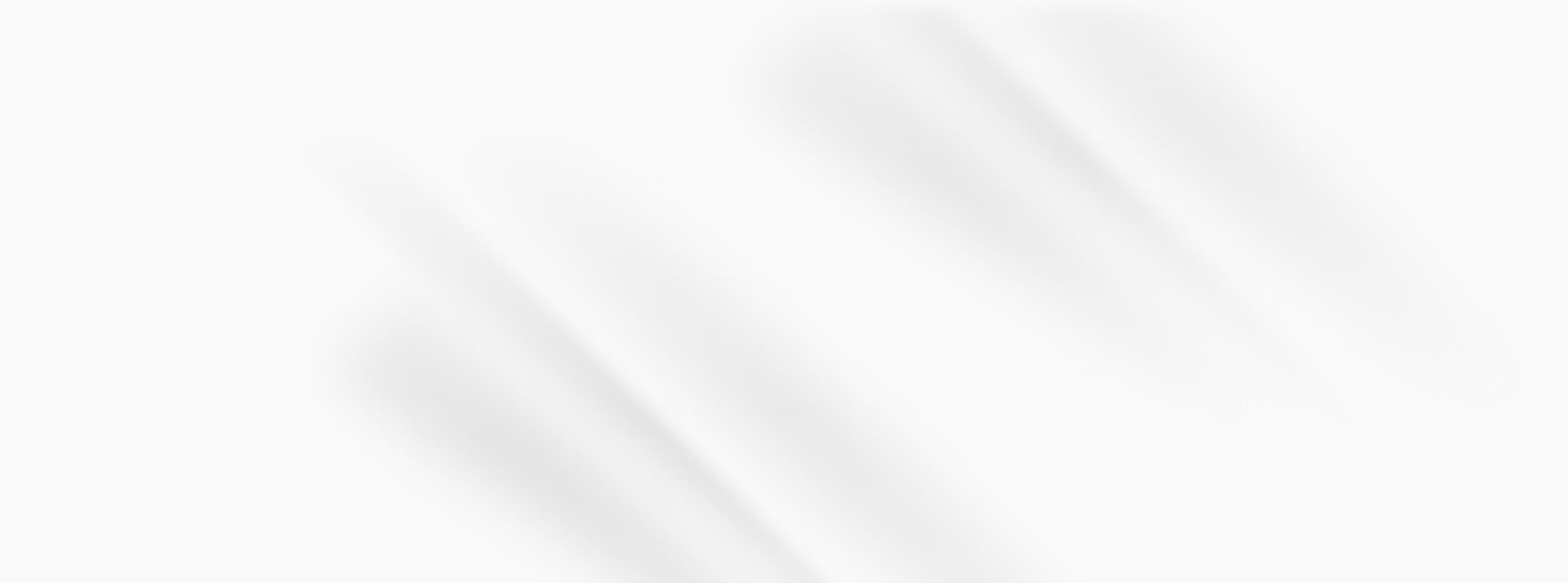
A Complete Guide to Sales Forecasting Methods
Feb 17, 2025
Feb 17, 2025
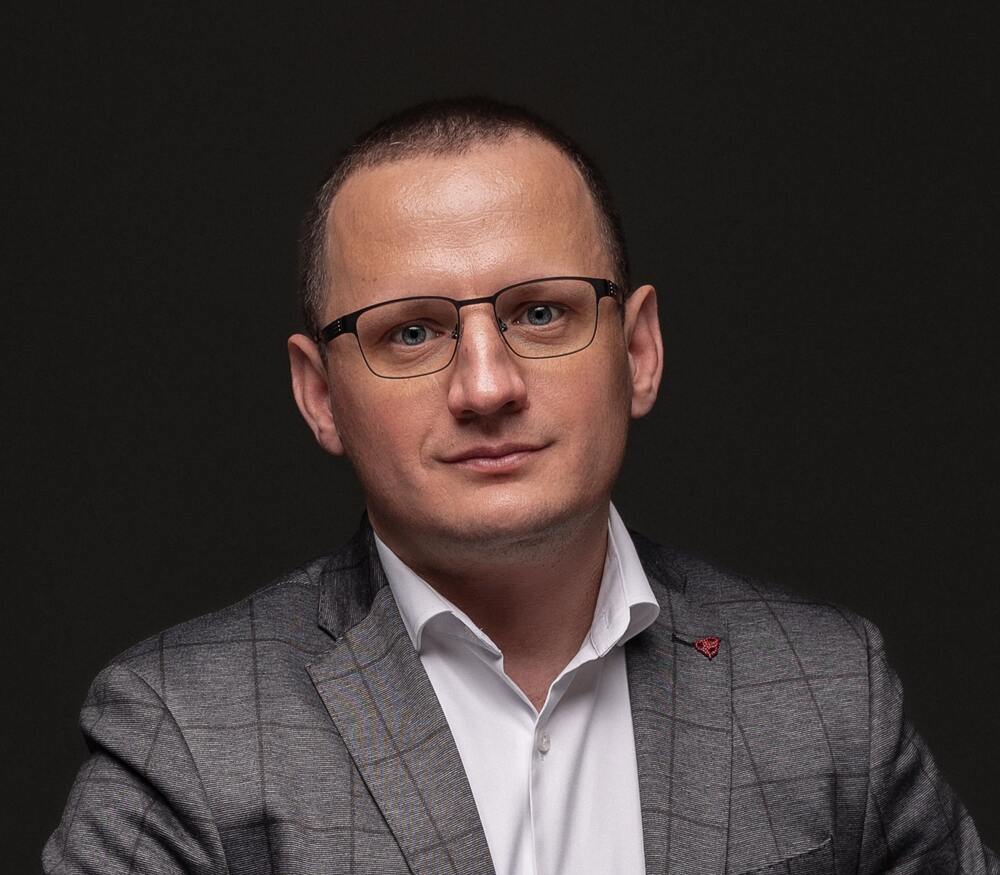
Alex Zlotko
CEO at Forecastio
Last updated
Feb 17, 2025
Reading time
21 min
Share:
Share
Table of Contents
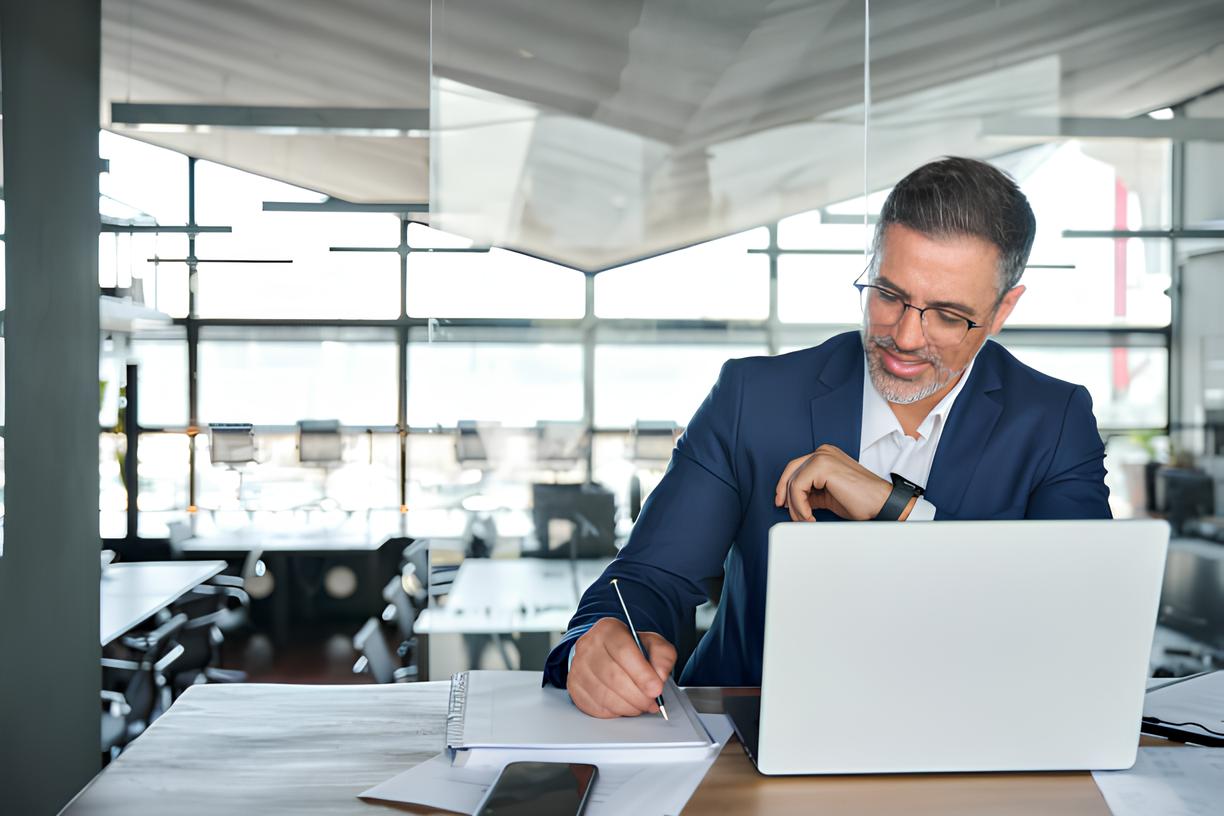
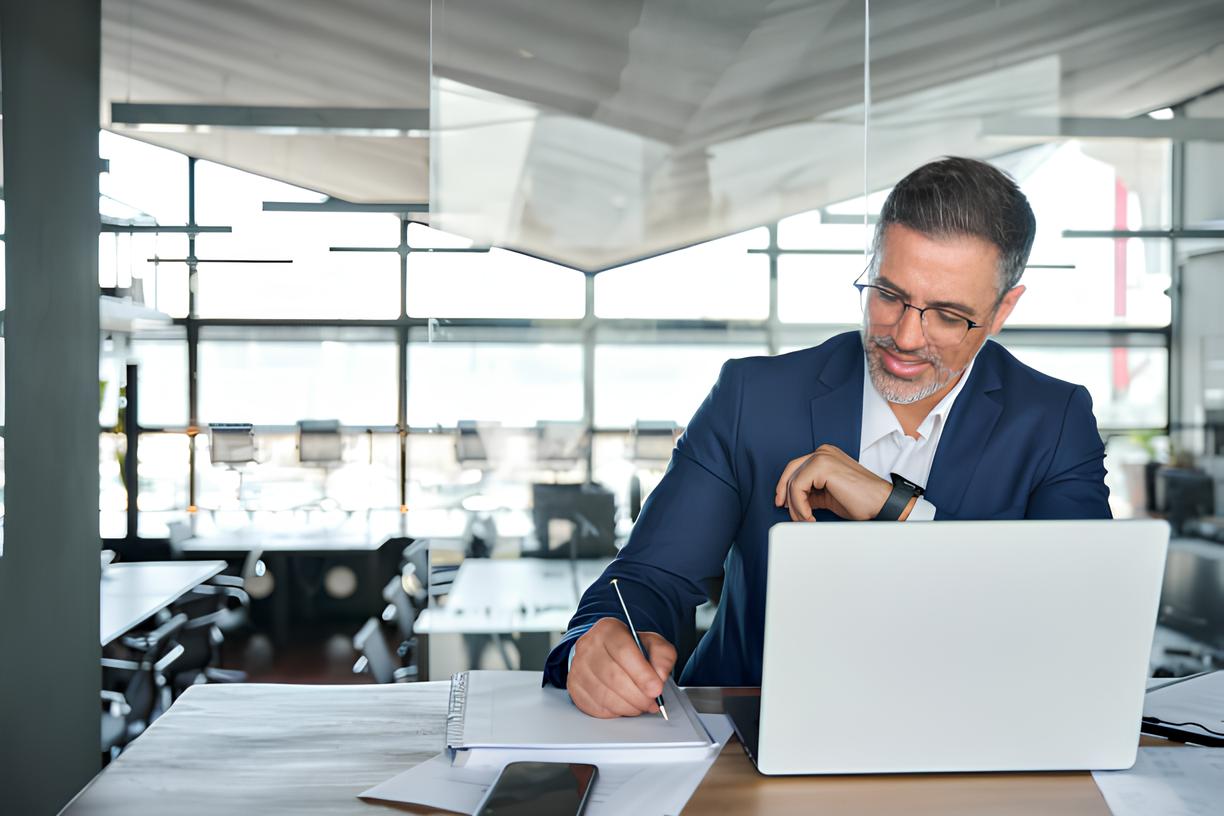
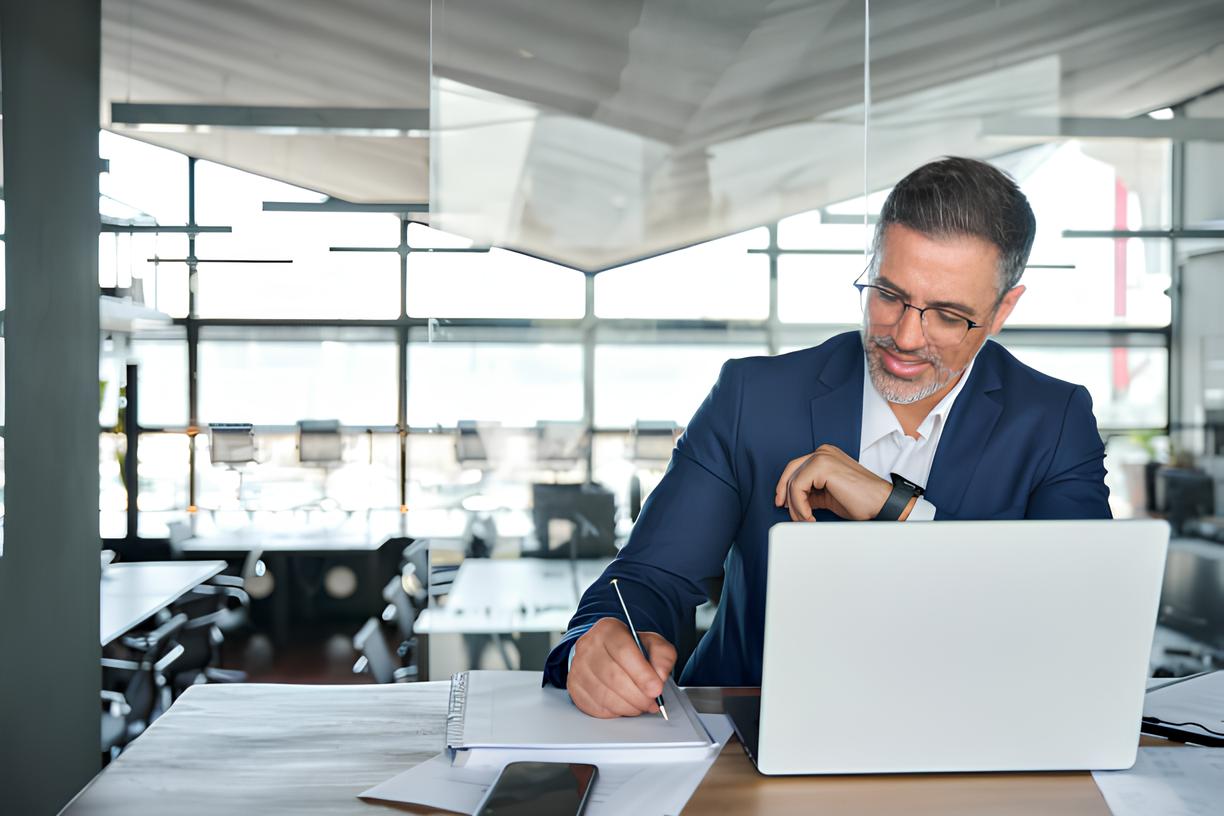
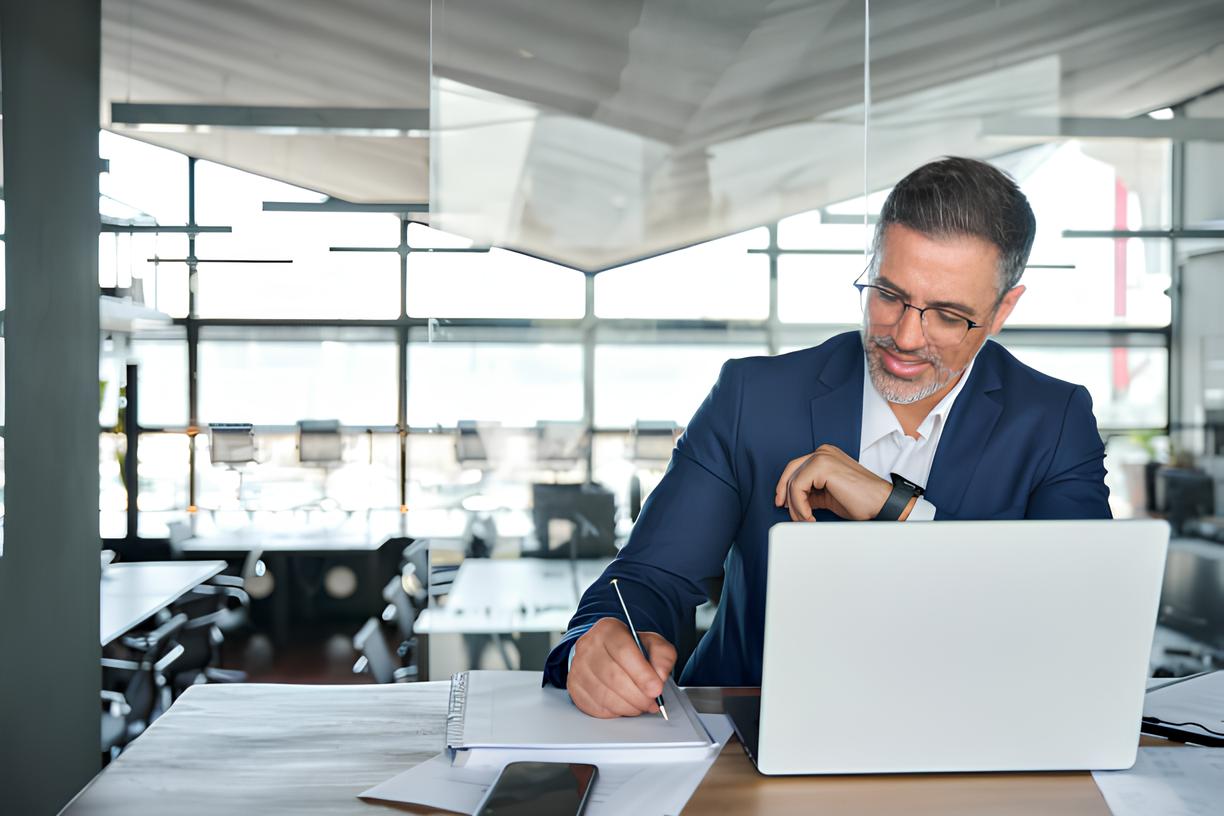
Quick Take
Quick Take
Sales forecasting directly impacts your revenue and business survival.
Over 50% of sales leaders lack confidence in their forecasts, but using the right method can fix this.
Start with bottom-up forecasting – look at individual deals and reps first, then build up to total projections.
Track deal stages and assign close probabilities (like 30% for discovery, 80% for negotiation).
For new businesses without history, gather insights from sales reps and market experts.
Avoid messy data and outdated spreadsheets – they kill accuracy.
Use forecasting software that connects to your CRM for real-time updates.
Sales forecasting directly impacts your revenue and business survival.
Over 50% of sales leaders lack confidence in their forecasts, but using the right method can fix this.
Start with bottom-up forecasting – look at individual deals and reps first, then build up to total projections.
Track deal stages and assign close probabilities (like 30% for discovery, 80% for negotiation).
For new businesses without history, gather insights from sales reps and market experts.
Avoid messy data and outdated spreadsheets – they kill accuracy.
Use forecasting software that connects to your CRM for real-time updates.
Introduction
Sales forecasting has become a cornerstone of modern sales strategy, frequently appearing in countless articles, LinkedIn posts, and influencer blogs. Despite its widespread discussion among industry experts and the wealth of resources available, many organizations still struggle to achieve accurate sales forecasts. According to the Gartner State of Sales Operation Survey, over 50% of sales leaders express a lack of confidence in their sales forecasting accuracy—a gap that often leads to misaligned goals and missed opportunities.
From my own experience, sales forecasting has always been a challenging yet essential task. The ability to predict future sales not only drives strategic planning but also ensures better resource allocation, budget planning, and revenue forecasting. However, mastering sales forecasting requires more than just intuition—it demands time, effort, internal buy-in, and the right sales forecasting software.
In this article, we’ll explore why achieving accurate sales forecasting is so critical for B2B organizations. We’ll examine the best sales forecasting methods available, such as regression analysis, historical sales data forecasting, and qualitative forecasting, while also addressing the common pitfalls that sales leaders, sales reps, and RevOps teams encounter during the sales forecasting process. By understanding these challenges and leveraging historical sales data effectively, organizations can improve sales forecasting accuracy and drive better business outcomes.
The definition of sales forecasting
Sales forecasting is the practice of predicting future sales by analyzing various data points, such as historical sales data, current market trends, sales pipeline performance, and broader economic indicators. This forecasting process is vital for businesses to anticipate future revenue, enabling strategic planning, resource allocation, and improved sales performance. Accurate sales forecasting helps businesses stay ahead of market fluctuations and ensures they make informed decisions.
The responsibility for creating an accurate sales forecast often depends on the company's size, organizational structure, and stage of development. In startups and small businesses, the VP of Sales or Head of Sales typically oversees the sales forecasting process. These sales leaders rely on their intimate understanding of the sales pipeline and past sales data to create intuitive forecasts. This hands-on approach allows smaller teams to adapt quickly to changing conditions and provides flexibility during the early stages of growth.
As companies expand, their forecasting needs grow more complex. At this stage, sales forecasting is often handed over to dedicated teams such as Sales Operations or Revenue Operations (RevOps). These teams leverage advanced sales forecasting models, like regression analysis and multivariable analysis forecasting, to improve sales forecasting accuracy. They gather relevant data from historical sales figures, current market trends, and opportunity stage forecasting to align sales projections with the company’s broader strategic goals. The formation of such teams reflects a company’s evolution toward a data-driven forecasting process, ensuring accurate forecasts that drive strategic planning.
For larger organizations, sales forecasting becomes even more critical. With higher annual revenues, bigger budgets, and diverse sales teams, forecasting accuracy plays a pivotal role in maintaining operational efficiency and meeting stakeholder expectations. In these scenarios, relying on the right sales forecasting methods—such as time series analysis, AI forecasting, and qualitative forecasting —becomes essential. Inaccurate sales forecasting can lead to resource mismanagement, missed sales opportunities, and decreased revenue, underlining the importance of improving sales forecasting accuracy.
In summary, sales forecasting is a dynamic function that evolves with a company’s growth. While initially managed by sales leaders in smaller businesses, it transitions to specialized teams as organizations scale. Whether you're using sales forecasting software or leveraging qualitative sales forecasting techniques, understanding this progression is key to predicting future sales accurately and supporting long-term business success.
The difference between sales forecasting and sales planning
Sales forecasting and sales planning are two fundamental processes in any sales organization. While they are closely related, they serve distinct purposes and operate within different time frames. Together, they form a comprehensive approach to predict future sales and drive business success. Understanding the differences between these two concepts is crucial for sales leaders, sales managers, and RevOps teams aiming to improve sales performance and align strategic goals.
Sales Forecasting vs. Sales Planning: A Detailed Comparison
Sales forecasting focuses on predicting future sales revenue by analyzing historical sales data, market trends, and the sales pipeline. This process is best suited for short-term predictions, such as monthly or quarterly sales projections. Accurate forecasts help businesses allocate resources, improve sales forecasting accuracy, and adapt quickly to real-time changes in the market.
On the other hand, sales planning takes a broader, long-term perspective. It involves setting strategic sales targets, estimating the resources needed to achieve those goals, and preparing for various scenarios that could impact future revenue. Sales planning often includes what-if analyses to simulate potential market changes, shifts in sales performance, or other influencing factors.
Here’s a side-by-side comparison to highlight the key differences:
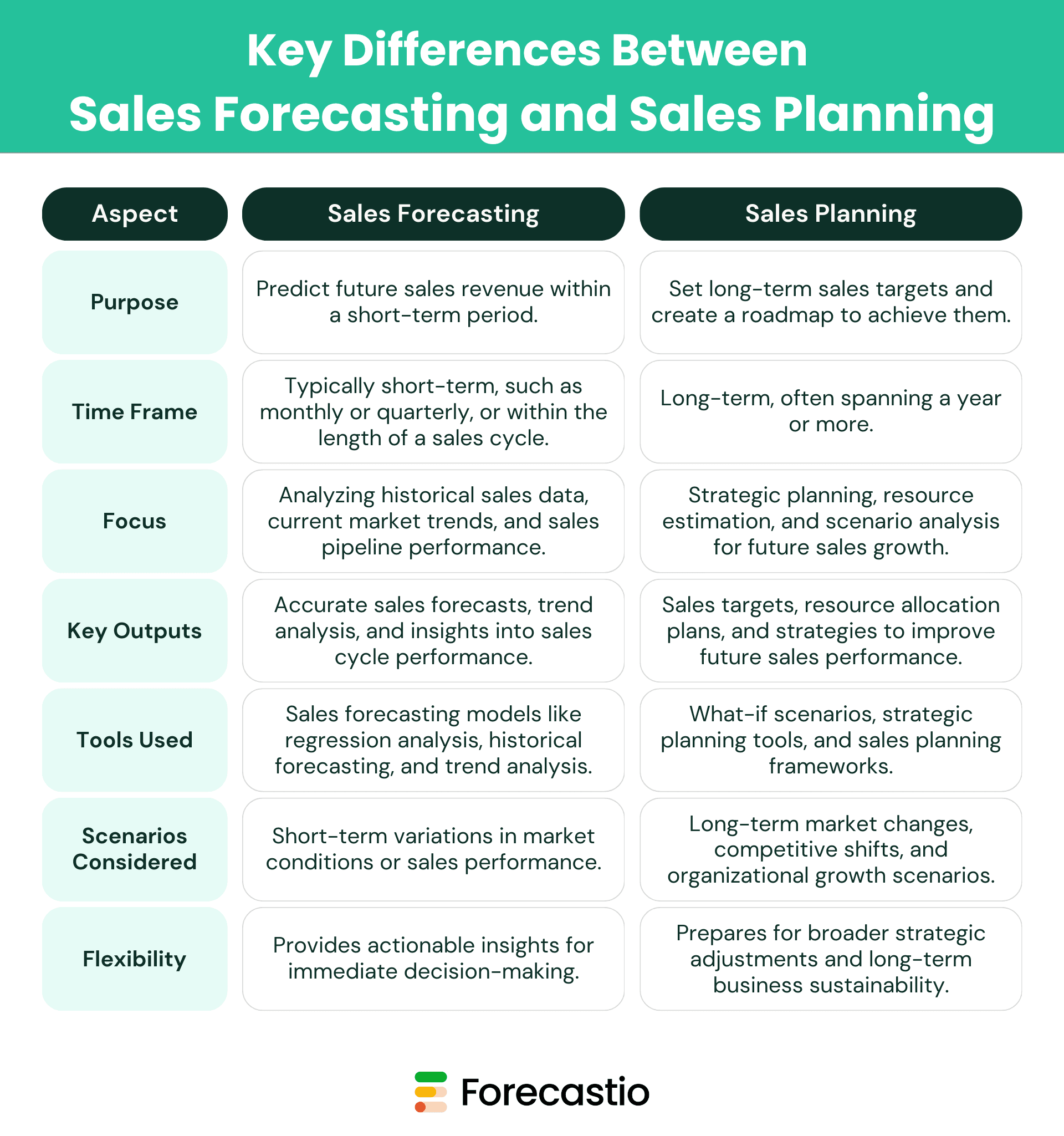
When to Use Sales Forecasting vs. Sales Planning
Sales forecasting is ideal for organizations that need to monitor sales performance closely and adjust their strategies in real time. It helps sales teams predict future sales within manageable time frames, ensuring efficient resource allocation and accurate forecasts.
Sales planning, on the other hand, is critical for companies aiming to set ambitious long-term goals and align their teams around a cohesive strategy. By considering potential market trends and future sales conditions, sales planning provides a robust framework for sustained growth and success.
Conclusion
While sales forecasting and sales planning are complementary processes, understanding their differences allows sales leaders, RevOps teams, and sales managers to use them effectively. Combining short-term forecasting accuracy with long-term strategic planning ensures businesses can navigate immediate challenges while staying focused on achieving their future sales targets.
By integrating accurate sales forecasting and comprehensive sales planning into their sales process, organizations can improve sales forecasting accuracy, anticipate future revenue, and drive long-term business success.
The importance of sales forecasting
"Accurately Predicting Sales is Impossible"—Is it Really?
Have you ever heard statements like, "Accurately predicting sales is impossible in this fast-changing market" or "As a startup, we’re too small to worry about forecasting; our priority is acquiring new accounts"? You’re not alone. Many businesses, especially startups, underestimate the vital role of accurate sales forecasting in ensuring long-term success.
To understand the significance of an accurate sales forecast, let’s explore the consequences of poor sales forecasting and its impact on business operations and growth:
1. Inefficient Resource Allocation
Inaccurate sales forecasts can lead to mismanagement of resources. Companies often overspend on marketing campaigns, hiring, sales tools, or product innovations, which drives up costs without improving efficiency. This misallocation not only reduces profitability but also increases cash burn—especially critical for startups operating on tight budgets.
2. Investor Confidence and Fundraising Challenges
Poor sales forecasting is often seen as a reflection of weak understanding of market trends, sales data, and revenue potential. Investors depend on reliable sales forecasting models to assess growth opportunities and risks. Overly optimistic or inconsistent sales projections can erode trust, damaging the company’s credibility and hindering its ability to raise funds.
3. Limited Investments in Growth and Innovation
Consistently missing sales projections creates unpredictability and reduces confidence in future forecasts. This uncertainty often forces businesses to prioritize managing cash risks over growth initiatives, such as scaling teams, investing in innovation, or experimenting with new sales strategies. Accurate forecasting is essential to balance these priorities effectively.
4. Leadership Credibility and Team Morale
Whether created by a Sales Leader, RevOps team, or Sales Operations department, inaccurate sales forecasting undermines leadership credibility. Missed revenue targets can highlight deficiencies in forecasting processes, historical sales data, tools, or team alignment. This not only casts doubt on leadership effectiveness but also negatively impacts team morale and sales performance.
Recognizing the importance of accurate sales forecasting is critical for business sustainability and growth. Leveraging the right sales forecasting methods can significantly improve sales forecasting accuracy.
Now, let’s delve into the best forecasting methods and models used by B2B sales organizations to predict future sales, align resources, and achieve reliable sales projections.
Sales forecasting methods
Sales forecasting is a critical aspect of strategic planning and revenue management, but there’s no universal solution. The 'one size fits all' approach simply doesn’t apply to sales forecasting methods.
Every company is unique, with its own set of influencing factors, such as:
The amount of historical sales data
The maturity of the sales process
The sales model (e.g., subscription-based, enterprise sales, or transactional sales)
Market dynamics and trends
The specific industry
These factors must be carefully considered when selecting the right sales forecasting method. Choosing a method that aligns with your business's structure and objectives can significantly improve sales forecasting accuracy and drive actionable insights.
Top-Down Sales Forecasting
Top-down sales forecasting begins with the big picture, analyzing overall market conditions, industry trends, and historical revenue data to predict future sales. This approach involves:
Assessing key market indicators, such as demand fluctuations and competitive dynamics.
Analyzing broad trends to estimate the total addressable market (TAM) and the company’s potential market share.
Using historical sales data and performance trends to refine projections.
This method works well for companies seeking to align sales strategies with macroeconomic conditions or when little granular data is available from sales reps. However, its effectiveness relies heavily on accurate trend analysis and a deep understanding of the market.
Bottom-Up Forecasting
In contrast, bottom-up forecasting starts at the individual level. This approach focuses on aggregating forecasts from sales reps, territories, or verticals to create a comprehensive forecast for the entire business. Key elements include:
Collecting individual sales projections during one-on-one meetings with sales reps.
Leveraging sales pipeline data and opportunity stage forecasting to assess potential revenue.
Summing up forecasts across teams, customer segments, or regions to provide a holistic sales projection.
Bottom-up forecasting benefits from the deep knowledge of sales reps, who are often closest to the customer and pipeline activity. This method is particularly effective for companies with a well-established sales process and a strong understanding of historical sales performance at the micro level.
Historical Sales Data Forecasting
Historical sales data forecasting is one of the most straightforward and widely used sales forecasting methods. It relies on analyzing past sales data to identify trends, seasonality, and patterns that can be used to predict future sales. This method is especially effective for companies with reliable and consistent historical revenue data and works well when external conditions, such as the market and industry trends, remain relatively stable.
When is Historical Sales Data Forecasting Applicable?
This method is best suited for:
Businesses with several years of historical sales data.
Stable industries with predictable sales cycles and minimal market disruption.
Companies looking for a straightforward approach to generate accurate sales forecasts without relying on complex models.
Scenarios where identifying seasonal trends or long-term patterns is necessary for resource planning, inventory management, and target setting.
However, it may not be ideal for businesses experiencing rapid changes in their sales process, market dynamics, or customer behavior, as it assumes past trends will continue into the future.
How Historical Forecasting Works
At its core, historical forecasting involves analyzing past sales performance to estimate future outcomes. Common techniques include the Simple Moving Average (SMA) approach.
Formula:
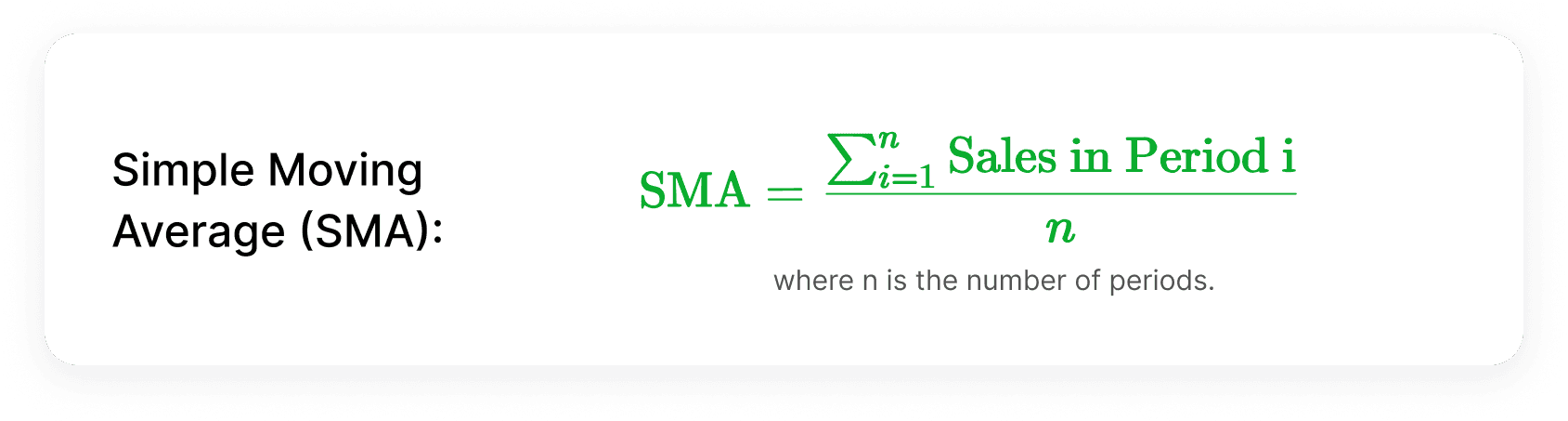
Where:
n = Number of periods used in the calculation.
Sales values are taken from historical sales data.
Example
A company wants to forecast sales for April using the sales data from January, February, and March:
January sales = $10,000
February sales = $12,000
March sales = $11,000
Using the SMA formula:
SMA for April = (10,000 + 12,000 + 11,000) / 3 = 11,000
The forecasted sales for April are $11,000
Opportunity Stage Forecasting
Opportunity stage forecasting is a sales forecasting method that evaluates deals in the sales pipeline based on their stage within the sales cycle. By assigning probabilities to each stage, businesses can estimate the likelihood of closing deals and generate more accurate sales forecasts. This method is particularly effective for companies with a well-defined sales process and a structured sales pipeline.
When is Opportunity Stage Forecasting Applicable?
This method works well for:
Companies with a mature sales process and clearly defined pipeline stages.
Businesses looking for a deal-by-deal approach to forecast future sales revenue.
Sales teams that rely on consistent sales rep input and CRM systems to track opportunities.
Scenarios where management wants to assess pipeline health and prioritize high-probability deals.
Opportunity stage forecasting is most applicable in environments with complex sales cycles, where deals progress through multiple stages before closing.
How Opportunity Stage Forecasting Works
Steps to Perform Opportunity Stage Forecasting
Define Sales Pipeline Stages
Clearly outline stages in the sales process, such as "Prospecting," "Discovery," "Proposal Sent," and "Negotiation."
Assign Probabilities to Each Stage
Assign a probability of closing for each stage based on historical data. For example:
Prospecting: 10%
Discovery: 30%
Proposal Sent: 60%
Negotiation: 80%
Closed-Won: 100%
Calculate Weighted Revenue for Each Opportunity
Multiply the deal value by the probability associated with its current stage.
Sum the Weighted Revenues
Add up all weighted revenues to forecast total pipeline revenue.
Example
A company has the following opportunities in its sales pipeline:
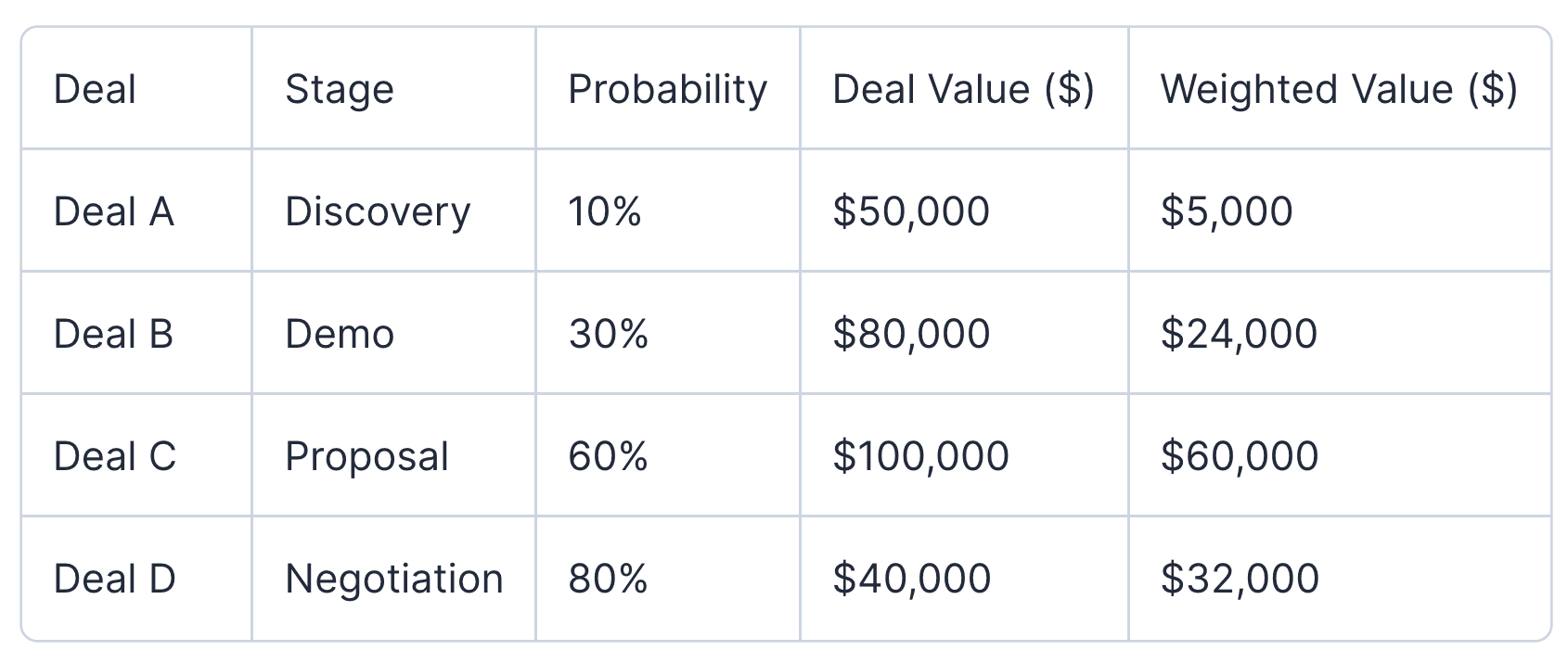
Total Forecasted Revenue = $5,000 + $24,000 + $60,000 + $32,000 = $121,000
Length of Sales Cycle Forecasting
Length of sales cycle forecasting is a method that predicts future sales revenue by analyzing the average time it takes for deals to progress through the sales cycle and close. By understanding the typical duration of a sales cycle, businesses can estimate when current opportunities are likely to close and generate accurate sales forecasts.
When is Length of Sales Cycle Forecasting Applicable?
This method is particularly effective for:
Companies with consistent and well-documented sales cycles.
Businesses tracking the time deals spend in each pipeline stage.
Organizations with repetitive or recurring sales processes, such as B2B subscription models.
Sales teams seek to predict revenue timing based on pipeline activity and deal velocity.
This approach works best in environments where the length of the sales cycle is stable and not influenced by external variables, such as market disruptions or significant changes in sales strategies.
How Length of Sales Cycle Forecasting Works
This method calculates the average duration of the sales cycle from initial contact to deal closure and applies this duration to current opportunities to estimate when revenue will be realized.
Steps to Perform Length of Sales Cycle Forecasting:
Analyze Historical Sales Data. Use historical revenue data to determine the average sales cycle length for past deals.
Segment Deals by Similar Characteristics. Group deals by size, industry, customer segment, or other relevant factors to identify patterns in the sales cycle.
Apply the Average Cycle Length to Current Opportunities. For each deal in the pipeline, estimate the closing date based on its entry date and the average cycle length.
Calculate Forecasted Revenue by Period. Sum the values of deals expected to close within a specific time frame (e.g., monthly or quarterly).
Limitations
Assumes sales cycle lengths remain consistent, which may not be true in dynamic markets.
Requires accurate tracking of deal entry dates and sales cycle duration.
Less effective for businesses with highly variable or undefined sales cycles.
To learn more about this method and explore real examples with numbers, download the eBook “13 Sales Forecasting Methods.
Lead-Driven Sales Forecasting
Lead-driven forecasting is a sales forecasting method that uses the volume of leads entering the sales pipeline and their historical conversion rates to estimate future sales revenue. This method ties sales projections directly to the performance of marketing and sales efforts, making it an essential tool for businesses with a high focus on lead generation and nurturing strategies.
When is Lead-Driven Forecasting Applicable?
Lead-driven forecasting is particularly effective for:
Companies with a predictable sales process and well-documented lead conversion rates.
Businesses that generate a high volume of leads through consistent sales strategies or marketing campaigns.
Organizations with a clear understanding of how lead quantity and quality impact sales performance.
Teams seek to predict future revenue based on the volume and behavior of leads entering the pipeline.
This method works best for companies with stable lead sources and consistent sales cycle durations.
How Lead-Driven Forecasting Works
This approach predicts future sales revenue by analyzing the following factors:
Lead Volume: The number of leads entering the pipeline within a specific period.
Conversion Rate: The historical percentage of leads that convert into closed deals.
Average Deal Value: The typical revenue generated from a single deal.
By multiplying these factors, businesses can estimate their total future sales revenue.
Formula:
Forecasted Revenue = Lead Volume X Conversion Rate X Average Deal Value
Limitations
Assumes consistent lead quality, which may not always be the case.
Relies on accurate tracking of leads and historical conversion data.
Less effective for companies with long or unpredictable sales cycles.
External factors, such as market changes or competitive dynamics, may impact actual sales outcomes.
To learn more about this method and explore real examples with numbers, download our free Sales Forecasting Guide.
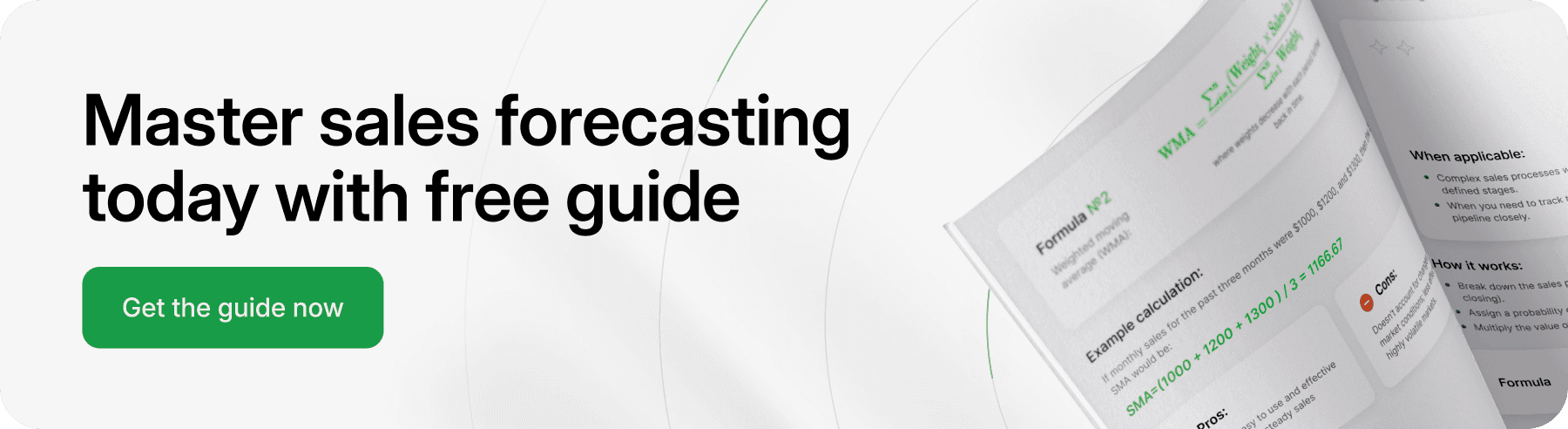
Forecasting based on deal probabilities
Forecasting based on deal probabilities is a method that predicts future sales revenue by assigning a probability of closure to each deal in the sales pipeline. This approach provides a granular, data-driven forecast that takes into account the likelihood of individual deals closing.
When is Forecasting Based on Deal Probabilities Applicable?
This method is particularly effective for:
Companies with a structured sales process and detailed deal tracking.
Businesses that manage a variety of deals with differing sizes, stages, and closing probabilities.
Teams looking to generate accurate sales forecasts by assessing the health of the sales pipeline at an individual deal level.
Organizations aiming to improve sales forecasting accuracy by incorporating real-time data into the forecasting process.
How Forecasting Based on Deal Probabilities Works
The method involves calculating the weighted value of each deal by multiplying its value by its probability of closing. These weighted values are then summed to create the total forecasted revenue.
Steps to Perform Deal Probability Forecasting:
Assign Probabilities to Each Deal. Determine the likelihood of each deal closing based on its stage in the sales cycle or other relevant factors.
Calculate Weighted Revenue. Multiply the deal value by its probability to determine its contribution to the forecast.
Sum the Weighted Values. Add up all weighted values to calculate the total forecasted revenue.
Example
A company has the following deals in its sales pipeline:
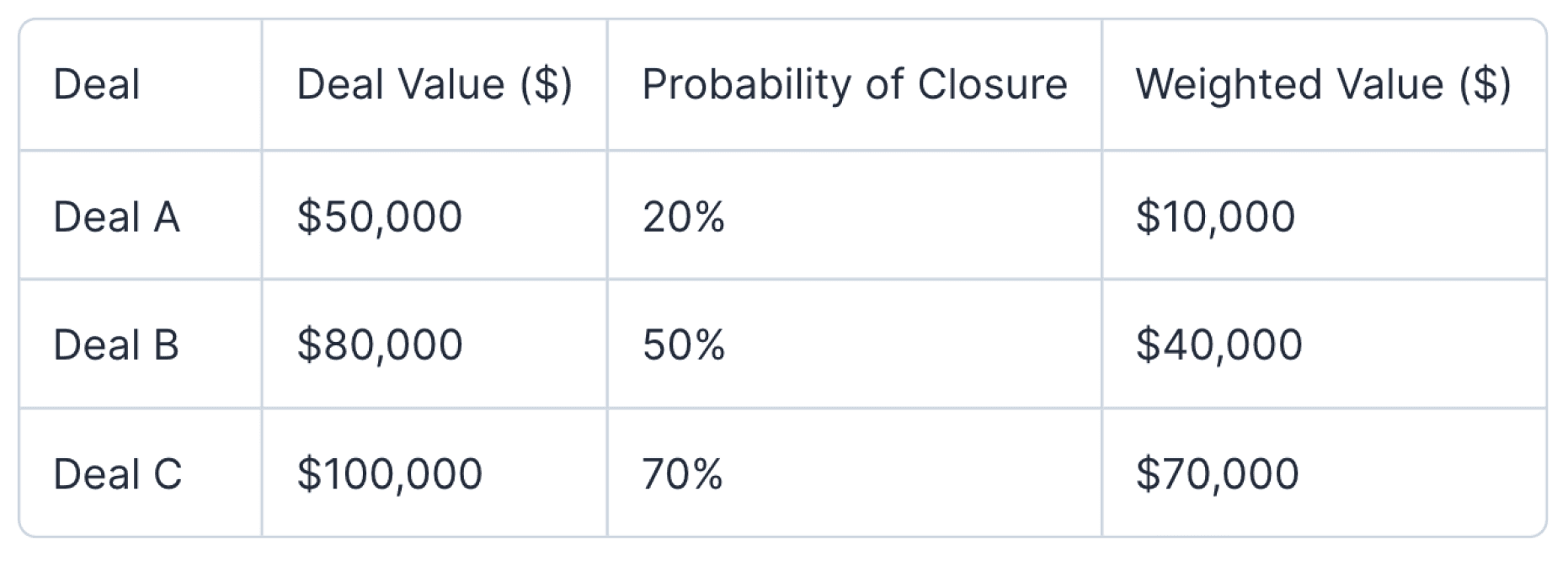
Forecasted Revenue = $10,000 + $40,000 + $70,000 = $120,000
Time Series Analysis
Time series analysis is a sales forecasting method that uses historical sales data to identify patterns, trends, and seasonality over time. By analyzing these recurring patterns, businesses can make data-driven predictions about future sales performance. This method is particularly effective for organizations with consistent sales data over an extended period and is widely used to create accurate forecasts.
When is Time Series Analysis Applicable?
This method is ideal for:
Companies with sufficient historical sales data to identify trends and patterns.
Businesses operating in industries with strong seasonality or predictable sales cycles.
Organizations looking to predict sales over specific time frames, such as monthly, quarterly, or annually.
Scenarios requiring long-term forecasts that incorporate historical trends and cyclicality.
Time series analysis works best when external market conditions and sales strategies remain relatively stable, ensuring historical patterns are a reliable predictor of future sales.
How Time Series Analysis Works
Time series analysis involves decomposing historical data into components such as trend, seasonality, and residuals. Common techniques include trend analysis, moving averages, and autoregressive models like ARIMA (Autoregressive Integrated Moving Average).
Steps in Time Series Analysis for Sales Forecasting:
Collect Historical Data
Use historical sales data to create a time series. Ensure data is consistent and spans a meaningful period.Decompose the Data
Break the time series into its components:Trend: Long-term direction of sales performance.
Seasonality: Regular, repeating patterns (e.g., higher sales in holiday seasons).
Residuals: Irregular variations or noise.
Choose a Time Series Model
Select an appropriate model, such as Simple Moving Average (SMA), ARIMA, or Exponential Smoothing, depending on the data’s complexity and objectives.Generate Forecasts
Use the model to predict future sales based on historical patterns and trends.
To learn more about this method and explore real examples with formulas and numbers, download the eBook “13 Sales Forecasting Methods.
Monte Carlo Simulation
The Monte Carlo method is a powerful and advanced sales forecasting technique that uses probability and random sampling to predict future sales performance. Unlike traditional deterministic methods, this approach accounts for uncertainty, variability, and randomness in the sales process, offering a range of possible outcomes rather than a single point estimate. It’s especially useful for businesses operating in complex, dynamic markets or those with high variability in sales cycles and deal sizes.
When is the Monte Carlo Method Applicable?
The Monte Carlo simulation is particularly effective for:
Companies dealing with uncertain variables, such as fluctuating sales cycles, conversion rates, or market conditions.
Businesses managing a large number of deals with varying probabilities of closure.
Scenarios requiring risk analysis or probabilistic forecasting to account for inaccurate sales forecasting or unpredictable events.
Organizations with access to robust sales forecasting software and relevant data for simulations.
It is ideal for businesses seeking to assess a range of potential outcomes and their likelihoods rather than relying on a single forecast.
How the Monte Carlo Method Works
The Monte Carlo simulation generates thousands of scenarios based on input variables (e.g., deal size, probability of closure, sales cycle length) to create a probabilistic distribution of outcomes. This allows businesses to see the likelihood of different revenue levels within a specified timeframe.
Steps to Perform Monte Carlo Simulation for Sales Forecasting:
Define Key Variables
Identify the variables that influence future sales revenue, such as deal size, conversion rates, sales cycle length, and lead volume.Set Probability Distributions
Assign probability distributions (e.g., normal, uniform) to each variable based on historical sales data or market trends.Run Simulations
Use random sampling techniques to simulate thousands (or more) possible outcomes by varying the input variables.Analyze Results
Examine the distribution of outcomes to identify likely revenue ranges, confidence intervals, and risks.
Formula
While the Monte Carlo method does not rely on a single formula, the general process involves:
Simulated Outcome = f (Random Inputs from Defined Distributions)
Where f is the model that calculates sales revenue based on the chosen input variables.
Example
A business has three key variables influencing sales revenue:
Number of Deals: Normally distributed with a mean of 50 and a standard deviation of 5.
Average Deal Size: Uniformly distributed between $10,000 and $15,000.
Conversion Rate: Normally distributed with a mean of 20% and a standard deviation of 3%.
Using these distributions, the Monte Carlo simulation generates thousands of random samples for each variable, calculates the revenue for each scenario, and creates a revenue distribution.
Sample Calculation for One Simulation:
Deals: 52 (randomly selected from the distribution)
Deal Size: $12,000 (randomly selected)
Conversion Rate: 22% (randomly selected)
Revenue for this simulation:
Revenue = 52 x 12,000 x 0.22 = $137, 280
Repeating this process thousands of times produces a range of potential revenues, such as:
10th Percentile: $800,000
50th Percentile (Median): $1,100,000
90th Percentile: $1,400,000
This result allows the business to predict future sales revenue within a probabilistic framework and assess risks.
Limitations
Requires significant data and expertise to set up and run simulations effectively.
Can be computationally intensive without access to the right tools.
Results depend heavily on the accuracy of input variables and probability distributions.
Not suitable for businesses with limited or poor-quality historical sales data.
Qualitative Sales Forecasting
Qualitative sales forecasting is a forecasting method that relies on subjective opinions and insights from sales reps, frontline teams, or industry experts rather than purely on historical sales data. This approach involves gathering individual perspectives to predict future sales performance, making it particularly useful in scenarios where quantitative data may be limited or unavailable.
When is Qualitative Sales Forecasting Applicable?
This method is commonly used in the following situations:
Startups or businesses without sufficient historical revenue data.
Industries experiencing rapid changes, where past trends may not reliably predict the future.
Companies entering new markets or launching new products without established sales histories.
Organizations seeking to incorporate on-the-ground insights from sales reps or subject matter experts.
While qualitative sales forecasting can offer valuable insights, its reliance on intuition and subjective judgments means it is best paired with other methods to enhance forecasting accuracy.
How Qualitative Sales Forecasting Works
This approach involves gathering insights and opinions from knowledgeable individuals through methods such as:
Pipeline Reviews
Sales managers and sales reps review the current sales pipeline, assess deals, and offer opinions on the likelihood of closing deals based on their sales process understanding.Expert Panels
A group of experts, such as industry leaders or market analysts, provides projections on future sales based on their understanding of market conditions, market trends, and competitive dynamics.Team Input
Frontline teams provide real-time feedback and intuitive insights during regular meetings, contributing to a more nuanced understanding of potential sales outcomes.Scenario Analysis
Opinions are gathered to simulate possible future scenarios, such as changes in sales strategies or shifts in customer behavior, to anticipate their impact on future sales revenue.
Key Features of Qualitative Sales Forecasting
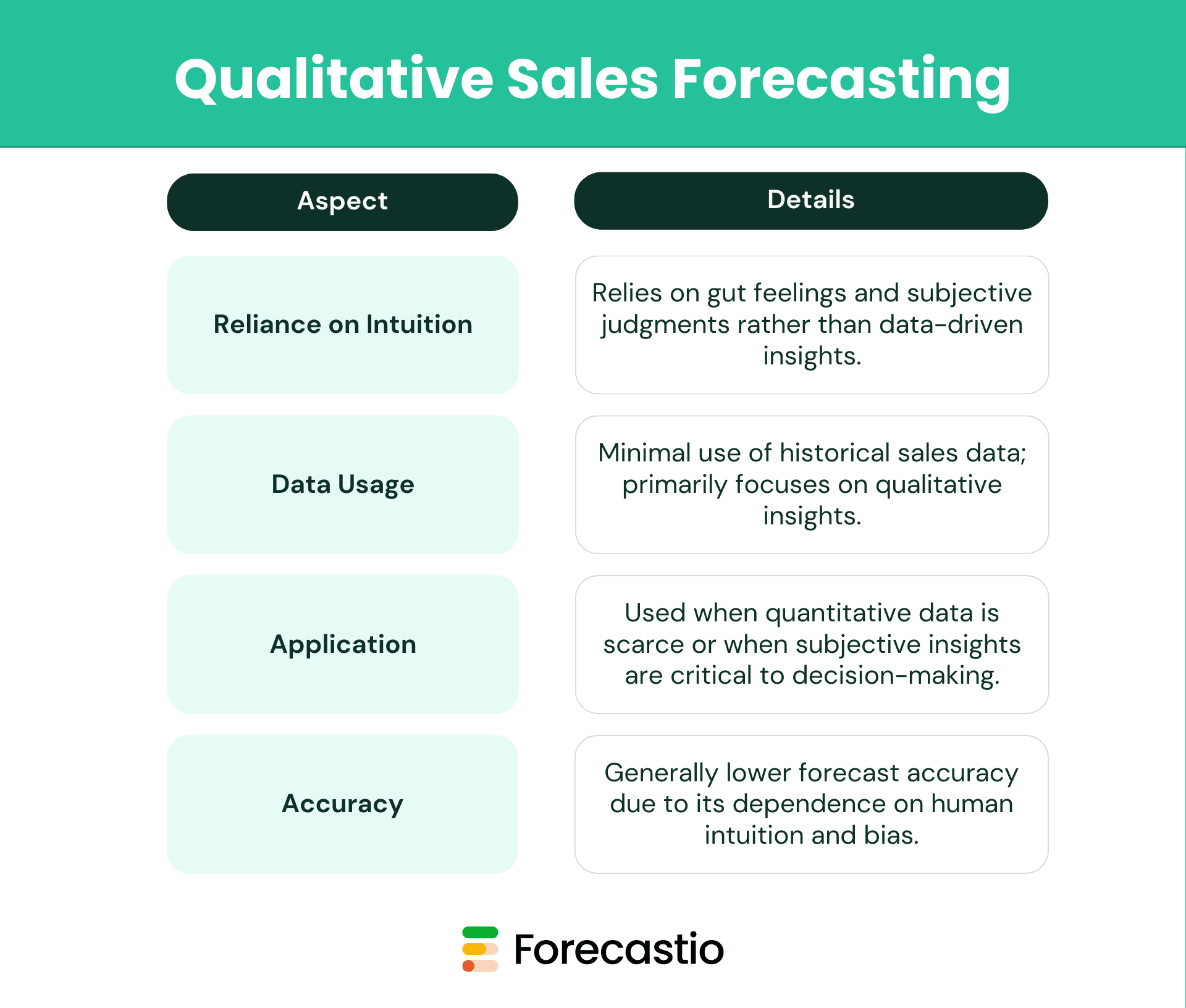
Example
Scenario: A company launching a new product in an unfamiliar market has no prior historical sales data. During a team meeting, sales reps provide opinions on the likelihood of closing deals in the current pipeline, while an expert panel forecasts sales growth based on anticipated customer demand and market trends.
Forecast: Based on this combined input, the company estimates potential revenue of $500,000 for the quarter, understanding that this is a subjective estimate that may require adjustments as more data becomes available.
Limitations
Highly subjective and prone to bias, reducing sales forecasting accuracy.
Relies heavily on the expertise and intuition of individuals, which may vary.
Lacks the robustness of data-driven methods, making it less suitable for precise sales projections.
Limited scalability in large organizations with complex sales cycles.
To learn more about other sales forecasting methods and explore real examples, download the eBook “13 Sales Forecasting Methods.
Most Common Mistakes and Pitfalls
Accurate sales forecasting is critical for predicting future sales, allocating resources, and achieving business growth. However, many companies face challenges that lead to inaccurate forecasts. Let’s explore the most common reasons behind failures in sales forecasting and how to overcome them using appropriate sales forecasting methods and tools.
1. Data Limitations
A lack of historical sales data is a common issue for startups and newly established businesses. Without a sales history, creating accurate sales forecasts can be challenging.
To address this:
Prioritize defining the data you need to collect and implement systems to gather it from the outset.
Employ qualitative sales forecasting or methods like pipeline-based forecasting, which rely on current sales pipeline insights rather than historical data. These techniques can provide valuable projections even with limited data.
2. Data Inaccuracy
Poor data quality is a leading cause of inaccurate sales forecasting. Ensuring data accuracy should be a top priority for any company aiming to improve forecast accuracy.
Common causes of data inaccuracy include:
Human error during manual data entry by sales reps.
Outdated or incomplete information in CRM systems.
Solutions:
Schedule regular data clean-up initiatives.
Use CRM rules and triggers to validate data.
Automate data enrichment processes, especially for customer information.
Ensure your CRM integrates seamlessly with other data sources.
Foster a culture of data accuracy within your team and offer incentives for maintaining clean data.
3. Irrelevant or Outdated Data
Even accurate data can lead to inaccurate forecasts if it is irrelevant or outdated. This issue is common among companies relying on spreadsheets for sales forecasting, as these tools often lack real-time updates and integration with CRM systems.
The solution:
Implement sales forecasting software that leverages real-time CRM data.
Replace outdated spreadsheet systems with tools that provide comprehensive and up-to-date sales forecasting models.
4. Lack of Stakeholder Buy-In
For sales forecasting to succeed, it must be ingrained in the company culture. Without the support of senior management and other stakeholders, forecasting accuracy can suffer.
Common challenges include:
Lack of prioritization of forecasting by leadership.
Perception that forecasting is unimportant for small businesses.
How to address this:
Emphasize the importance of sales forecasting accuracy for business survival and growth.
Demonstrate the value of accurate forecasts using techniques suited to the company’s size and stage.
5. Undefined Forecasting Process
Sales forecasting is an ongoing process, not a one-time task. Companies without a clear process often face challenges in achieving reliable sales projections.
Key components of a defined forecasting process:
Establish data entry standards for sales reps.
Schedule regular forecasting reviews and team meetings.
Deploy appropriate tools, such as sales forecasting software.
Continuously refine forecasting models based on feedback and outcomes.
6. Inappropriate Forecasting Methods
Choosing the wrong sales forecasting method is a common pitfall. Not all methods suit every business or situation, and selecting an inappropriate model can lead to inaccurate sales forecasting.
Examples of mismatches:
Time series forecasting requires sufficient historical data and is unsuitable for companies lacking this.
Sales cycle length forecasting doesn’t work effectively if the cycle is too short to yield meaningful insights.
Opportunity stage forecasting fails if your sales process lacks structure or sufficient data to calculate probabilities.
The solution:
Select the right sales forecasting method that aligns with your current data, process maturity, and goals.
7. Failure to Employ Forecasting Software
Many companies still rely on spreadsheets for sales forecasting, a practice that is both time-consuming and prone to errors. Spreadsheets lack the capabilities to implement advanced sales forecasting models effectively.
Challenges with spreadsheets:
Manual updates are labor-intensive and error-prone.
Limited support for complex forecasting techniques, such as Monte Carlo simulations or multivariable analysis forecasting.
Why use forecasting software:
Modern tools integrate seamlessly with CRM systems, enabling real-time data updates.
They support advanced methods like opportunity stage forecasting, historical forecasting, and lead-driven forecasting.
These tools are increasingly affordable and scalable for businesses of all sizes.
Recommendation:
Invest in sales forecasting software that aligns with your needs, offers robust capabilities, and requires minimal setup or implementation complexity.
Final Words on Sales Forecasting
Sales forecasting has been a cornerstone of my work since I first entered the B2B sales space. I am a strong advocate for the power of sales forecasting, believing it is essential for businesses striving to achieve predictable growth and improve sales performance.
No matter the size of the organization or the sales model it employs, I firmly believe that every company should prioritize sales forecasting. By dedicating time to refining their sales forecasting process and implementing strategies to enhance forecast accuracy, businesses can pave the way for sustainable and efficient growth.
Investing in accurate sales forecasts is not just about predicting revenue—it’s about making informed decisions that drive success.
To learn more about other sales forecasting methods and explore real examples, download the eBook “13 Sales Forecasting Methods.
Introduction
Sales forecasting has become a cornerstone of modern sales strategy, frequently appearing in countless articles, LinkedIn posts, and influencer blogs. Despite its widespread discussion among industry experts and the wealth of resources available, many organizations still struggle to achieve accurate sales forecasts. According to the Gartner State of Sales Operation Survey, over 50% of sales leaders express a lack of confidence in their sales forecasting accuracy—a gap that often leads to misaligned goals and missed opportunities.
From my own experience, sales forecasting has always been a challenging yet essential task. The ability to predict future sales not only drives strategic planning but also ensures better resource allocation, budget planning, and revenue forecasting. However, mastering sales forecasting requires more than just intuition—it demands time, effort, internal buy-in, and the right sales forecasting software.
In this article, we’ll explore why achieving accurate sales forecasting is so critical for B2B organizations. We’ll examine the best sales forecasting methods available, such as regression analysis, historical sales data forecasting, and qualitative forecasting, while also addressing the common pitfalls that sales leaders, sales reps, and RevOps teams encounter during the sales forecasting process. By understanding these challenges and leveraging historical sales data effectively, organizations can improve sales forecasting accuracy and drive better business outcomes.
The definition of sales forecasting
Sales forecasting is the practice of predicting future sales by analyzing various data points, such as historical sales data, current market trends, sales pipeline performance, and broader economic indicators. This forecasting process is vital for businesses to anticipate future revenue, enabling strategic planning, resource allocation, and improved sales performance. Accurate sales forecasting helps businesses stay ahead of market fluctuations and ensures they make informed decisions.
The responsibility for creating an accurate sales forecast often depends on the company's size, organizational structure, and stage of development. In startups and small businesses, the VP of Sales or Head of Sales typically oversees the sales forecasting process. These sales leaders rely on their intimate understanding of the sales pipeline and past sales data to create intuitive forecasts. This hands-on approach allows smaller teams to adapt quickly to changing conditions and provides flexibility during the early stages of growth.
As companies expand, their forecasting needs grow more complex. At this stage, sales forecasting is often handed over to dedicated teams such as Sales Operations or Revenue Operations (RevOps). These teams leverage advanced sales forecasting models, like regression analysis and multivariable analysis forecasting, to improve sales forecasting accuracy. They gather relevant data from historical sales figures, current market trends, and opportunity stage forecasting to align sales projections with the company’s broader strategic goals. The formation of such teams reflects a company’s evolution toward a data-driven forecasting process, ensuring accurate forecasts that drive strategic planning.
For larger organizations, sales forecasting becomes even more critical. With higher annual revenues, bigger budgets, and diverse sales teams, forecasting accuracy plays a pivotal role in maintaining operational efficiency and meeting stakeholder expectations. In these scenarios, relying on the right sales forecasting methods—such as time series analysis, AI forecasting, and qualitative forecasting —becomes essential. Inaccurate sales forecasting can lead to resource mismanagement, missed sales opportunities, and decreased revenue, underlining the importance of improving sales forecasting accuracy.
In summary, sales forecasting is a dynamic function that evolves with a company’s growth. While initially managed by sales leaders in smaller businesses, it transitions to specialized teams as organizations scale. Whether you're using sales forecasting software or leveraging qualitative sales forecasting techniques, understanding this progression is key to predicting future sales accurately and supporting long-term business success.
The difference between sales forecasting and sales planning
Sales forecasting and sales planning are two fundamental processes in any sales organization. While they are closely related, they serve distinct purposes and operate within different time frames. Together, they form a comprehensive approach to predict future sales and drive business success. Understanding the differences between these two concepts is crucial for sales leaders, sales managers, and RevOps teams aiming to improve sales performance and align strategic goals.
Sales Forecasting vs. Sales Planning: A Detailed Comparison
Sales forecasting focuses on predicting future sales revenue by analyzing historical sales data, market trends, and the sales pipeline. This process is best suited for short-term predictions, such as monthly or quarterly sales projections. Accurate forecasts help businesses allocate resources, improve sales forecasting accuracy, and adapt quickly to real-time changes in the market.
On the other hand, sales planning takes a broader, long-term perspective. It involves setting strategic sales targets, estimating the resources needed to achieve those goals, and preparing for various scenarios that could impact future revenue. Sales planning often includes what-if analyses to simulate potential market changes, shifts in sales performance, or other influencing factors.
Here’s a side-by-side comparison to highlight the key differences:
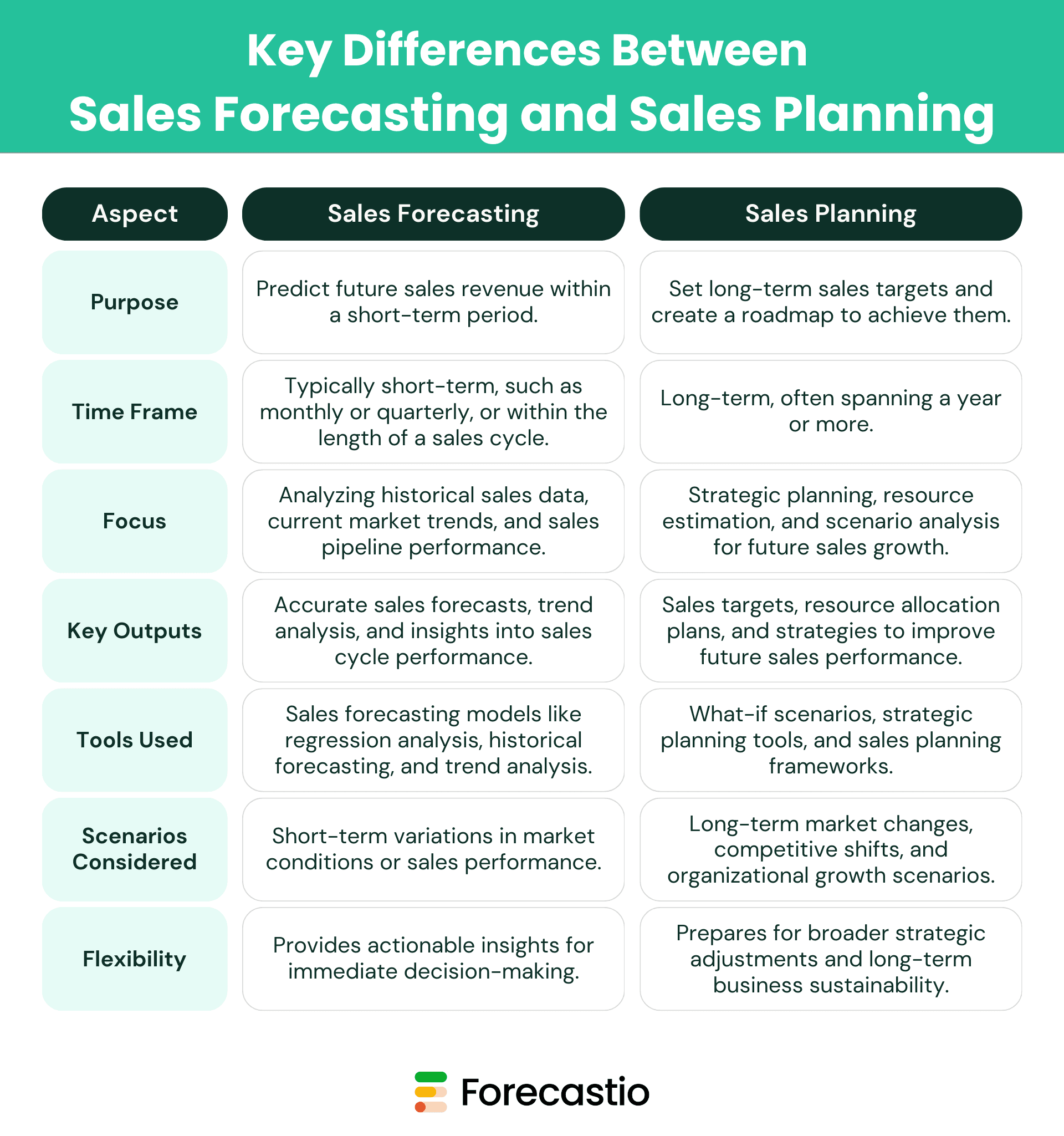
When to Use Sales Forecasting vs. Sales Planning
Sales forecasting is ideal for organizations that need to monitor sales performance closely and adjust their strategies in real time. It helps sales teams predict future sales within manageable time frames, ensuring efficient resource allocation and accurate forecasts.
Sales planning, on the other hand, is critical for companies aiming to set ambitious long-term goals and align their teams around a cohesive strategy. By considering potential market trends and future sales conditions, sales planning provides a robust framework for sustained growth and success.
Conclusion
While sales forecasting and sales planning are complementary processes, understanding their differences allows sales leaders, RevOps teams, and sales managers to use them effectively. Combining short-term forecasting accuracy with long-term strategic planning ensures businesses can navigate immediate challenges while staying focused on achieving their future sales targets.
By integrating accurate sales forecasting and comprehensive sales planning into their sales process, organizations can improve sales forecasting accuracy, anticipate future revenue, and drive long-term business success.
The importance of sales forecasting
"Accurately Predicting Sales is Impossible"—Is it Really?
Have you ever heard statements like, "Accurately predicting sales is impossible in this fast-changing market" or "As a startup, we’re too small to worry about forecasting; our priority is acquiring new accounts"? You’re not alone. Many businesses, especially startups, underestimate the vital role of accurate sales forecasting in ensuring long-term success.
To understand the significance of an accurate sales forecast, let’s explore the consequences of poor sales forecasting and its impact on business operations and growth:
1. Inefficient Resource Allocation
Inaccurate sales forecasts can lead to mismanagement of resources. Companies often overspend on marketing campaigns, hiring, sales tools, or product innovations, which drives up costs without improving efficiency. This misallocation not only reduces profitability but also increases cash burn—especially critical for startups operating on tight budgets.
2. Investor Confidence and Fundraising Challenges
Poor sales forecasting is often seen as a reflection of weak understanding of market trends, sales data, and revenue potential. Investors depend on reliable sales forecasting models to assess growth opportunities and risks. Overly optimistic or inconsistent sales projections can erode trust, damaging the company’s credibility and hindering its ability to raise funds.
3. Limited Investments in Growth and Innovation
Consistently missing sales projections creates unpredictability and reduces confidence in future forecasts. This uncertainty often forces businesses to prioritize managing cash risks over growth initiatives, such as scaling teams, investing in innovation, or experimenting with new sales strategies. Accurate forecasting is essential to balance these priorities effectively.
4. Leadership Credibility and Team Morale
Whether created by a Sales Leader, RevOps team, or Sales Operations department, inaccurate sales forecasting undermines leadership credibility. Missed revenue targets can highlight deficiencies in forecasting processes, historical sales data, tools, or team alignment. This not only casts doubt on leadership effectiveness but also negatively impacts team morale and sales performance.
Recognizing the importance of accurate sales forecasting is critical for business sustainability and growth. Leveraging the right sales forecasting methods can significantly improve sales forecasting accuracy.
Now, let’s delve into the best forecasting methods and models used by B2B sales organizations to predict future sales, align resources, and achieve reliable sales projections.
Sales forecasting methods
Sales forecasting is a critical aspect of strategic planning and revenue management, but there’s no universal solution. The 'one size fits all' approach simply doesn’t apply to sales forecasting methods.
Every company is unique, with its own set of influencing factors, such as:
The amount of historical sales data
The maturity of the sales process
The sales model (e.g., subscription-based, enterprise sales, or transactional sales)
Market dynamics and trends
The specific industry
These factors must be carefully considered when selecting the right sales forecasting method. Choosing a method that aligns with your business's structure and objectives can significantly improve sales forecasting accuracy and drive actionable insights.
Top-Down Sales Forecasting
Top-down sales forecasting begins with the big picture, analyzing overall market conditions, industry trends, and historical revenue data to predict future sales. This approach involves:
Assessing key market indicators, such as demand fluctuations and competitive dynamics.
Analyzing broad trends to estimate the total addressable market (TAM) and the company’s potential market share.
Using historical sales data and performance trends to refine projections.
This method works well for companies seeking to align sales strategies with macroeconomic conditions or when little granular data is available from sales reps. However, its effectiveness relies heavily on accurate trend analysis and a deep understanding of the market.
Bottom-Up Forecasting
In contrast, bottom-up forecasting starts at the individual level. This approach focuses on aggregating forecasts from sales reps, territories, or verticals to create a comprehensive forecast for the entire business. Key elements include:
Collecting individual sales projections during one-on-one meetings with sales reps.
Leveraging sales pipeline data and opportunity stage forecasting to assess potential revenue.
Summing up forecasts across teams, customer segments, or regions to provide a holistic sales projection.
Bottom-up forecasting benefits from the deep knowledge of sales reps, who are often closest to the customer and pipeline activity. This method is particularly effective for companies with a well-established sales process and a strong understanding of historical sales performance at the micro level.
Historical Sales Data Forecasting
Historical sales data forecasting is one of the most straightforward and widely used sales forecasting methods. It relies on analyzing past sales data to identify trends, seasonality, and patterns that can be used to predict future sales. This method is especially effective for companies with reliable and consistent historical revenue data and works well when external conditions, such as the market and industry trends, remain relatively stable.
When is Historical Sales Data Forecasting Applicable?
This method is best suited for:
Businesses with several years of historical sales data.
Stable industries with predictable sales cycles and minimal market disruption.
Companies looking for a straightforward approach to generate accurate sales forecasts without relying on complex models.
Scenarios where identifying seasonal trends or long-term patterns is necessary for resource planning, inventory management, and target setting.
However, it may not be ideal for businesses experiencing rapid changes in their sales process, market dynamics, or customer behavior, as it assumes past trends will continue into the future.
How Historical Forecasting Works
At its core, historical forecasting involves analyzing past sales performance to estimate future outcomes. Common techniques include the Simple Moving Average (SMA) approach.
Formula:
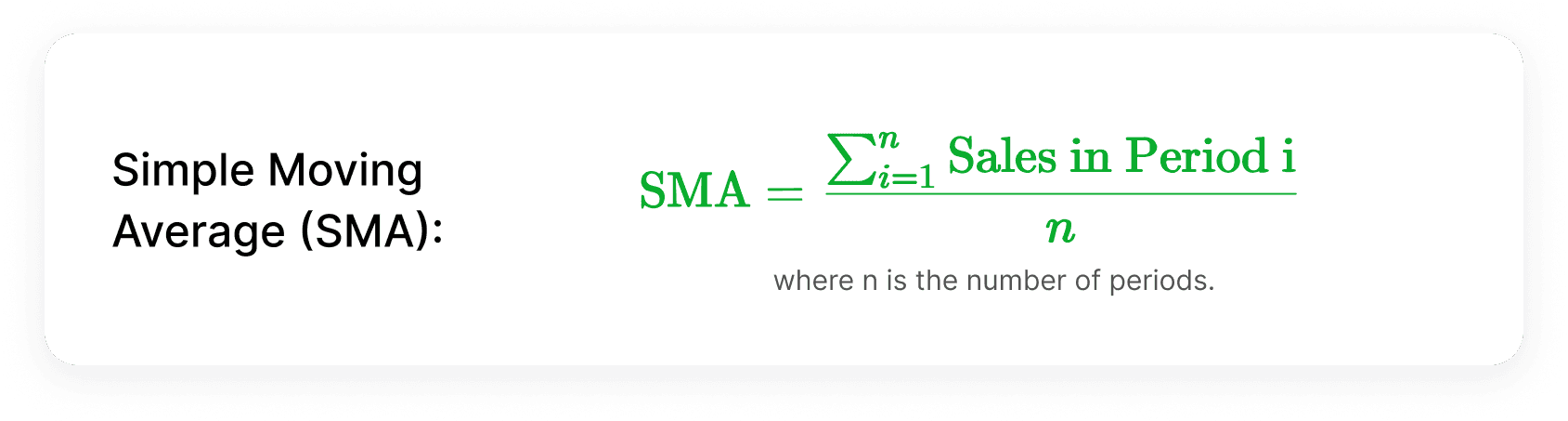
Where:
n = Number of periods used in the calculation.
Sales values are taken from historical sales data.
Example
A company wants to forecast sales for April using the sales data from January, February, and March:
January sales = $10,000
February sales = $12,000
March sales = $11,000
Using the SMA formula:
SMA for April = (10,000 + 12,000 + 11,000) / 3 = 11,000
The forecasted sales for April are $11,000
Opportunity Stage Forecasting
Opportunity stage forecasting is a sales forecasting method that evaluates deals in the sales pipeline based on their stage within the sales cycle. By assigning probabilities to each stage, businesses can estimate the likelihood of closing deals and generate more accurate sales forecasts. This method is particularly effective for companies with a well-defined sales process and a structured sales pipeline.
When is Opportunity Stage Forecasting Applicable?
This method works well for:
Companies with a mature sales process and clearly defined pipeline stages.
Businesses looking for a deal-by-deal approach to forecast future sales revenue.
Sales teams that rely on consistent sales rep input and CRM systems to track opportunities.
Scenarios where management wants to assess pipeline health and prioritize high-probability deals.
Opportunity stage forecasting is most applicable in environments with complex sales cycles, where deals progress through multiple stages before closing.
How Opportunity Stage Forecasting Works
Steps to Perform Opportunity Stage Forecasting
Define Sales Pipeline Stages
Clearly outline stages in the sales process, such as "Prospecting," "Discovery," "Proposal Sent," and "Negotiation."
Assign Probabilities to Each Stage
Assign a probability of closing for each stage based on historical data. For example:
Prospecting: 10%
Discovery: 30%
Proposal Sent: 60%
Negotiation: 80%
Closed-Won: 100%
Calculate Weighted Revenue for Each Opportunity
Multiply the deal value by the probability associated with its current stage.
Sum the Weighted Revenues
Add up all weighted revenues to forecast total pipeline revenue.
Example
A company has the following opportunities in its sales pipeline:
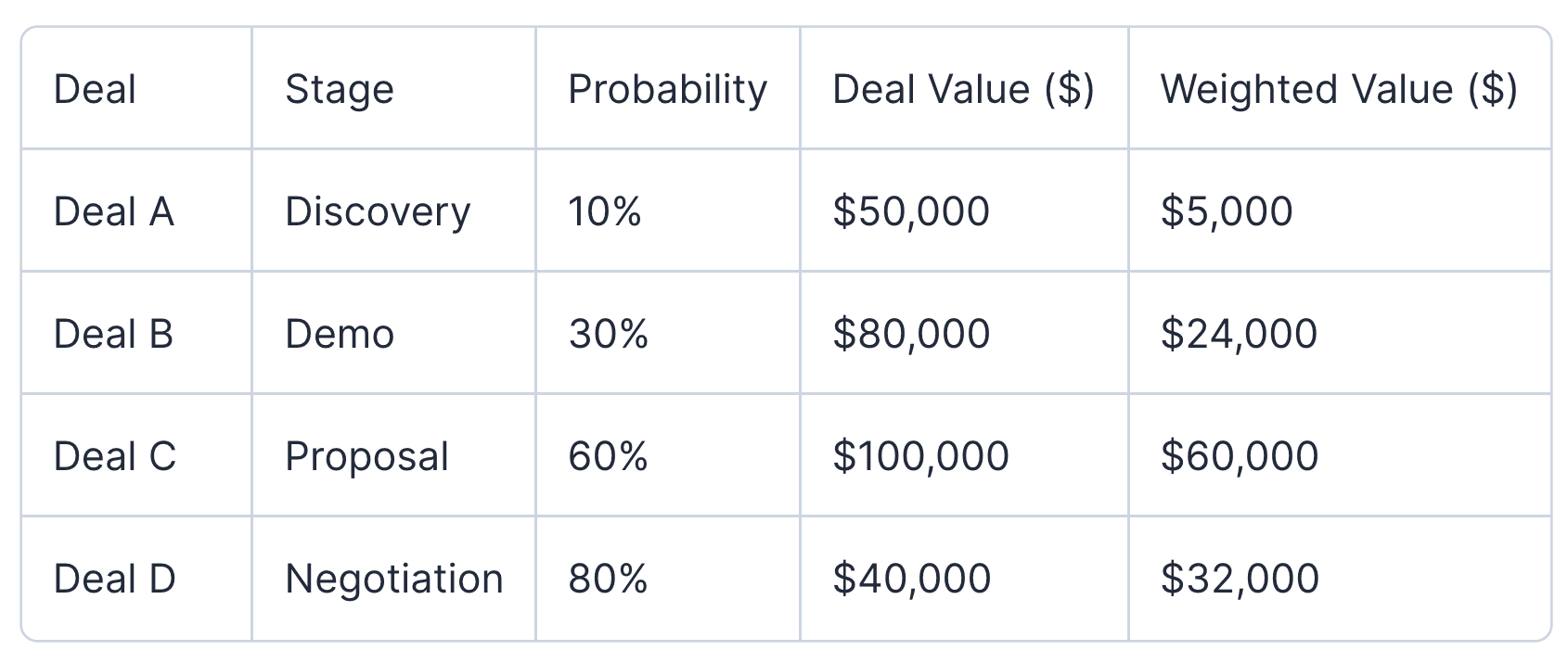
Total Forecasted Revenue = $5,000 + $24,000 + $60,000 + $32,000 = $121,000
Length of Sales Cycle Forecasting
Length of sales cycle forecasting is a method that predicts future sales revenue by analyzing the average time it takes for deals to progress through the sales cycle and close. By understanding the typical duration of a sales cycle, businesses can estimate when current opportunities are likely to close and generate accurate sales forecasts.
When is Length of Sales Cycle Forecasting Applicable?
This method is particularly effective for:
Companies with consistent and well-documented sales cycles.
Businesses tracking the time deals spend in each pipeline stage.
Organizations with repetitive or recurring sales processes, such as B2B subscription models.
Sales teams seek to predict revenue timing based on pipeline activity and deal velocity.
This approach works best in environments where the length of the sales cycle is stable and not influenced by external variables, such as market disruptions or significant changes in sales strategies.
How Length of Sales Cycle Forecasting Works
This method calculates the average duration of the sales cycle from initial contact to deal closure and applies this duration to current opportunities to estimate when revenue will be realized.
Steps to Perform Length of Sales Cycle Forecasting:
Analyze Historical Sales Data. Use historical revenue data to determine the average sales cycle length for past deals.
Segment Deals by Similar Characteristics. Group deals by size, industry, customer segment, or other relevant factors to identify patterns in the sales cycle.
Apply the Average Cycle Length to Current Opportunities. For each deal in the pipeline, estimate the closing date based on its entry date and the average cycle length.
Calculate Forecasted Revenue by Period. Sum the values of deals expected to close within a specific time frame (e.g., monthly or quarterly).
Limitations
Assumes sales cycle lengths remain consistent, which may not be true in dynamic markets.
Requires accurate tracking of deal entry dates and sales cycle duration.
Less effective for businesses with highly variable or undefined sales cycles.
To learn more about this method and explore real examples with numbers, download the eBook “13 Sales Forecasting Methods.
Lead-Driven Sales Forecasting
Lead-driven forecasting is a sales forecasting method that uses the volume of leads entering the sales pipeline and their historical conversion rates to estimate future sales revenue. This method ties sales projections directly to the performance of marketing and sales efforts, making it an essential tool for businesses with a high focus on lead generation and nurturing strategies.
When is Lead-Driven Forecasting Applicable?
Lead-driven forecasting is particularly effective for:
Companies with a predictable sales process and well-documented lead conversion rates.
Businesses that generate a high volume of leads through consistent sales strategies or marketing campaigns.
Organizations with a clear understanding of how lead quantity and quality impact sales performance.
Teams seek to predict future revenue based on the volume and behavior of leads entering the pipeline.
This method works best for companies with stable lead sources and consistent sales cycle durations.
How Lead-Driven Forecasting Works
This approach predicts future sales revenue by analyzing the following factors:
Lead Volume: The number of leads entering the pipeline within a specific period.
Conversion Rate: The historical percentage of leads that convert into closed deals.
Average Deal Value: The typical revenue generated from a single deal.
By multiplying these factors, businesses can estimate their total future sales revenue.
Formula:
Forecasted Revenue = Lead Volume X Conversion Rate X Average Deal Value
Limitations
Assumes consistent lead quality, which may not always be the case.
Relies on accurate tracking of leads and historical conversion data.
Less effective for companies with long or unpredictable sales cycles.
External factors, such as market changes or competitive dynamics, may impact actual sales outcomes.
To learn more about this method and explore real examples with numbers, download our free Sales Forecasting Guide.
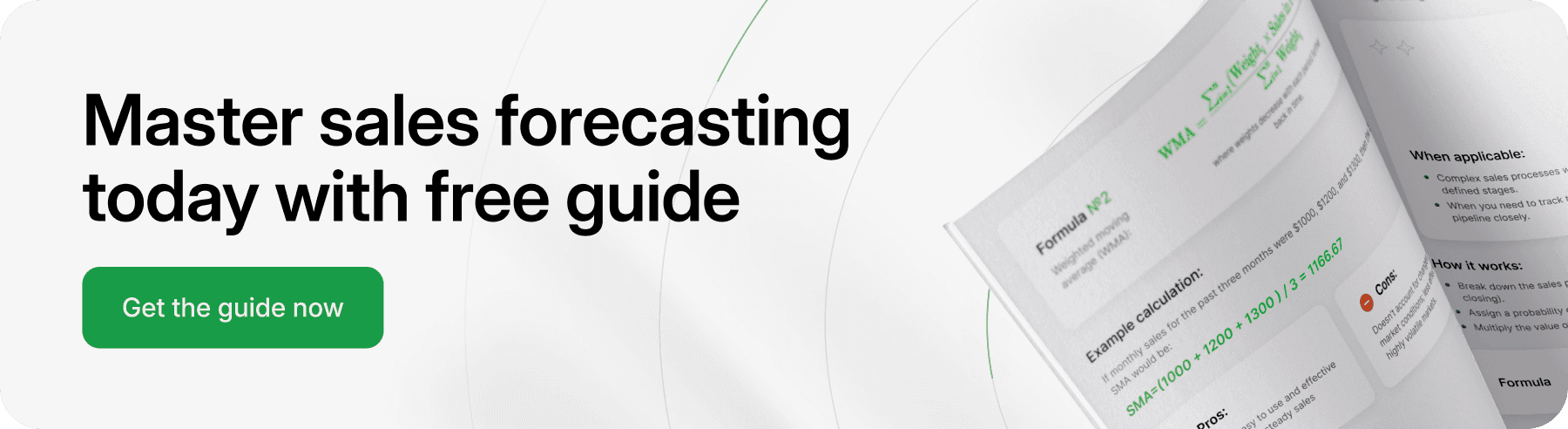
Forecasting based on deal probabilities
Forecasting based on deal probabilities is a method that predicts future sales revenue by assigning a probability of closure to each deal in the sales pipeline. This approach provides a granular, data-driven forecast that takes into account the likelihood of individual deals closing.
When is Forecasting Based on Deal Probabilities Applicable?
This method is particularly effective for:
Companies with a structured sales process and detailed deal tracking.
Businesses that manage a variety of deals with differing sizes, stages, and closing probabilities.
Teams looking to generate accurate sales forecasts by assessing the health of the sales pipeline at an individual deal level.
Organizations aiming to improve sales forecasting accuracy by incorporating real-time data into the forecasting process.
How Forecasting Based on Deal Probabilities Works
The method involves calculating the weighted value of each deal by multiplying its value by its probability of closing. These weighted values are then summed to create the total forecasted revenue.
Steps to Perform Deal Probability Forecasting:
Assign Probabilities to Each Deal. Determine the likelihood of each deal closing based on its stage in the sales cycle or other relevant factors.
Calculate Weighted Revenue. Multiply the deal value by its probability to determine its contribution to the forecast.
Sum the Weighted Values. Add up all weighted values to calculate the total forecasted revenue.
Example
A company has the following deals in its sales pipeline:
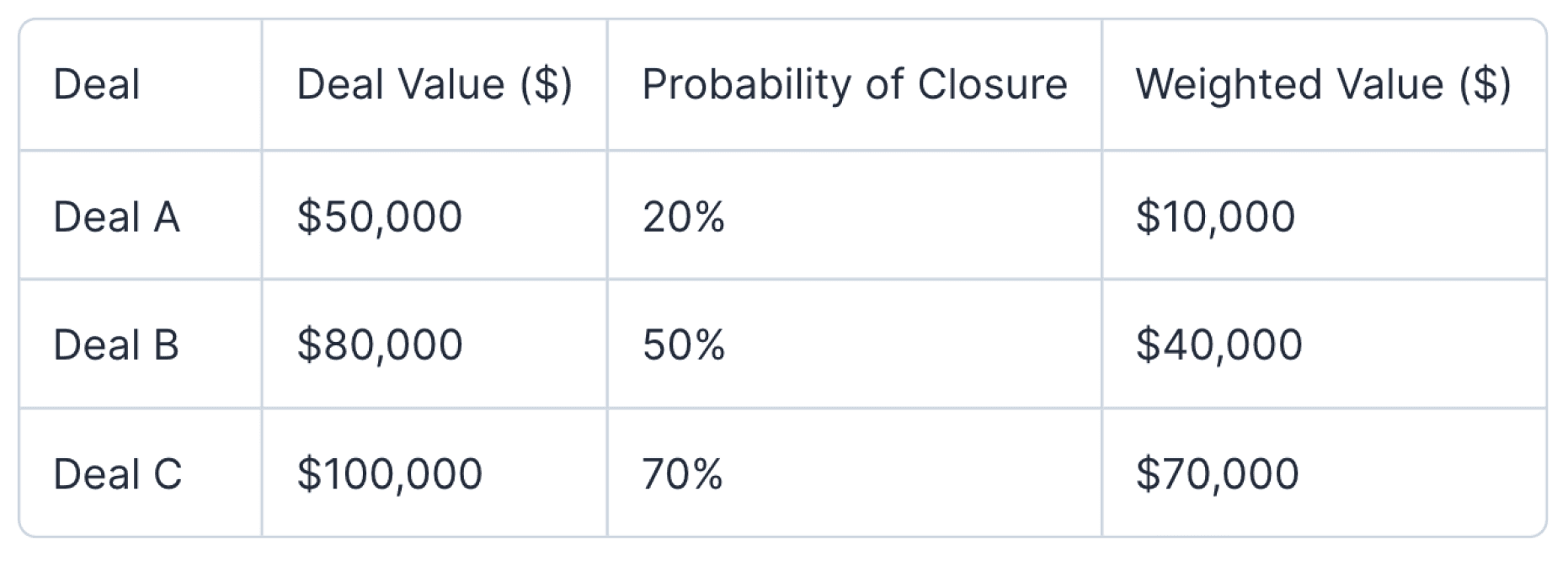
Forecasted Revenue = $10,000 + $40,000 + $70,000 = $120,000
Time Series Analysis
Time series analysis is a sales forecasting method that uses historical sales data to identify patterns, trends, and seasonality over time. By analyzing these recurring patterns, businesses can make data-driven predictions about future sales performance. This method is particularly effective for organizations with consistent sales data over an extended period and is widely used to create accurate forecasts.
When is Time Series Analysis Applicable?
This method is ideal for:
Companies with sufficient historical sales data to identify trends and patterns.
Businesses operating in industries with strong seasonality or predictable sales cycles.
Organizations looking to predict sales over specific time frames, such as monthly, quarterly, or annually.
Scenarios requiring long-term forecasts that incorporate historical trends and cyclicality.
Time series analysis works best when external market conditions and sales strategies remain relatively stable, ensuring historical patterns are a reliable predictor of future sales.
How Time Series Analysis Works
Time series analysis involves decomposing historical data into components such as trend, seasonality, and residuals. Common techniques include trend analysis, moving averages, and autoregressive models like ARIMA (Autoregressive Integrated Moving Average).
Steps in Time Series Analysis for Sales Forecasting:
Collect Historical Data
Use historical sales data to create a time series. Ensure data is consistent and spans a meaningful period.Decompose the Data
Break the time series into its components:Trend: Long-term direction of sales performance.
Seasonality: Regular, repeating patterns (e.g., higher sales in holiday seasons).
Residuals: Irregular variations or noise.
Choose a Time Series Model
Select an appropriate model, such as Simple Moving Average (SMA), ARIMA, or Exponential Smoothing, depending on the data’s complexity and objectives.Generate Forecasts
Use the model to predict future sales based on historical patterns and trends.
To learn more about this method and explore real examples with formulas and numbers, download the eBook “13 Sales Forecasting Methods.
Monte Carlo Simulation
The Monte Carlo method is a powerful and advanced sales forecasting technique that uses probability and random sampling to predict future sales performance. Unlike traditional deterministic methods, this approach accounts for uncertainty, variability, and randomness in the sales process, offering a range of possible outcomes rather than a single point estimate. It’s especially useful for businesses operating in complex, dynamic markets or those with high variability in sales cycles and deal sizes.
When is the Monte Carlo Method Applicable?
The Monte Carlo simulation is particularly effective for:
Companies dealing with uncertain variables, such as fluctuating sales cycles, conversion rates, or market conditions.
Businesses managing a large number of deals with varying probabilities of closure.
Scenarios requiring risk analysis or probabilistic forecasting to account for inaccurate sales forecasting or unpredictable events.
Organizations with access to robust sales forecasting software and relevant data for simulations.
It is ideal for businesses seeking to assess a range of potential outcomes and their likelihoods rather than relying on a single forecast.
How the Monte Carlo Method Works
The Monte Carlo simulation generates thousands of scenarios based on input variables (e.g., deal size, probability of closure, sales cycle length) to create a probabilistic distribution of outcomes. This allows businesses to see the likelihood of different revenue levels within a specified timeframe.
Steps to Perform Monte Carlo Simulation for Sales Forecasting:
Define Key Variables
Identify the variables that influence future sales revenue, such as deal size, conversion rates, sales cycle length, and lead volume.Set Probability Distributions
Assign probability distributions (e.g., normal, uniform) to each variable based on historical sales data or market trends.Run Simulations
Use random sampling techniques to simulate thousands (or more) possible outcomes by varying the input variables.Analyze Results
Examine the distribution of outcomes to identify likely revenue ranges, confidence intervals, and risks.
Formula
While the Monte Carlo method does not rely on a single formula, the general process involves:
Simulated Outcome = f (Random Inputs from Defined Distributions)
Where f is the model that calculates sales revenue based on the chosen input variables.
Example
A business has three key variables influencing sales revenue:
Number of Deals: Normally distributed with a mean of 50 and a standard deviation of 5.
Average Deal Size: Uniformly distributed between $10,000 and $15,000.
Conversion Rate: Normally distributed with a mean of 20% and a standard deviation of 3%.
Using these distributions, the Monte Carlo simulation generates thousands of random samples for each variable, calculates the revenue for each scenario, and creates a revenue distribution.
Sample Calculation for One Simulation:
Deals: 52 (randomly selected from the distribution)
Deal Size: $12,000 (randomly selected)
Conversion Rate: 22% (randomly selected)
Revenue for this simulation:
Revenue = 52 x 12,000 x 0.22 = $137, 280
Repeating this process thousands of times produces a range of potential revenues, such as:
10th Percentile: $800,000
50th Percentile (Median): $1,100,000
90th Percentile: $1,400,000
This result allows the business to predict future sales revenue within a probabilistic framework and assess risks.
Limitations
Requires significant data and expertise to set up and run simulations effectively.
Can be computationally intensive without access to the right tools.
Results depend heavily on the accuracy of input variables and probability distributions.
Not suitable for businesses with limited or poor-quality historical sales data.
Qualitative Sales Forecasting
Qualitative sales forecasting is a forecasting method that relies on subjective opinions and insights from sales reps, frontline teams, or industry experts rather than purely on historical sales data. This approach involves gathering individual perspectives to predict future sales performance, making it particularly useful in scenarios where quantitative data may be limited or unavailable.
When is Qualitative Sales Forecasting Applicable?
This method is commonly used in the following situations:
Startups or businesses without sufficient historical revenue data.
Industries experiencing rapid changes, where past trends may not reliably predict the future.
Companies entering new markets or launching new products without established sales histories.
Organizations seeking to incorporate on-the-ground insights from sales reps or subject matter experts.
While qualitative sales forecasting can offer valuable insights, its reliance on intuition and subjective judgments means it is best paired with other methods to enhance forecasting accuracy.
How Qualitative Sales Forecasting Works
This approach involves gathering insights and opinions from knowledgeable individuals through methods such as:
Pipeline Reviews
Sales managers and sales reps review the current sales pipeline, assess deals, and offer opinions on the likelihood of closing deals based on their sales process understanding.Expert Panels
A group of experts, such as industry leaders or market analysts, provides projections on future sales based on their understanding of market conditions, market trends, and competitive dynamics.Team Input
Frontline teams provide real-time feedback and intuitive insights during regular meetings, contributing to a more nuanced understanding of potential sales outcomes.Scenario Analysis
Opinions are gathered to simulate possible future scenarios, such as changes in sales strategies or shifts in customer behavior, to anticipate their impact on future sales revenue.
Key Features of Qualitative Sales Forecasting
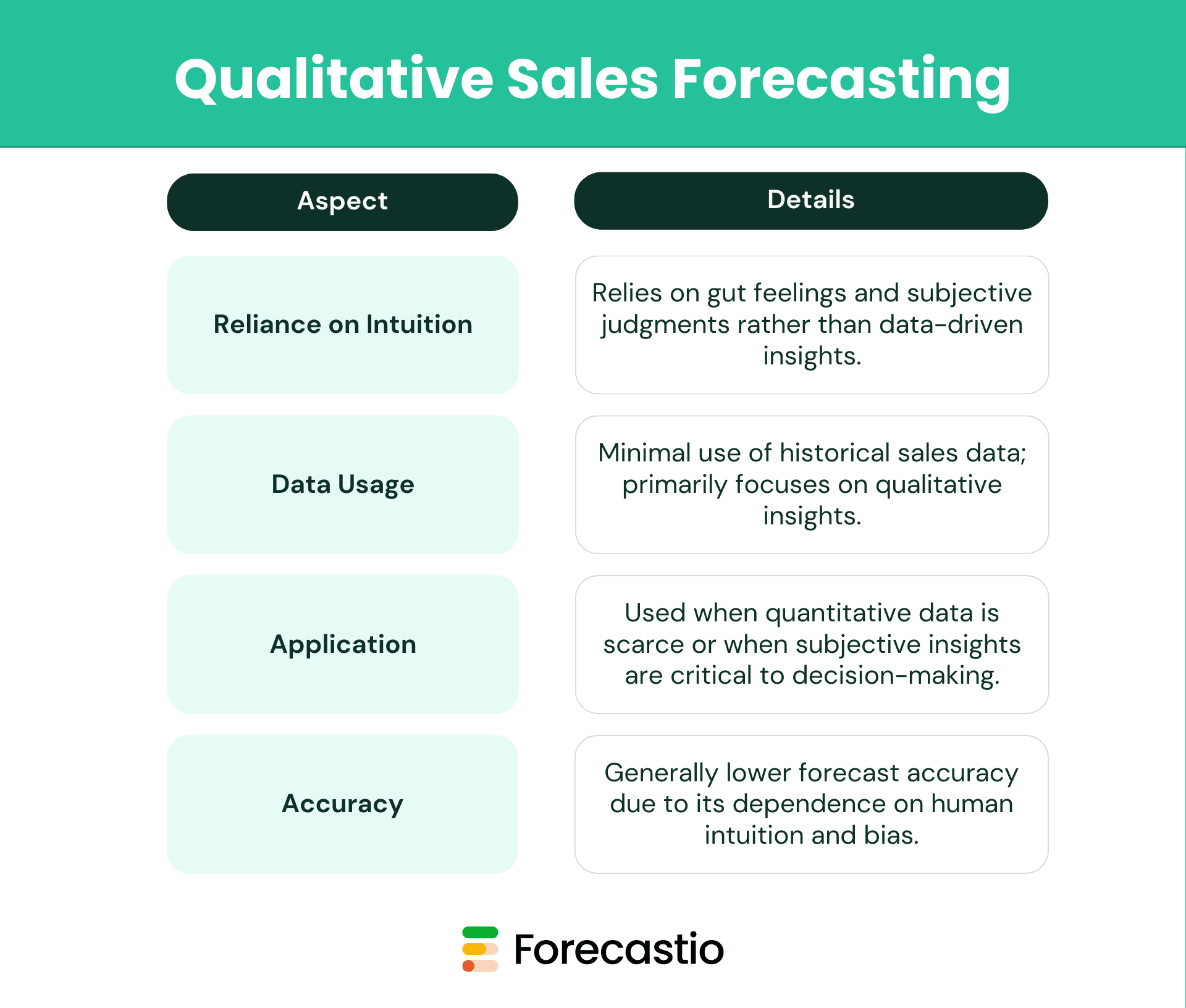
Example
Scenario: A company launching a new product in an unfamiliar market has no prior historical sales data. During a team meeting, sales reps provide opinions on the likelihood of closing deals in the current pipeline, while an expert panel forecasts sales growth based on anticipated customer demand and market trends.
Forecast: Based on this combined input, the company estimates potential revenue of $500,000 for the quarter, understanding that this is a subjective estimate that may require adjustments as more data becomes available.
Limitations
Highly subjective and prone to bias, reducing sales forecasting accuracy.
Relies heavily on the expertise and intuition of individuals, which may vary.
Lacks the robustness of data-driven methods, making it less suitable for precise sales projections.
Limited scalability in large organizations with complex sales cycles.
To learn more about other sales forecasting methods and explore real examples, download the eBook “13 Sales Forecasting Methods.
Most Common Mistakes and Pitfalls
Accurate sales forecasting is critical for predicting future sales, allocating resources, and achieving business growth. However, many companies face challenges that lead to inaccurate forecasts. Let’s explore the most common reasons behind failures in sales forecasting and how to overcome them using appropriate sales forecasting methods and tools.
1. Data Limitations
A lack of historical sales data is a common issue for startups and newly established businesses. Without a sales history, creating accurate sales forecasts can be challenging.
To address this:
Prioritize defining the data you need to collect and implement systems to gather it from the outset.
Employ qualitative sales forecasting or methods like pipeline-based forecasting, which rely on current sales pipeline insights rather than historical data. These techniques can provide valuable projections even with limited data.
2. Data Inaccuracy
Poor data quality is a leading cause of inaccurate sales forecasting. Ensuring data accuracy should be a top priority for any company aiming to improve forecast accuracy.
Common causes of data inaccuracy include:
Human error during manual data entry by sales reps.
Outdated or incomplete information in CRM systems.
Solutions:
Schedule regular data clean-up initiatives.
Use CRM rules and triggers to validate data.
Automate data enrichment processes, especially for customer information.
Ensure your CRM integrates seamlessly with other data sources.
Foster a culture of data accuracy within your team and offer incentives for maintaining clean data.
3. Irrelevant or Outdated Data
Even accurate data can lead to inaccurate forecasts if it is irrelevant or outdated. This issue is common among companies relying on spreadsheets for sales forecasting, as these tools often lack real-time updates and integration with CRM systems.
The solution:
Implement sales forecasting software that leverages real-time CRM data.
Replace outdated spreadsheet systems with tools that provide comprehensive and up-to-date sales forecasting models.
4. Lack of Stakeholder Buy-In
For sales forecasting to succeed, it must be ingrained in the company culture. Without the support of senior management and other stakeholders, forecasting accuracy can suffer.
Common challenges include:
Lack of prioritization of forecasting by leadership.
Perception that forecasting is unimportant for small businesses.
How to address this:
Emphasize the importance of sales forecasting accuracy for business survival and growth.
Demonstrate the value of accurate forecasts using techniques suited to the company’s size and stage.
5. Undefined Forecasting Process
Sales forecasting is an ongoing process, not a one-time task. Companies without a clear process often face challenges in achieving reliable sales projections.
Key components of a defined forecasting process:
Establish data entry standards for sales reps.
Schedule regular forecasting reviews and team meetings.
Deploy appropriate tools, such as sales forecasting software.
Continuously refine forecasting models based on feedback and outcomes.
6. Inappropriate Forecasting Methods
Choosing the wrong sales forecasting method is a common pitfall. Not all methods suit every business or situation, and selecting an inappropriate model can lead to inaccurate sales forecasting.
Examples of mismatches:
Time series forecasting requires sufficient historical data and is unsuitable for companies lacking this.
Sales cycle length forecasting doesn’t work effectively if the cycle is too short to yield meaningful insights.
Opportunity stage forecasting fails if your sales process lacks structure or sufficient data to calculate probabilities.
The solution:
Select the right sales forecasting method that aligns with your current data, process maturity, and goals.
7. Failure to Employ Forecasting Software
Many companies still rely on spreadsheets for sales forecasting, a practice that is both time-consuming and prone to errors. Spreadsheets lack the capabilities to implement advanced sales forecasting models effectively.
Challenges with spreadsheets:
Manual updates are labor-intensive and error-prone.
Limited support for complex forecasting techniques, such as Monte Carlo simulations or multivariable analysis forecasting.
Why use forecasting software:
Modern tools integrate seamlessly with CRM systems, enabling real-time data updates.
They support advanced methods like opportunity stage forecasting, historical forecasting, and lead-driven forecasting.
These tools are increasingly affordable and scalable for businesses of all sizes.
Recommendation:
Invest in sales forecasting software that aligns with your needs, offers robust capabilities, and requires minimal setup or implementation complexity.
Final Words on Sales Forecasting
Sales forecasting has been a cornerstone of my work since I first entered the B2B sales space. I am a strong advocate for the power of sales forecasting, believing it is essential for businesses striving to achieve predictable growth and improve sales performance.
No matter the size of the organization or the sales model it employs, I firmly believe that every company should prioritize sales forecasting. By dedicating time to refining their sales forecasting process and implementing strategies to enhance forecast accuracy, businesses can pave the way for sustainable and efficient growth.
Investing in accurate sales forecasts is not just about predicting revenue—it’s about making informed decisions that drive success.
To learn more about other sales forecasting methods and explore real examples, download the eBook “13 Sales Forecasting Methods.
Introduction
Sales forecasting has become a cornerstone of modern sales strategy, frequently appearing in countless articles, LinkedIn posts, and influencer blogs. Despite its widespread discussion among industry experts and the wealth of resources available, many organizations still struggle to achieve accurate sales forecasts. According to the Gartner State of Sales Operation Survey, over 50% of sales leaders express a lack of confidence in their sales forecasting accuracy—a gap that often leads to misaligned goals and missed opportunities.
From my own experience, sales forecasting has always been a challenging yet essential task. The ability to predict future sales not only drives strategic planning but also ensures better resource allocation, budget planning, and revenue forecasting. However, mastering sales forecasting requires more than just intuition—it demands time, effort, internal buy-in, and the right sales forecasting software.
In this article, we’ll explore why achieving accurate sales forecasting is so critical for B2B organizations. We’ll examine the best sales forecasting methods available, such as regression analysis, historical sales data forecasting, and qualitative forecasting, while also addressing the common pitfalls that sales leaders, sales reps, and RevOps teams encounter during the sales forecasting process. By understanding these challenges and leveraging historical sales data effectively, organizations can improve sales forecasting accuracy and drive better business outcomes.
The definition of sales forecasting
Sales forecasting is the practice of predicting future sales by analyzing various data points, such as historical sales data, current market trends, sales pipeline performance, and broader economic indicators. This forecasting process is vital for businesses to anticipate future revenue, enabling strategic planning, resource allocation, and improved sales performance. Accurate sales forecasting helps businesses stay ahead of market fluctuations and ensures they make informed decisions.
The responsibility for creating an accurate sales forecast often depends on the company's size, organizational structure, and stage of development. In startups and small businesses, the VP of Sales or Head of Sales typically oversees the sales forecasting process. These sales leaders rely on their intimate understanding of the sales pipeline and past sales data to create intuitive forecasts. This hands-on approach allows smaller teams to adapt quickly to changing conditions and provides flexibility during the early stages of growth.
As companies expand, their forecasting needs grow more complex. At this stage, sales forecasting is often handed over to dedicated teams such as Sales Operations or Revenue Operations (RevOps). These teams leverage advanced sales forecasting models, like regression analysis and multivariable analysis forecasting, to improve sales forecasting accuracy. They gather relevant data from historical sales figures, current market trends, and opportunity stage forecasting to align sales projections with the company’s broader strategic goals. The formation of such teams reflects a company’s evolution toward a data-driven forecasting process, ensuring accurate forecasts that drive strategic planning.
For larger organizations, sales forecasting becomes even more critical. With higher annual revenues, bigger budgets, and diverse sales teams, forecasting accuracy plays a pivotal role in maintaining operational efficiency and meeting stakeholder expectations. In these scenarios, relying on the right sales forecasting methods—such as time series analysis, AI forecasting, and qualitative forecasting —becomes essential. Inaccurate sales forecasting can lead to resource mismanagement, missed sales opportunities, and decreased revenue, underlining the importance of improving sales forecasting accuracy.
In summary, sales forecasting is a dynamic function that evolves with a company’s growth. While initially managed by sales leaders in smaller businesses, it transitions to specialized teams as organizations scale. Whether you're using sales forecasting software or leveraging qualitative sales forecasting techniques, understanding this progression is key to predicting future sales accurately and supporting long-term business success.
The difference between sales forecasting and sales planning
Sales forecasting and sales planning are two fundamental processes in any sales organization. While they are closely related, they serve distinct purposes and operate within different time frames. Together, they form a comprehensive approach to predict future sales and drive business success. Understanding the differences between these two concepts is crucial for sales leaders, sales managers, and RevOps teams aiming to improve sales performance and align strategic goals.
Sales Forecasting vs. Sales Planning: A Detailed Comparison
Sales forecasting focuses on predicting future sales revenue by analyzing historical sales data, market trends, and the sales pipeline. This process is best suited for short-term predictions, such as monthly or quarterly sales projections. Accurate forecasts help businesses allocate resources, improve sales forecasting accuracy, and adapt quickly to real-time changes in the market.
On the other hand, sales planning takes a broader, long-term perspective. It involves setting strategic sales targets, estimating the resources needed to achieve those goals, and preparing for various scenarios that could impact future revenue. Sales planning often includes what-if analyses to simulate potential market changes, shifts in sales performance, or other influencing factors.
Here’s a side-by-side comparison to highlight the key differences:
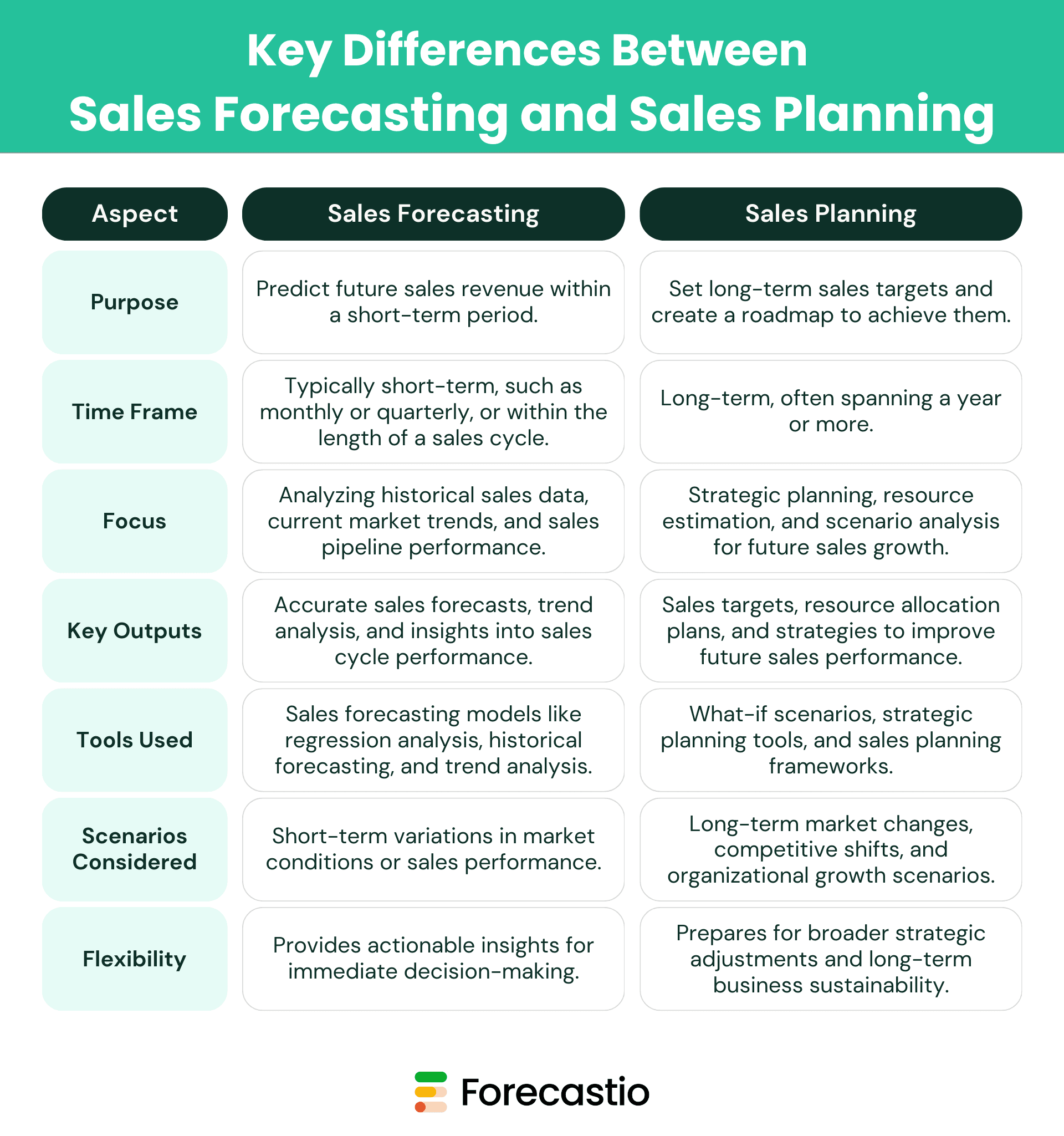
When to Use Sales Forecasting vs. Sales Planning
Sales forecasting is ideal for organizations that need to monitor sales performance closely and adjust their strategies in real time. It helps sales teams predict future sales within manageable time frames, ensuring efficient resource allocation and accurate forecasts.
Sales planning, on the other hand, is critical for companies aiming to set ambitious long-term goals and align their teams around a cohesive strategy. By considering potential market trends and future sales conditions, sales planning provides a robust framework for sustained growth and success.
Conclusion
While sales forecasting and sales planning are complementary processes, understanding their differences allows sales leaders, RevOps teams, and sales managers to use them effectively. Combining short-term forecasting accuracy with long-term strategic planning ensures businesses can navigate immediate challenges while staying focused on achieving their future sales targets.
By integrating accurate sales forecasting and comprehensive sales planning into their sales process, organizations can improve sales forecasting accuracy, anticipate future revenue, and drive long-term business success.
The importance of sales forecasting
"Accurately Predicting Sales is Impossible"—Is it Really?
Have you ever heard statements like, "Accurately predicting sales is impossible in this fast-changing market" or "As a startup, we’re too small to worry about forecasting; our priority is acquiring new accounts"? You’re not alone. Many businesses, especially startups, underestimate the vital role of accurate sales forecasting in ensuring long-term success.
To understand the significance of an accurate sales forecast, let’s explore the consequences of poor sales forecasting and its impact on business operations and growth:
1. Inefficient Resource Allocation
Inaccurate sales forecasts can lead to mismanagement of resources. Companies often overspend on marketing campaigns, hiring, sales tools, or product innovations, which drives up costs without improving efficiency. This misallocation not only reduces profitability but also increases cash burn—especially critical for startups operating on tight budgets.
2. Investor Confidence and Fundraising Challenges
Poor sales forecasting is often seen as a reflection of weak understanding of market trends, sales data, and revenue potential. Investors depend on reliable sales forecasting models to assess growth opportunities and risks. Overly optimistic or inconsistent sales projections can erode trust, damaging the company’s credibility and hindering its ability to raise funds.
3. Limited Investments in Growth and Innovation
Consistently missing sales projections creates unpredictability and reduces confidence in future forecasts. This uncertainty often forces businesses to prioritize managing cash risks over growth initiatives, such as scaling teams, investing in innovation, or experimenting with new sales strategies. Accurate forecasting is essential to balance these priorities effectively.
4. Leadership Credibility and Team Morale
Whether created by a Sales Leader, RevOps team, or Sales Operations department, inaccurate sales forecasting undermines leadership credibility. Missed revenue targets can highlight deficiencies in forecasting processes, historical sales data, tools, or team alignment. This not only casts doubt on leadership effectiveness but also negatively impacts team morale and sales performance.
Recognizing the importance of accurate sales forecasting is critical for business sustainability and growth. Leveraging the right sales forecasting methods can significantly improve sales forecasting accuracy.
Now, let’s delve into the best forecasting methods and models used by B2B sales organizations to predict future sales, align resources, and achieve reliable sales projections.
Sales forecasting methods
Sales forecasting is a critical aspect of strategic planning and revenue management, but there’s no universal solution. The 'one size fits all' approach simply doesn’t apply to sales forecasting methods.
Every company is unique, with its own set of influencing factors, such as:
The amount of historical sales data
The maturity of the sales process
The sales model (e.g., subscription-based, enterprise sales, or transactional sales)
Market dynamics and trends
The specific industry
These factors must be carefully considered when selecting the right sales forecasting method. Choosing a method that aligns with your business's structure and objectives can significantly improve sales forecasting accuracy and drive actionable insights.
Top-Down Sales Forecasting
Top-down sales forecasting begins with the big picture, analyzing overall market conditions, industry trends, and historical revenue data to predict future sales. This approach involves:
Assessing key market indicators, such as demand fluctuations and competitive dynamics.
Analyzing broad trends to estimate the total addressable market (TAM) and the company’s potential market share.
Using historical sales data and performance trends to refine projections.
This method works well for companies seeking to align sales strategies with macroeconomic conditions or when little granular data is available from sales reps. However, its effectiveness relies heavily on accurate trend analysis and a deep understanding of the market.
Bottom-Up Forecasting
In contrast, bottom-up forecasting starts at the individual level. This approach focuses on aggregating forecasts from sales reps, territories, or verticals to create a comprehensive forecast for the entire business. Key elements include:
Collecting individual sales projections during one-on-one meetings with sales reps.
Leveraging sales pipeline data and opportunity stage forecasting to assess potential revenue.
Summing up forecasts across teams, customer segments, or regions to provide a holistic sales projection.
Bottom-up forecasting benefits from the deep knowledge of sales reps, who are often closest to the customer and pipeline activity. This method is particularly effective for companies with a well-established sales process and a strong understanding of historical sales performance at the micro level.
Historical Sales Data Forecasting
Historical sales data forecasting is one of the most straightforward and widely used sales forecasting methods. It relies on analyzing past sales data to identify trends, seasonality, and patterns that can be used to predict future sales. This method is especially effective for companies with reliable and consistent historical revenue data and works well when external conditions, such as the market and industry trends, remain relatively stable.
When is Historical Sales Data Forecasting Applicable?
This method is best suited for:
Businesses with several years of historical sales data.
Stable industries with predictable sales cycles and minimal market disruption.
Companies looking for a straightforward approach to generate accurate sales forecasts without relying on complex models.
Scenarios where identifying seasonal trends or long-term patterns is necessary for resource planning, inventory management, and target setting.
However, it may not be ideal for businesses experiencing rapid changes in their sales process, market dynamics, or customer behavior, as it assumes past trends will continue into the future.
How Historical Forecasting Works
At its core, historical forecasting involves analyzing past sales performance to estimate future outcomes. Common techniques include the Simple Moving Average (SMA) approach.
Formula:
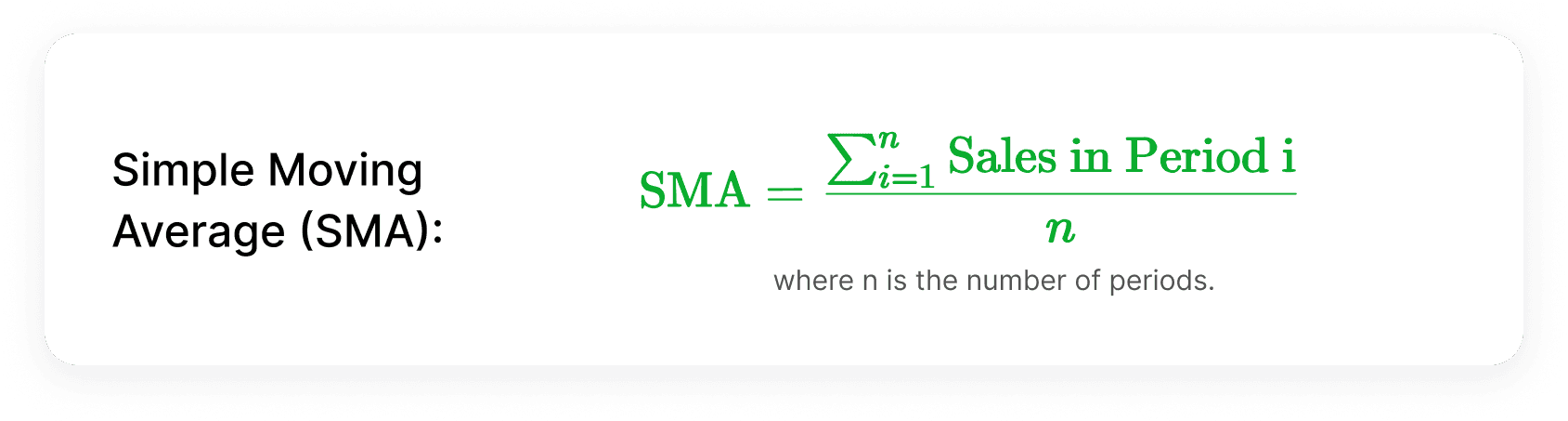
Where:
n = Number of periods used in the calculation.
Sales values are taken from historical sales data.
Example
A company wants to forecast sales for April using the sales data from January, February, and March:
January sales = $10,000
February sales = $12,000
March sales = $11,000
Using the SMA formula:
SMA for April = (10,000 + 12,000 + 11,000) / 3 = 11,000
The forecasted sales for April are $11,000
Opportunity Stage Forecasting
Opportunity stage forecasting is a sales forecasting method that evaluates deals in the sales pipeline based on their stage within the sales cycle. By assigning probabilities to each stage, businesses can estimate the likelihood of closing deals and generate more accurate sales forecasts. This method is particularly effective for companies with a well-defined sales process and a structured sales pipeline.
When is Opportunity Stage Forecasting Applicable?
This method works well for:
Companies with a mature sales process and clearly defined pipeline stages.
Businesses looking for a deal-by-deal approach to forecast future sales revenue.
Sales teams that rely on consistent sales rep input and CRM systems to track opportunities.
Scenarios where management wants to assess pipeline health and prioritize high-probability deals.
Opportunity stage forecasting is most applicable in environments with complex sales cycles, where deals progress through multiple stages before closing.
How Opportunity Stage Forecasting Works
Steps to Perform Opportunity Stage Forecasting
Define Sales Pipeline Stages
Clearly outline stages in the sales process, such as "Prospecting," "Discovery," "Proposal Sent," and "Negotiation."
Assign Probabilities to Each Stage
Assign a probability of closing for each stage based on historical data. For example:
Prospecting: 10%
Discovery: 30%
Proposal Sent: 60%
Negotiation: 80%
Closed-Won: 100%
Calculate Weighted Revenue for Each Opportunity
Multiply the deal value by the probability associated with its current stage.
Sum the Weighted Revenues
Add up all weighted revenues to forecast total pipeline revenue.
Example
A company has the following opportunities in its sales pipeline:
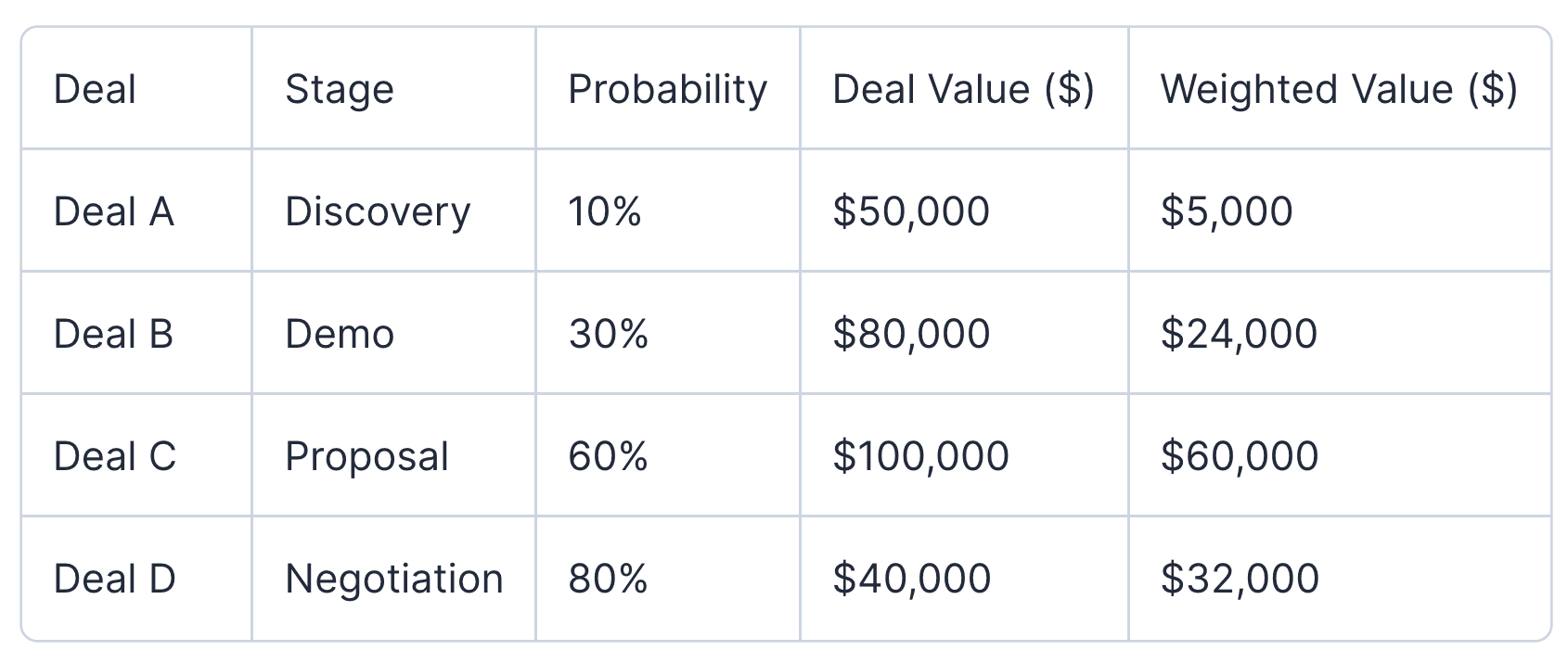
Total Forecasted Revenue = $5,000 + $24,000 + $60,000 + $32,000 = $121,000
Length of Sales Cycle Forecasting
Length of sales cycle forecasting is a method that predicts future sales revenue by analyzing the average time it takes for deals to progress through the sales cycle and close. By understanding the typical duration of a sales cycle, businesses can estimate when current opportunities are likely to close and generate accurate sales forecasts.
When is Length of Sales Cycle Forecasting Applicable?
This method is particularly effective for:
Companies with consistent and well-documented sales cycles.
Businesses tracking the time deals spend in each pipeline stage.
Organizations with repetitive or recurring sales processes, such as B2B subscription models.
Sales teams seek to predict revenue timing based on pipeline activity and deal velocity.
This approach works best in environments where the length of the sales cycle is stable and not influenced by external variables, such as market disruptions or significant changes in sales strategies.
How Length of Sales Cycle Forecasting Works
This method calculates the average duration of the sales cycle from initial contact to deal closure and applies this duration to current opportunities to estimate when revenue will be realized.
Steps to Perform Length of Sales Cycle Forecasting:
Analyze Historical Sales Data. Use historical revenue data to determine the average sales cycle length for past deals.
Segment Deals by Similar Characteristics. Group deals by size, industry, customer segment, or other relevant factors to identify patterns in the sales cycle.
Apply the Average Cycle Length to Current Opportunities. For each deal in the pipeline, estimate the closing date based on its entry date and the average cycle length.
Calculate Forecasted Revenue by Period. Sum the values of deals expected to close within a specific time frame (e.g., monthly or quarterly).
Limitations
Assumes sales cycle lengths remain consistent, which may not be true in dynamic markets.
Requires accurate tracking of deal entry dates and sales cycle duration.
Less effective for businesses with highly variable or undefined sales cycles.
To learn more about this method and explore real examples with numbers, download the eBook “13 Sales Forecasting Methods.
Lead-Driven Sales Forecasting
Lead-driven forecasting is a sales forecasting method that uses the volume of leads entering the sales pipeline and their historical conversion rates to estimate future sales revenue. This method ties sales projections directly to the performance of marketing and sales efforts, making it an essential tool for businesses with a high focus on lead generation and nurturing strategies.
When is Lead-Driven Forecasting Applicable?
Lead-driven forecasting is particularly effective for:
Companies with a predictable sales process and well-documented lead conversion rates.
Businesses that generate a high volume of leads through consistent sales strategies or marketing campaigns.
Organizations with a clear understanding of how lead quantity and quality impact sales performance.
Teams seek to predict future revenue based on the volume and behavior of leads entering the pipeline.
This method works best for companies with stable lead sources and consistent sales cycle durations.
How Lead-Driven Forecasting Works
This approach predicts future sales revenue by analyzing the following factors:
Lead Volume: The number of leads entering the pipeline within a specific period.
Conversion Rate: The historical percentage of leads that convert into closed deals.
Average Deal Value: The typical revenue generated from a single deal.
By multiplying these factors, businesses can estimate their total future sales revenue.
Formula:
Forecasted Revenue = Lead Volume X Conversion Rate X Average Deal Value
Limitations
Assumes consistent lead quality, which may not always be the case.
Relies on accurate tracking of leads and historical conversion data.
Less effective for companies with long or unpredictable sales cycles.
External factors, such as market changes or competitive dynamics, may impact actual sales outcomes.
To learn more about this method and explore real examples with numbers, download our free Sales Forecasting Guide.
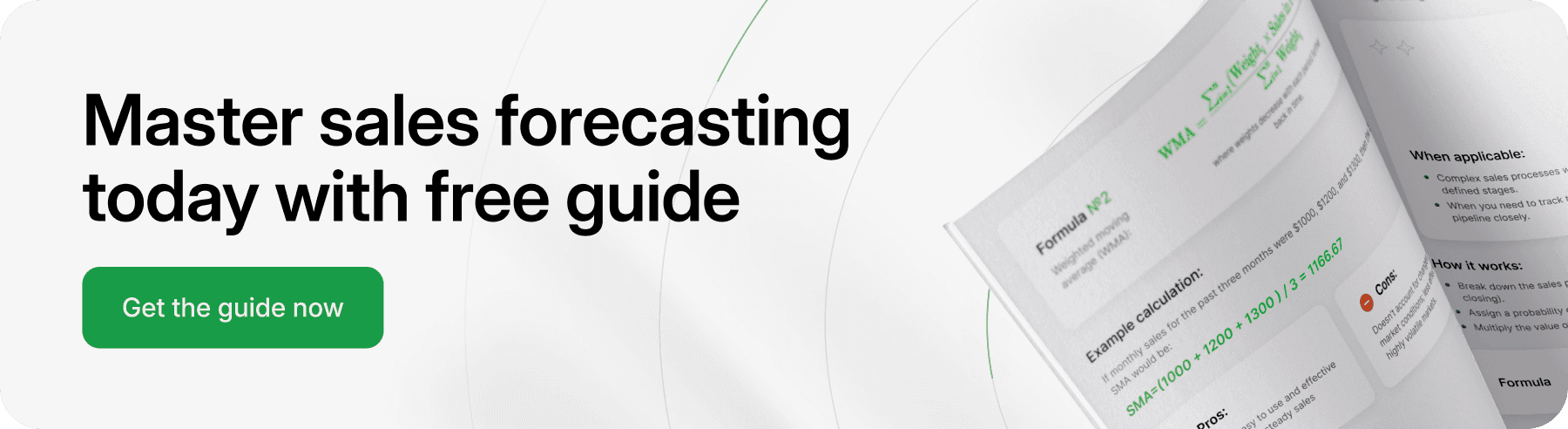
Forecasting based on deal probabilities
Forecasting based on deal probabilities is a method that predicts future sales revenue by assigning a probability of closure to each deal in the sales pipeline. This approach provides a granular, data-driven forecast that takes into account the likelihood of individual deals closing.
When is Forecasting Based on Deal Probabilities Applicable?
This method is particularly effective for:
Companies with a structured sales process and detailed deal tracking.
Businesses that manage a variety of deals with differing sizes, stages, and closing probabilities.
Teams looking to generate accurate sales forecasts by assessing the health of the sales pipeline at an individual deal level.
Organizations aiming to improve sales forecasting accuracy by incorporating real-time data into the forecasting process.
How Forecasting Based on Deal Probabilities Works
The method involves calculating the weighted value of each deal by multiplying its value by its probability of closing. These weighted values are then summed to create the total forecasted revenue.
Steps to Perform Deal Probability Forecasting:
Assign Probabilities to Each Deal. Determine the likelihood of each deal closing based on its stage in the sales cycle or other relevant factors.
Calculate Weighted Revenue. Multiply the deal value by its probability to determine its contribution to the forecast.
Sum the Weighted Values. Add up all weighted values to calculate the total forecasted revenue.
Example
A company has the following deals in its sales pipeline:
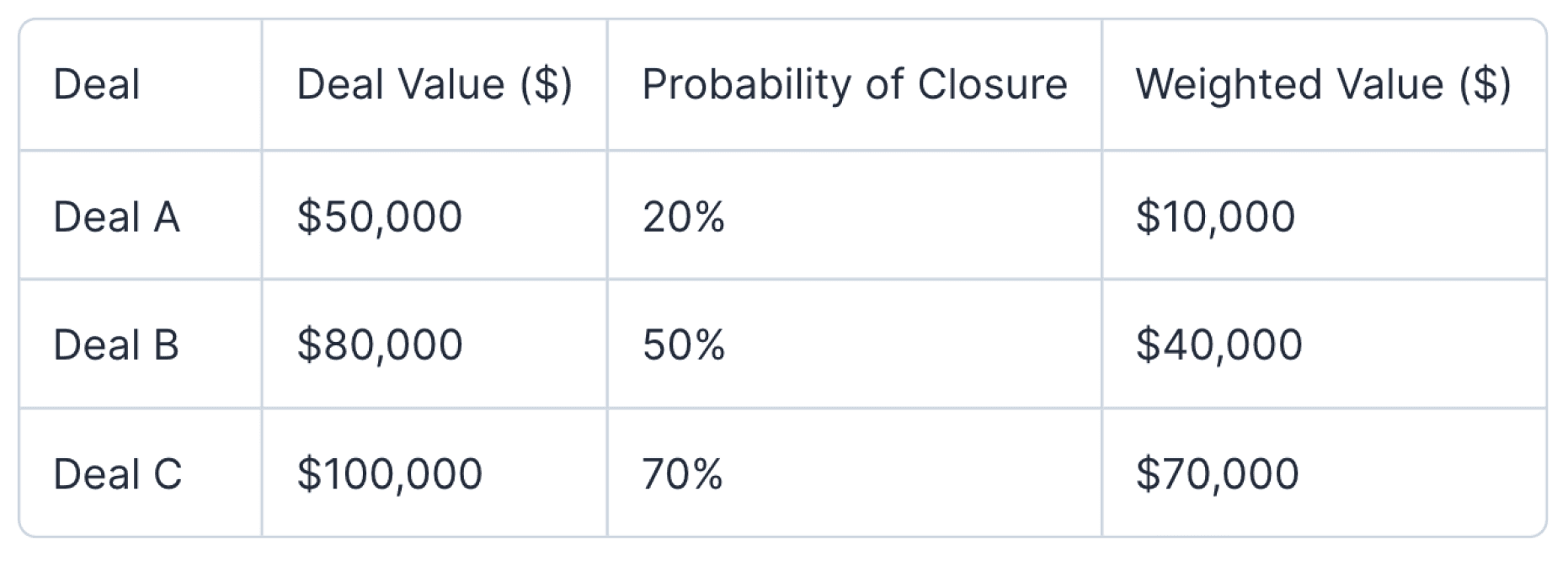
Forecasted Revenue = $10,000 + $40,000 + $70,000 = $120,000
Time Series Analysis
Time series analysis is a sales forecasting method that uses historical sales data to identify patterns, trends, and seasonality over time. By analyzing these recurring patterns, businesses can make data-driven predictions about future sales performance. This method is particularly effective for organizations with consistent sales data over an extended period and is widely used to create accurate forecasts.
When is Time Series Analysis Applicable?
This method is ideal for:
Companies with sufficient historical sales data to identify trends and patterns.
Businesses operating in industries with strong seasonality or predictable sales cycles.
Organizations looking to predict sales over specific time frames, such as monthly, quarterly, or annually.
Scenarios requiring long-term forecasts that incorporate historical trends and cyclicality.
Time series analysis works best when external market conditions and sales strategies remain relatively stable, ensuring historical patterns are a reliable predictor of future sales.
How Time Series Analysis Works
Time series analysis involves decomposing historical data into components such as trend, seasonality, and residuals. Common techniques include trend analysis, moving averages, and autoregressive models like ARIMA (Autoregressive Integrated Moving Average).
Steps in Time Series Analysis for Sales Forecasting:
Collect Historical Data
Use historical sales data to create a time series. Ensure data is consistent and spans a meaningful period.Decompose the Data
Break the time series into its components:Trend: Long-term direction of sales performance.
Seasonality: Regular, repeating patterns (e.g., higher sales in holiday seasons).
Residuals: Irregular variations or noise.
Choose a Time Series Model
Select an appropriate model, such as Simple Moving Average (SMA), ARIMA, or Exponential Smoothing, depending on the data’s complexity and objectives.Generate Forecasts
Use the model to predict future sales based on historical patterns and trends.
To learn more about this method and explore real examples with formulas and numbers, download the eBook “13 Sales Forecasting Methods.
Monte Carlo Simulation
The Monte Carlo method is a powerful and advanced sales forecasting technique that uses probability and random sampling to predict future sales performance. Unlike traditional deterministic methods, this approach accounts for uncertainty, variability, and randomness in the sales process, offering a range of possible outcomes rather than a single point estimate. It’s especially useful for businesses operating in complex, dynamic markets or those with high variability in sales cycles and deal sizes.
When is the Monte Carlo Method Applicable?
The Monte Carlo simulation is particularly effective for:
Companies dealing with uncertain variables, such as fluctuating sales cycles, conversion rates, or market conditions.
Businesses managing a large number of deals with varying probabilities of closure.
Scenarios requiring risk analysis or probabilistic forecasting to account for inaccurate sales forecasting or unpredictable events.
Organizations with access to robust sales forecasting software and relevant data for simulations.
It is ideal for businesses seeking to assess a range of potential outcomes and their likelihoods rather than relying on a single forecast.
How the Monte Carlo Method Works
The Monte Carlo simulation generates thousands of scenarios based on input variables (e.g., deal size, probability of closure, sales cycle length) to create a probabilistic distribution of outcomes. This allows businesses to see the likelihood of different revenue levels within a specified timeframe.
Steps to Perform Monte Carlo Simulation for Sales Forecasting:
Define Key Variables
Identify the variables that influence future sales revenue, such as deal size, conversion rates, sales cycle length, and lead volume.Set Probability Distributions
Assign probability distributions (e.g., normal, uniform) to each variable based on historical sales data or market trends.Run Simulations
Use random sampling techniques to simulate thousands (or more) possible outcomes by varying the input variables.Analyze Results
Examine the distribution of outcomes to identify likely revenue ranges, confidence intervals, and risks.
Formula
While the Monte Carlo method does not rely on a single formula, the general process involves:
Simulated Outcome = f (Random Inputs from Defined Distributions)
Where f is the model that calculates sales revenue based on the chosen input variables.
Example
A business has three key variables influencing sales revenue:
Number of Deals: Normally distributed with a mean of 50 and a standard deviation of 5.
Average Deal Size: Uniformly distributed between $10,000 and $15,000.
Conversion Rate: Normally distributed with a mean of 20% and a standard deviation of 3%.
Using these distributions, the Monte Carlo simulation generates thousands of random samples for each variable, calculates the revenue for each scenario, and creates a revenue distribution.
Sample Calculation for One Simulation:
Deals: 52 (randomly selected from the distribution)
Deal Size: $12,000 (randomly selected)
Conversion Rate: 22% (randomly selected)
Revenue for this simulation:
Revenue = 52 x 12,000 x 0.22 = $137, 280
Repeating this process thousands of times produces a range of potential revenues, such as:
10th Percentile: $800,000
50th Percentile (Median): $1,100,000
90th Percentile: $1,400,000
This result allows the business to predict future sales revenue within a probabilistic framework and assess risks.
Limitations
Requires significant data and expertise to set up and run simulations effectively.
Can be computationally intensive without access to the right tools.
Results depend heavily on the accuracy of input variables and probability distributions.
Not suitable for businesses with limited or poor-quality historical sales data.
Qualitative Sales Forecasting
Qualitative sales forecasting is a forecasting method that relies on subjective opinions and insights from sales reps, frontline teams, or industry experts rather than purely on historical sales data. This approach involves gathering individual perspectives to predict future sales performance, making it particularly useful in scenarios where quantitative data may be limited or unavailable.
When is Qualitative Sales Forecasting Applicable?
This method is commonly used in the following situations:
Startups or businesses without sufficient historical revenue data.
Industries experiencing rapid changes, where past trends may not reliably predict the future.
Companies entering new markets or launching new products without established sales histories.
Organizations seeking to incorporate on-the-ground insights from sales reps or subject matter experts.
While qualitative sales forecasting can offer valuable insights, its reliance on intuition and subjective judgments means it is best paired with other methods to enhance forecasting accuracy.
How Qualitative Sales Forecasting Works
This approach involves gathering insights and opinions from knowledgeable individuals through methods such as:
Pipeline Reviews
Sales managers and sales reps review the current sales pipeline, assess deals, and offer opinions on the likelihood of closing deals based on their sales process understanding.Expert Panels
A group of experts, such as industry leaders or market analysts, provides projections on future sales based on their understanding of market conditions, market trends, and competitive dynamics.Team Input
Frontline teams provide real-time feedback and intuitive insights during regular meetings, contributing to a more nuanced understanding of potential sales outcomes.Scenario Analysis
Opinions are gathered to simulate possible future scenarios, such as changes in sales strategies or shifts in customer behavior, to anticipate their impact on future sales revenue.
Key Features of Qualitative Sales Forecasting
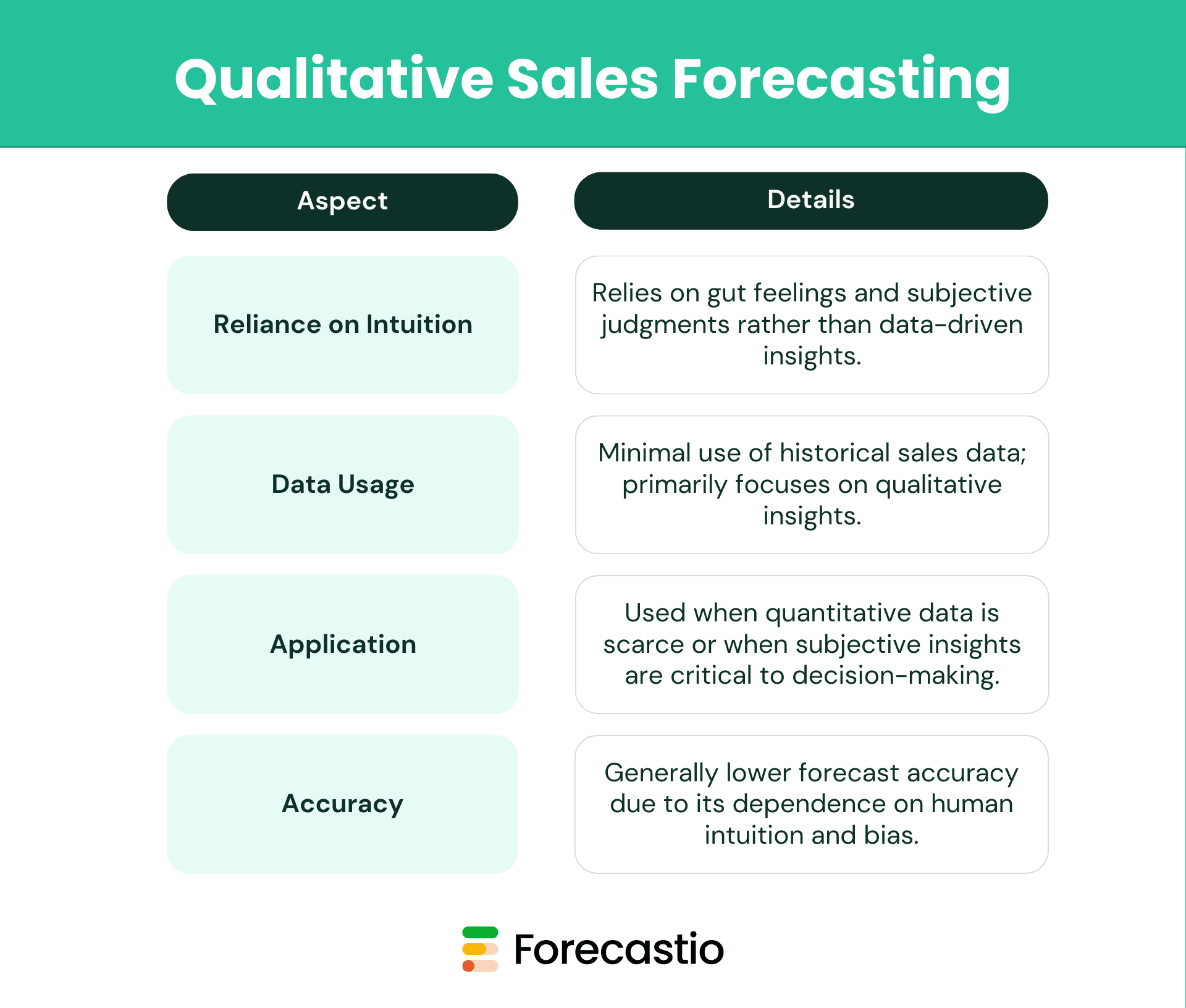
Example
Scenario: A company launching a new product in an unfamiliar market has no prior historical sales data. During a team meeting, sales reps provide opinions on the likelihood of closing deals in the current pipeline, while an expert panel forecasts sales growth based on anticipated customer demand and market trends.
Forecast: Based on this combined input, the company estimates potential revenue of $500,000 for the quarter, understanding that this is a subjective estimate that may require adjustments as more data becomes available.
Limitations
Highly subjective and prone to bias, reducing sales forecasting accuracy.
Relies heavily on the expertise and intuition of individuals, which may vary.
Lacks the robustness of data-driven methods, making it less suitable for precise sales projections.
Limited scalability in large organizations with complex sales cycles.
To learn more about other sales forecasting methods and explore real examples, download the eBook “13 Sales Forecasting Methods.
Most Common Mistakes and Pitfalls
Accurate sales forecasting is critical for predicting future sales, allocating resources, and achieving business growth. However, many companies face challenges that lead to inaccurate forecasts. Let’s explore the most common reasons behind failures in sales forecasting and how to overcome them using appropriate sales forecasting methods and tools.
1. Data Limitations
A lack of historical sales data is a common issue for startups and newly established businesses. Without a sales history, creating accurate sales forecasts can be challenging.
To address this:
Prioritize defining the data you need to collect and implement systems to gather it from the outset.
Employ qualitative sales forecasting or methods like pipeline-based forecasting, which rely on current sales pipeline insights rather than historical data. These techniques can provide valuable projections even with limited data.
2. Data Inaccuracy
Poor data quality is a leading cause of inaccurate sales forecasting. Ensuring data accuracy should be a top priority for any company aiming to improve forecast accuracy.
Common causes of data inaccuracy include:
Human error during manual data entry by sales reps.
Outdated or incomplete information in CRM systems.
Solutions:
Schedule regular data clean-up initiatives.
Use CRM rules and triggers to validate data.
Automate data enrichment processes, especially for customer information.
Ensure your CRM integrates seamlessly with other data sources.
Foster a culture of data accuracy within your team and offer incentives for maintaining clean data.
3. Irrelevant or Outdated Data
Even accurate data can lead to inaccurate forecasts if it is irrelevant or outdated. This issue is common among companies relying on spreadsheets for sales forecasting, as these tools often lack real-time updates and integration with CRM systems.
The solution:
Implement sales forecasting software that leverages real-time CRM data.
Replace outdated spreadsheet systems with tools that provide comprehensive and up-to-date sales forecasting models.
4. Lack of Stakeholder Buy-In
For sales forecasting to succeed, it must be ingrained in the company culture. Without the support of senior management and other stakeholders, forecasting accuracy can suffer.
Common challenges include:
Lack of prioritization of forecasting by leadership.
Perception that forecasting is unimportant for small businesses.
How to address this:
Emphasize the importance of sales forecasting accuracy for business survival and growth.
Demonstrate the value of accurate forecasts using techniques suited to the company’s size and stage.
5. Undefined Forecasting Process
Sales forecasting is an ongoing process, not a one-time task. Companies without a clear process often face challenges in achieving reliable sales projections.
Key components of a defined forecasting process:
Establish data entry standards for sales reps.
Schedule regular forecasting reviews and team meetings.
Deploy appropriate tools, such as sales forecasting software.
Continuously refine forecasting models based on feedback and outcomes.
6. Inappropriate Forecasting Methods
Choosing the wrong sales forecasting method is a common pitfall. Not all methods suit every business or situation, and selecting an inappropriate model can lead to inaccurate sales forecasting.
Examples of mismatches:
Time series forecasting requires sufficient historical data and is unsuitable for companies lacking this.
Sales cycle length forecasting doesn’t work effectively if the cycle is too short to yield meaningful insights.
Opportunity stage forecasting fails if your sales process lacks structure or sufficient data to calculate probabilities.
The solution:
Select the right sales forecasting method that aligns with your current data, process maturity, and goals.
7. Failure to Employ Forecasting Software
Many companies still rely on spreadsheets for sales forecasting, a practice that is both time-consuming and prone to errors. Spreadsheets lack the capabilities to implement advanced sales forecasting models effectively.
Challenges with spreadsheets:
Manual updates are labor-intensive and error-prone.
Limited support for complex forecasting techniques, such as Monte Carlo simulations or multivariable analysis forecasting.
Why use forecasting software:
Modern tools integrate seamlessly with CRM systems, enabling real-time data updates.
They support advanced methods like opportunity stage forecasting, historical forecasting, and lead-driven forecasting.
These tools are increasingly affordable and scalable for businesses of all sizes.
Recommendation:
Invest in sales forecasting software that aligns with your needs, offers robust capabilities, and requires minimal setup or implementation complexity.
Final Words on Sales Forecasting
Sales forecasting has been a cornerstone of my work since I first entered the B2B sales space. I am a strong advocate for the power of sales forecasting, believing it is essential for businesses striving to achieve predictable growth and improve sales performance.
No matter the size of the organization or the sales model it employs, I firmly believe that every company should prioritize sales forecasting. By dedicating time to refining their sales forecasting process and implementing strategies to enhance forecast accuracy, businesses can pave the way for sustainable and efficient growth.
Investing in accurate sales forecasts is not just about predicting revenue—it’s about making informed decisions that drive success.
To learn more about other sales forecasting methods and explore real examples, download the eBook “13 Sales Forecasting Methods.
Introduction
Sales forecasting has become a cornerstone of modern sales strategy, frequently appearing in countless articles, LinkedIn posts, and influencer blogs. Despite its widespread discussion among industry experts and the wealth of resources available, many organizations still struggle to achieve accurate sales forecasts. According to the Gartner State of Sales Operation Survey, over 50% of sales leaders express a lack of confidence in their sales forecasting accuracy—a gap that often leads to misaligned goals and missed opportunities.
From my own experience, sales forecasting has always been a challenging yet essential task. The ability to predict future sales not only drives strategic planning but also ensures better resource allocation, budget planning, and revenue forecasting. However, mastering sales forecasting requires more than just intuition—it demands time, effort, internal buy-in, and the right sales forecasting software.
In this article, we’ll explore why achieving accurate sales forecasting is so critical for B2B organizations. We’ll examine the best sales forecasting methods available, such as regression analysis, historical sales data forecasting, and qualitative forecasting, while also addressing the common pitfalls that sales leaders, sales reps, and RevOps teams encounter during the sales forecasting process. By understanding these challenges and leveraging historical sales data effectively, organizations can improve sales forecasting accuracy and drive better business outcomes.
The definition of sales forecasting
Sales forecasting is the practice of predicting future sales by analyzing various data points, such as historical sales data, current market trends, sales pipeline performance, and broader economic indicators. This forecasting process is vital for businesses to anticipate future revenue, enabling strategic planning, resource allocation, and improved sales performance. Accurate sales forecasting helps businesses stay ahead of market fluctuations and ensures they make informed decisions.
The responsibility for creating an accurate sales forecast often depends on the company's size, organizational structure, and stage of development. In startups and small businesses, the VP of Sales or Head of Sales typically oversees the sales forecasting process. These sales leaders rely on their intimate understanding of the sales pipeline and past sales data to create intuitive forecasts. This hands-on approach allows smaller teams to adapt quickly to changing conditions and provides flexibility during the early stages of growth.
As companies expand, their forecasting needs grow more complex. At this stage, sales forecasting is often handed over to dedicated teams such as Sales Operations or Revenue Operations (RevOps). These teams leverage advanced sales forecasting models, like regression analysis and multivariable analysis forecasting, to improve sales forecasting accuracy. They gather relevant data from historical sales figures, current market trends, and opportunity stage forecasting to align sales projections with the company’s broader strategic goals. The formation of such teams reflects a company’s evolution toward a data-driven forecasting process, ensuring accurate forecasts that drive strategic planning.
For larger organizations, sales forecasting becomes even more critical. With higher annual revenues, bigger budgets, and diverse sales teams, forecasting accuracy plays a pivotal role in maintaining operational efficiency and meeting stakeholder expectations. In these scenarios, relying on the right sales forecasting methods—such as time series analysis, AI forecasting, and qualitative forecasting —becomes essential. Inaccurate sales forecasting can lead to resource mismanagement, missed sales opportunities, and decreased revenue, underlining the importance of improving sales forecasting accuracy.
In summary, sales forecasting is a dynamic function that evolves with a company’s growth. While initially managed by sales leaders in smaller businesses, it transitions to specialized teams as organizations scale. Whether you're using sales forecasting software or leveraging qualitative sales forecasting techniques, understanding this progression is key to predicting future sales accurately and supporting long-term business success.
The difference between sales forecasting and sales planning
Sales forecasting and sales planning are two fundamental processes in any sales organization. While they are closely related, they serve distinct purposes and operate within different time frames. Together, they form a comprehensive approach to predict future sales and drive business success. Understanding the differences between these two concepts is crucial for sales leaders, sales managers, and RevOps teams aiming to improve sales performance and align strategic goals.
Sales Forecasting vs. Sales Planning: A Detailed Comparison
Sales forecasting focuses on predicting future sales revenue by analyzing historical sales data, market trends, and the sales pipeline. This process is best suited for short-term predictions, such as monthly or quarterly sales projections. Accurate forecasts help businesses allocate resources, improve sales forecasting accuracy, and adapt quickly to real-time changes in the market.
On the other hand, sales planning takes a broader, long-term perspective. It involves setting strategic sales targets, estimating the resources needed to achieve those goals, and preparing for various scenarios that could impact future revenue. Sales planning often includes what-if analyses to simulate potential market changes, shifts in sales performance, or other influencing factors.
Here’s a side-by-side comparison to highlight the key differences:
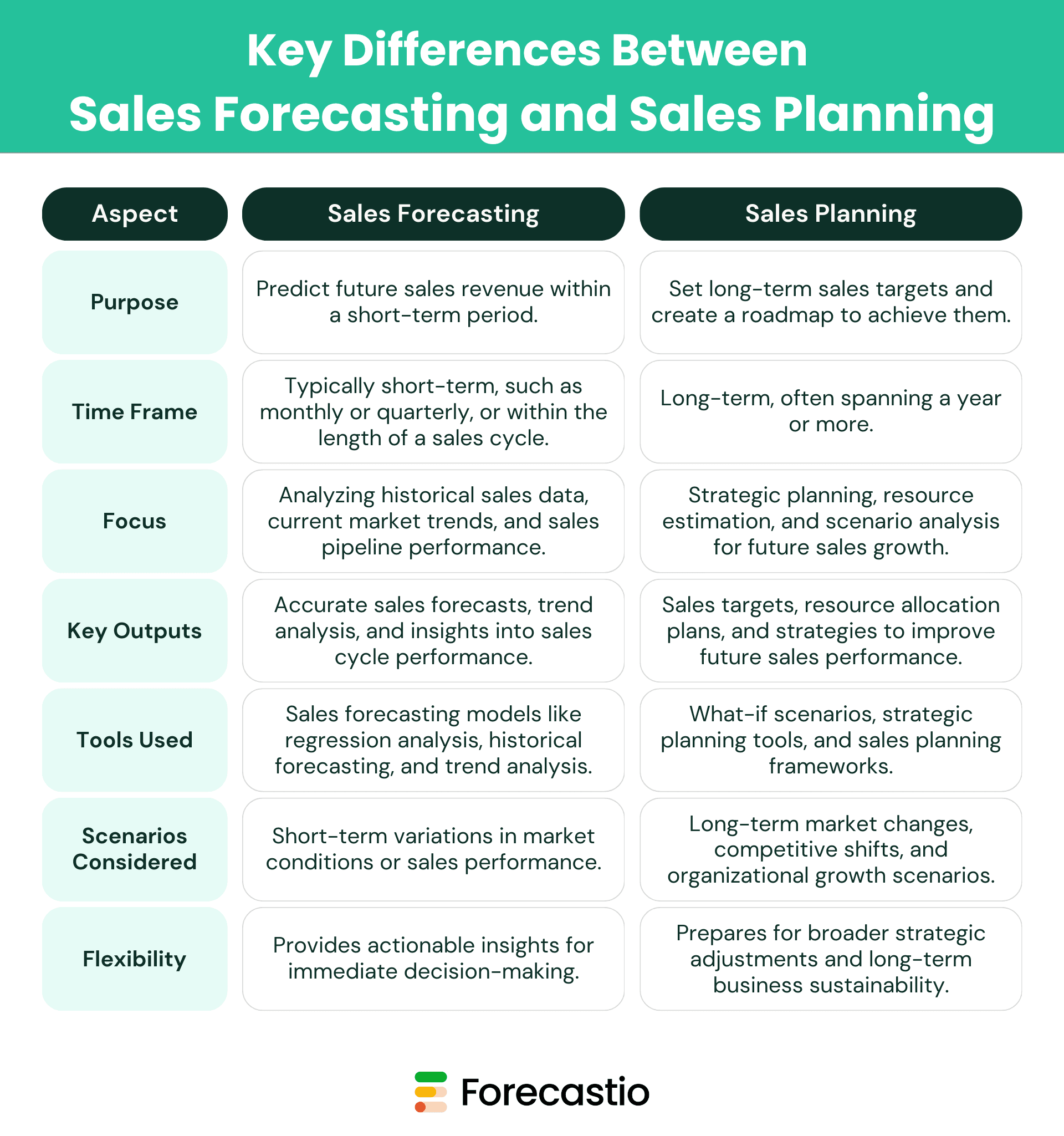
When to Use Sales Forecasting vs. Sales Planning
Sales forecasting is ideal for organizations that need to monitor sales performance closely and adjust their strategies in real time. It helps sales teams predict future sales within manageable time frames, ensuring efficient resource allocation and accurate forecasts.
Sales planning, on the other hand, is critical for companies aiming to set ambitious long-term goals and align their teams around a cohesive strategy. By considering potential market trends and future sales conditions, sales planning provides a robust framework for sustained growth and success.
Conclusion
While sales forecasting and sales planning are complementary processes, understanding their differences allows sales leaders, RevOps teams, and sales managers to use them effectively. Combining short-term forecasting accuracy with long-term strategic planning ensures businesses can navigate immediate challenges while staying focused on achieving their future sales targets.
By integrating accurate sales forecasting and comprehensive sales planning into their sales process, organizations can improve sales forecasting accuracy, anticipate future revenue, and drive long-term business success.
The importance of sales forecasting
"Accurately Predicting Sales is Impossible"—Is it Really?
Have you ever heard statements like, "Accurately predicting sales is impossible in this fast-changing market" or "As a startup, we’re too small to worry about forecasting; our priority is acquiring new accounts"? You’re not alone. Many businesses, especially startups, underestimate the vital role of accurate sales forecasting in ensuring long-term success.
To understand the significance of an accurate sales forecast, let’s explore the consequences of poor sales forecasting and its impact on business operations and growth:
1. Inefficient Resource Allocation
Inaccurate sales forecasts can lead to mismanagement of resources. Companies often overspend on marketing campaigns, hiring, sales tools, or product innovations, which drives up costs without improving efficiency. This misallocation not only reduces profitability but also increases cash burn—especially critical for startups operating on tight budgets.
2. Investor Confidence and Fundraising Challenges
Poor sales forecasting is often seen as a reflection of weak understanding of market trends, sales data, and revenue potential. Investors depend on reliable sales forecasting models to assess growth opportunities and risks. Overly optimistic or inconsistent sales projections can erode trust, damaging the company’s credibility and hindering its ability to raise funds.
3. Limited Investments in Growth and Innovation
Consistently missing sales projections creates unpredictability and reduces confidence in future forecasts. This uncertainty often forces businesses to prioritize managing cash risks over growth initiatives, such as scaling teams, investing in innovation, or experimenting with new sales strategies. Accurate forecasting is essential to balance these priorities effectively.
4. Leadership Credibility and Team Morale
Whether created by a Sales Leader, RevOps team, or Sales Operations department, inaccurate sales forecasting undermines leadership credibility. Missed revenue targets can highlight deficiencies in forecasting processes, historical sales data, tools, or team alignment. This not only casts doubt on leadership effectiveness but also negatively impacts team morale and sales performance.
Recognizing the importance of accurate sales forecasting is critical for business sustainability and growth. Leveraging the right sales forecasting methods can significantly improve sales forecasting accuracy.
Now, let’s delve into the best forecasting methods and models used by B2B sales organizations to predict future sales, align resources, and achieve reliable sales projections.
Sales forecasting methods
Sales forecasting is a critical aspect of strategic planning and revenue management, but there’s no universal solution. The 'one size fits all' approach simply doesn’t apply to sales forecasting methods.
Every company is unique, with its own set of influencing factors, such as:
The amount of historical sales data
The maturity of the sales process
The sales model (e.g., subscription-based, enterprise sales, or transactional sales)
Market dynamics and trends
The specific industry
These factors must be carefully considered when selecting the right sales forecasting method. Choosing a method that aligns with your business's structure and objectives can significantly improve sales forecasting accuracy and drive actionable insights.
Top-Down Sales Forecasting
Top-down sales forecasting begins with the big picture, analyzing overall market conditions, industry trends, and historical revenue data to predict future sales. This approach involves:
Assessing key market indicators, such as demand fluctuations and competitive dynamics.
Analyzing broad trends to estimate the total addressable market (TAM) and the company’s potential market share.
Using historical sales data and performance trends to refine projections.
This method works well for companies seeking to align sales strategies with macroeconomic conditions or when little granular data is available from sales reps. However, its effectiveness relies heavily on accurate trend analysis and a deep understanding of the market.
Bottom-Up Forecasting
In contrast, bottom-up forecasting starts at the individual level. This approach focuses on aggregating forecasts from sales reps, territories, or verticals to create a comprehensive forecast for the entire business. Key elements include:
Collecting individual sales projections during one-on-one meetings with sales reps.
Leveraging sales pipeline data and opportunity stage forecasting to assess potential revenue.
Summing up forecasts across teams, customer segments, or regions to provide a holistic sales projection.
Bottom-up forecasting benefits from the deep knowledge of sales reps, who are often closest to the customer and pipeline activity. This method is particularly effective for companies with a well-established sales process and a strong understanding of historical sales performance at the micro level.
Historical Sales Data Forecasting
Historical sales data forecasting is one of the most straightforward and widely used sales forecasting methods. It relies on analyzing past sales data to identify trends, seasonality, and patterns that can be used to predict future sales. This method is especially effective for companies with reliable and consistent historical revenue data and works well when external conditions, such as the market and industry trends, remain relatively stable.
When is Historical Sales Data Forecasting Applicable?
This method is best suited for:
Businesses with several years of historical sales data.
Stable industries with predictable sales cycles and minimal market disruption.
Companies looking for a straightforward approach to generate accurate sales forecasts without relying on complex models.
Scenarios where identifying seasonal trends or long-term patterns is necessary for resource planning, inventory management, and target setting.
However, it may not be ideal for businesses experiencing rapid changes in their sales process, market dynamics, or customer behavior, as it assumes past trends will continue into the future.
How Historical Forecasting Works
At its core, historical forecasting involves analyzing past sales performance to estimate future outcomes. Common techniques include the Simple Moving Average (SMA) approach.
Formula:
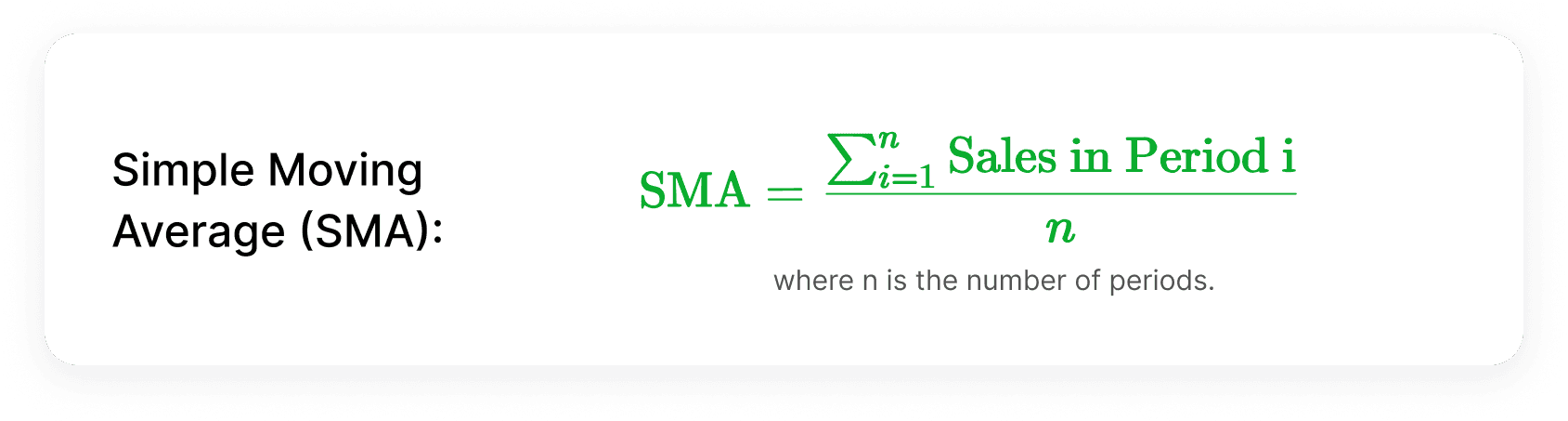
Where:
n = Number of periods used in the calculation.
Sales values are taken from historical sales data.
Example
A company wants to forecast sales for April using the sales data from January, February, and March:
January sales = $10,000
February sales = $12,000
March sales = $11,000
Using the SMA formula:
SMA for April = (10,000 + 12,000 + 11,000) / 3 = 11,000
The forecasted sales for April are $11,000
Opportunity Stage Forecasting
Opportunity stage forecasting is a sales forecasting method that evaluates deals in the sales pipeline based on their stage within the sales cycle. By assigning probabilities to each stage, businesses can estimate the likelihood of closing deals and generate more accurate sales forecasts. This method is particularly effective for companies with a well-defined sales process and a structured sales pipeline.
When is Opportunity Stage Forecasting Applicable?
This method works well for:
Companies with a mature sales process and clearly defined pipeline stages.
Businesses looking for a deal-by-deal approach to forecast future sales revenue.
Sales teams that rely on consistent sales rep input and CRM systems to track opportunities.
Scenarios where management wants to assess pipeline health and prioritize high-probability deals.
Opportunity stage forecasting is most applicable in environments with complex sales cycles, where deals progress through multiple stages before closing.
How Opportunity Stage Forecasting Works
Steps to Perform Opportunity Stage Forecasting
Define Sales Pipeline Stages
Clearly outline stages in the sales process, such as "Prospecting," "Discovery," "Proposal Sent," and "Negotiation."
Assign Probabilities to Each Stage
Assign a probability of closing for each stage based on historical data. For example:
Prospecting: 10%
Discovery: 30%
Proposal Sent: 60%
Negotiation: 80%
Closed-Won: 100%
Calculate Weighted Revenue for Each Opportunity
Multiply the deal value by the probability associated with its current stage.
Sum the Weighted Revenues
Add up all weighted revenues to forecast total pipeline revenue.
Example
A company has the following opportunities in its sales pipeline:
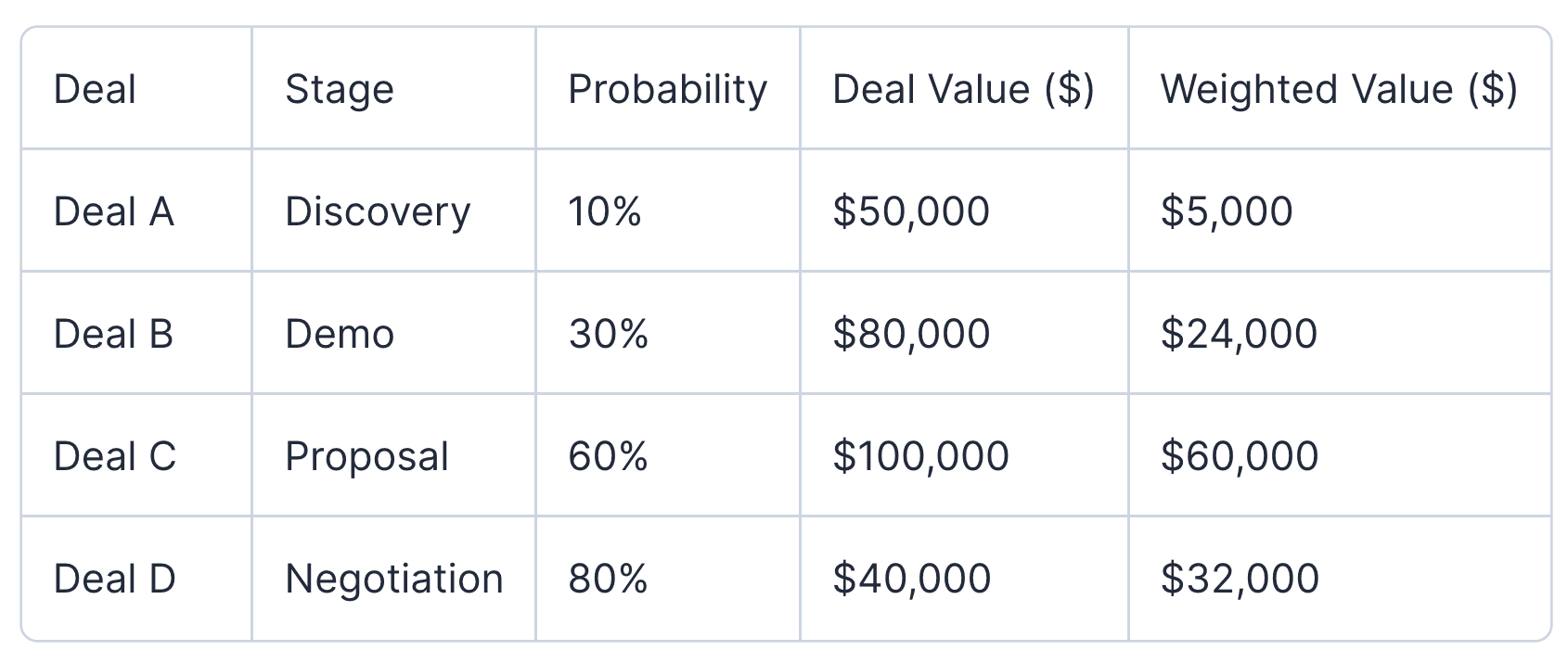
Total Forecasted Revenue = $5,000 + $24,000 + $60,000 + $32,000 = $121,000
Length of Sales Cycle Forecasting
Length of sales cycle forecasting is a method that predicts future sales revenue by analyzing the average time it takes for deals to progress through the sales cycle and close. By understanding the typical duration of a sales cycle, businesses can estimate when current opportunities are likely to close and generate accurate sales forecasts.
When is Length of Sales Cycle Forecasting Applicable?
This method is particularly effective for:
Companies with consistent and well-documented sales cycles.
Businesses tracking the time deals spend in each pipeline stage.
Organizations with repetitive or recurring sales processes, such as B2B subscription models.
Sales teams seek to predict revenue timing based on pipeline activity and deal velocity.
This approach works best in environments where the length of the sales cycle is stable and not influenced by external variables, such as market disruptions or significant changes in sales strategies.
How Length of Sales Cycle Forecasting Works
This method calculates the average duration of the sales cycle from initial contact to deal closure and applies this duration to current opportunities to estimate when revenue will be realized.
Steps to Perform Length of Sales Cycle Forecasting:
Analyze Historical Sales Data. Use historical revenue data to determine the average sales cycle length for past deals.
Segment Deals by Similar Characteristics. Group deals by size, industry, customer segment, or other relevant factors to identify patterns in the sales cycle.
Apply the Average Cycle Length to Current Opportunities. For each deal in the pipeline, estimate the closing date based on its entry date and the average cycle length.
Calculate Forecasted Revenue by Period. Sum the values of deals expected to close within a specific time frame (e.g., monthly or quarterly).
Limitations
Assumes sales cycle lengths remain consistent, which may not be true in dynamic markets.
Requires accurate tracking of deal entry dates and sales cycle duration.
Less effective for businesses with highly variable or undefined sales cycles.
To learn more about this method and explore real examples with numbers, download the eBook “13 Sales Forecasting Methods.
Lead-Driven Sales Forecasting
Lead-driven forecasting is a sales forecasting method that uses the volume of leads entering the sales pipeline and their historical conversion rates to estimate future sales revenue. This method ties sales projections directly to the performance of marketing and sales efforts, making it an essential tool for businesses with a high focus on lead generation and nurturing strategies.
When is Lead-Driven Forecasting Applicable?
Lead-driven forecasting is particularly effective for:
Companies with a predictable sales process and well-documented lead conversion rates.
Businesses that generate a high volume of leads through consistent sales strategies or marketing campaigns.
Organizations with a clear understanding of how lead quantity and quality impact sales performance.
Teams seek to predict future revenue based on the volume and behavior of leads entering the pipeline.
This method works best for companies with stable lead sources and consistent sales cycle durations.
How Lead-Driven Forecasting Works
This approach predicts future sales revenue by analyzing the following factors:
Lead Volume: The number of leads entering the pipeline within a specific period.
Conversion Rate: The historical percentage of leads that convert into closed deals.
Average Deal Value: The typical revenue generated from a single deal.
By multiplying these factors, businesses can estimate their total future sales revenue.
Formula:
Forecasted Revenue = Lead Volume X Conversion Rate X Average Deal Value
Limitations
Assumes consistent lead quality, which may not always be the case.
Relies on accurate tracking of leads and historical conversion data.
Less effective for companies with long or unpredictable sales cycles.
External factors, such as market changes or competitive dynamics, may impact actual sales outcomes.
To learn more about this method and explore real examples with numbers, download our free Sales Forecasting Guide.
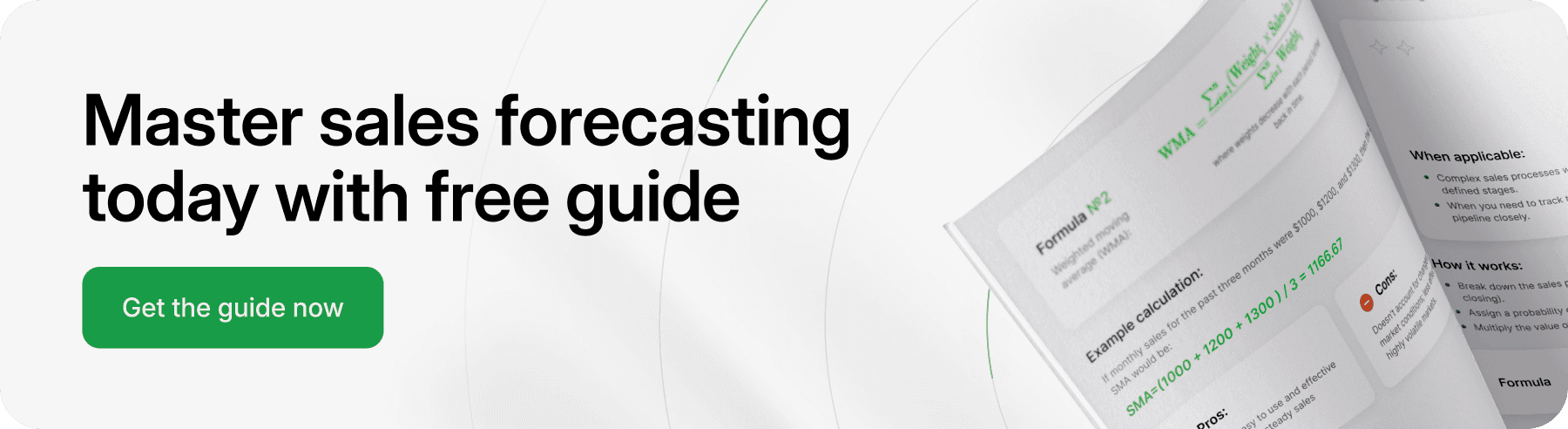
Forecasting based on deal probabilities
Forecasting based on deal probabilities is a method that predicts future sales revenue by assigning a probability of closure to each deal in the sales pipeline. This approach provides a granular, data-driven forecast that takes into account the likelihood of individual deals closing.
When is Forecasting Based on Deal Probabilities Applicable?
This method is particularly effective for:
Companies with a structured sales process and detailed deal tracking.
Businesses that manage a variety of deals with differing sizes, stages, and closing probabilities.
Teams looking to generate accurate sales forecasts by assessing the health of the sales pipeline at an individual deal level.
Organizations aiming to improve sales forecasting accuracy by incorporating real-time data into the forecasting process.
How Forecasting Based on Deal Probabilities Works
The method involves calculating the weighted value of each deal by multiplying its value by its probability of closing. These weighted values are then summed to create the total forecasted revenue.
Steps to Perform Deal Probability Forecasting:
Assign Probabilities to Each Deal. Determine the likelihood of each deal closing based on its stage in the sales cycle or other relevant factors.
Calculate Weighted Revenue. Multiply the deal value by its probability to determine its contribution to the forecast.
Sum the Weighted Values. Add up all weighted values to calculate the total forecasted revenue.
Example
A company has the following deals in its sales pipeline:
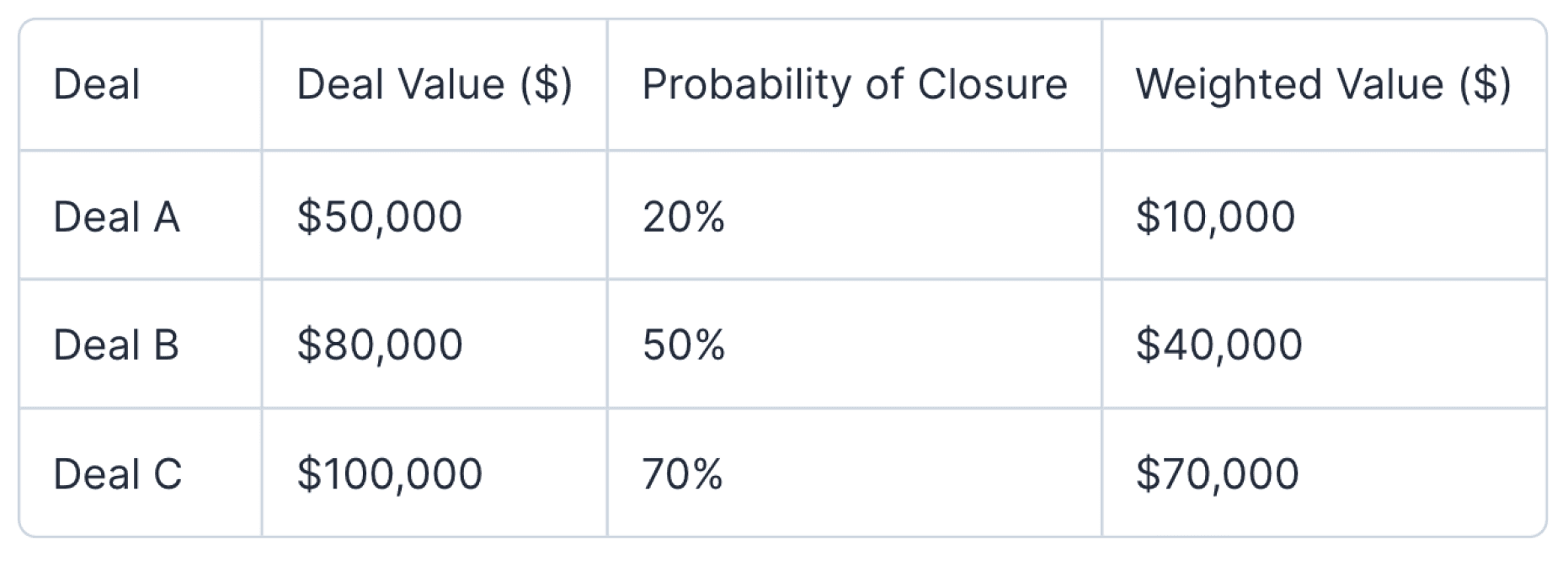
Forecasted Revenue = $10,000 + $40,000 + $70,000 = $120,000
Time Series Analysis
Time series analysis is a sales forecasting method that uses historical sales data to identify patterns, trends, and seasonality over time. By analyzing these recurring patterns, businesses can make data-driven predictions about future sales performance. This method is particularly effective for organizations with consistent sales data over an extended period and is widely used to create accurate forecasts.
When is Time Series Analysis Applicable?
This method is ideal for:
Companies with sufficient historical sales data to identify trends and patterns.
Businesses operating in industries with strong seasonality or predictable sales cycles.
Organizations looking to predict sales over specific time frames, such as monthly, quarterly, or annually.
Scenarios requiring long-term forecasts that incorporate historical trends and cyclicality.
Time series analysis works best when external market conditions and sales strategies remain relatively stable, ensuring historical patterns are a reliable predictor of future sales.
How Time Series Analysis Works
Time series analysis involves decomposing historical data into components such as trend, seasonality, and residuals. Common techniques include trend analysis, moving averages, and autoregressive models like ARIMA (Autoregressive Integrated Moving Average).
Steps in Time Series Analysis for Sales Forecasting:
Collect Historical Data
Use historical sales data to create a time series. Ensure data is consistent and spans a meaningful period.Decompose the Data
Break the time series into its components:Trend: Long-term direction of sales performance.
Seasonality: Regular, repeating patterns (e.g., higher sales in holiday seasons).
Residuals: Irregular variations or noise.
Choose a Time Series Model
Select an appropriate model, such as Simple Moving Average (SMA), ARIMA, or Exponential Smoothing, depending on the data’s complexity and objectives.Generate Forecasts
Use the model to predict future sales based on historical patterns and trends.
To learn more about this method and explore real examples with formulas and numbers, download the eBook “13 Sales Forecasting Methods.
Monte Carlo Simulation
The Monte Carlo method is a powerful and advanced sales forecasting technique that uses probability and random sampling to predict future sales performance. Unlike traditional deterministic methods, this approach accounts for uncertainty, variability, and randomness in the sales process, offering a range of possible outcomes rather than a single point estimate. It’s especially useful for businesses operating in complex, dynamic markets or those with high variability in sales cycles and deal sizes.
When is the Monte Carlo Method Applicable?
The Monte Carlo simulation is particularly effective for:
Companies dealing with uncertain variables, such as fluctuating sales cycles, conversion rates, or market conditions.
Businesses managing a large number of deals with varying probabilities of closure.
Scenarios requiring risk analysis or probabilistic forecasting to account for inaccurate sales forecasting or unpredictable events.
Organizations with access to robust sales forecasting software and relevant data for simulations.
It is ideal for businesses seeking to assess a range of potential outcomes and their likelihoods rather than relying on a single forecast.
How the Monte Carlo Method Works
The Monte Carlo simulation generates thousands of scenarios based on input variables (e.g., deal size, probability of closure, sales cycle length) to create a probabilistic distribution of outcomes. This allows businesses to see the likelihood of different revenue levels within a specified timeframe.
Steps to Perform Monte Carlo Simulation for Sales Forecasting:
Define Key Variables
Identify the variables that influence future sales revenue, such as deal size, conversion rates, sales cycle length, and lead volume.Set Probability Distributions
Assign probability distributions (e.g., normal, uniform) to each variable based on historical sales data or market trends.Run Simulations
Use random sampling techniques to simulate thousands (or more) possible outcomes by varying the input variables.Analyze Results
Examine the distribution of outcomes to identify likely revenue ranges, confidence intervals, and risks.
Formula
While the Monte Carlo method does not rely on a single formula, the general process involves:
Simulated Outcome = f (Random Inputs from Defined Distributions)
Where f is the model that calculates sales revenue based on the chosen input variables.
Example
A business has three key variables influencing sales revenue:
Number of Deals: Normally distributed with a mean of 50 and a standard deviation of 5.
Average Deal Size: Uniformly distributed between $10,000 and $15,000.
Conversion Rate: Normally distributed with a mean of 20% and a standard deviation of 3%.
Using these distributions, the Monte Carlo simulation generates thousands of random samples for each variable, calculates the revenue for each scenario, and creates a revenue distribution.
Sample Calculation for One Simulation:
Deals: 52 (randomly selected from the distribution)
Deal Size: $12,000 (randomly selected)
Conversion Rate: 22% (randomly selected)
Revenue for this simulation:
Revenue = 52 x 12,000 x 0.22 = $137, 280
Repeating this process thousands of times produces a range of potential revenues, such as:
10th Percentile: $800,000
50th Percentile (Median): $1,100,000
90th Percentile: $1,400,000
This result allows the business to predict future sales revenue within a probabilistic framework and assess risks.
Limitations
Requires significant data and expertise to set up and run simulations effectively.
Can be computationally intensive without access to the right tools.
Results depend heavily on the accuracy of input variables and probability distributions.
Not suitable for businesses with limited or poor-quality historical sales data.
Qualitative Sales Forecasting
Qualitative sales forecasting is a forecasting method that relies on subjective opinions and insights from sales reps, frontline teams, or industry experts rather than purely on historical sales data. This approach involves gathering individual perspectives to predict future sales performance, making it particularly useful in scenarios where quantitative data may be limited or unavailable.
When is Qualitative Sales Forecasting Applicable?
This method is commonly used in the following situations:
Startups or businesses without sufficient historical revenue data.
Industries experiencing rapid changes, where past trends may not reliably predict the future.
Companies entering new markets or launching new products without established sales histories.
Organizations seeking to incorporate on-the-ground insights from sales reps or subject matter experts.
While qualitative sales forecasting can offer valuable insights, its reliance on intuition and subjective judgments means it is best paired with other methods to enhance forecasting accuracy.
How Qualitative Sales Forecasting Works
This approach involves gathering insights and opinions from knowledgeable individuals through methods such as:
Pipeline Reviews
Sales managers and sales reps review the current sales pipeline, assess deals, and offer opinions on the likelihood of closing deals based on their sales process understanding.Expert Panels
A group of experts, such as industry leaders or market analysts, provides projections on future sales based on their understanding of market conditions, market trends, and competitive dynamics.Team Input
Frontline teams provide real-time feedback and intuitive insights during regular meetings, contributing to a more nuanced understanding of potential sales outcomes.Scenario Analysis
Opinions are gathered to simulate possible future scenarios, such as changes in sales strategies or shifts in customer behavior, to anticipate their impact on future sales revenue.
Key Features of Qualitative Sales Forecasting
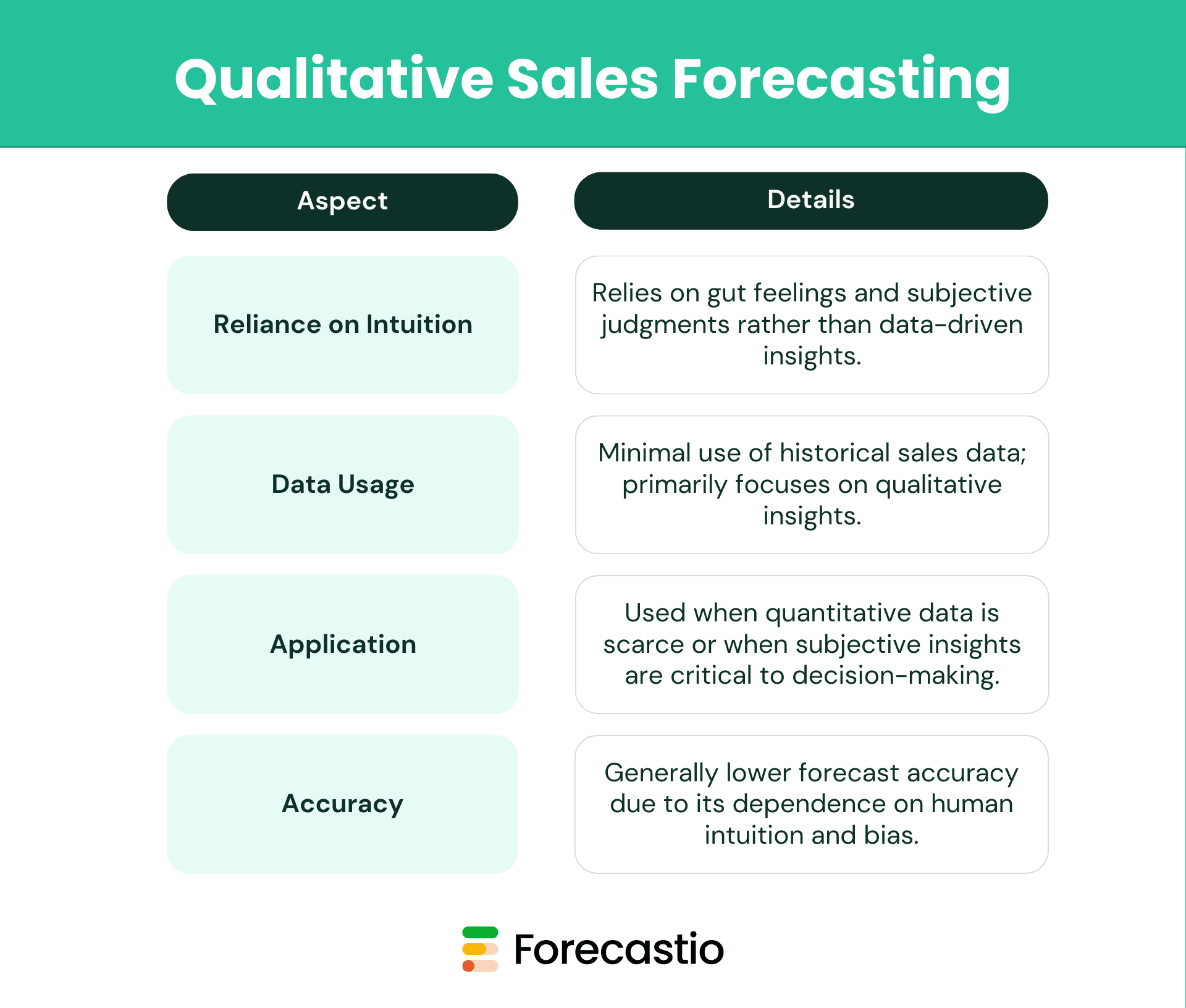
Example
Scenario: A company launching a new product in an unfamiliar market has no prior historical sales data. During a team meeting, sales reps provide opinions on the likelihood of closing deals in the current pipeline, while an expert panel forecasts sales growth based on anticipated customer demand and market trends.
Forecast: Based on this combined input, the company estimates potential revenue of $500,000 for the quarter, understanding that this is a subjective estimate that may require adjustments as more data becomes available.
Limitations
Highly subjective and prone to bias, reducing sales forecasting accuracy.
Relies heavily on the expertise and intuition of individuals, which may vary.
Lacks the robustness of data-driven methods, making it less suitable for precise sales projections.
Limited scalability in large organizations with complex sales cycles.
To learn more about other sales forecasting methods and explore real examples, download the eBook “13 Sales Forecasting Methods.
Most Common Mistakes and Pitfalls
Accurate sales forecasting is critical for predicting future sales, allocating resources, and achieving business growth. However, many companies face challenges that lead to inaccurate forecasts. Let’s explore the most common reasons behind failures in sales forecasting and how to overcome them using appropriate sales forecasting methods and tools.
1. Data Limitations
A lack of historical sales data is a common issue for startups and newly established businesses. Without a sales history, creating accurate sales forecasts can be challenging.
To address this:
Prioritize defining the data you need to collect and implement systems to gather it from the outset.
Employ qualitative sales forecasting or methods like pipeline-based forecasting, which rely on current sales pipeline insights rather than historical data. These techniques can provide valuable projections even with limited data.
2. Data Inaccuracy
Poor data quality is a leading cause of inaccurate sales forecasting. Ensuring data accuracy should be a top priority for any company aiming to improve forecast accuracy.
Common causes of data inaccuracy include:
Human error during manual data entry by sales reps.
Outdated or incomplete information in CRM systems.
Solutions:
Schedule regular data clean-up initiatives.
Use CRM rules and triggers to validate data.
Automate data enrichment processes, especially for customer information.
Ensure your CRM integrates seamlessly with other data sources.
Foster a culture of data accuracy within your team and offer incentives for maintaining clean data.
3. Irrelevant or Outdated Data
Even accurate data can lead to inaccurate forecasts if it is irrelevant or outdated. This issue is common among companies relying on spreadsheets for sales forecasting, as these tools often lack real-time updates and integration with CRM systems.
The solution:
Implement sales forecasting software that leverages real-time CRM data.
Replace outdated spreadsheet systems with tools that provide comprehensive and up-to-date sales forecasting models.
4. Lack of Stakeholder Buy-In
For sales forecasting to succeed, it must be ingrained in the company culture. Without the support of senior management and other stakeholders, forecasting accuracy can suffer.
Common challenges include:
Lack of prioritization of forecasting by leadership.
Perception that forecasting is unimportant for small businesses.
How to address this:
Emphasize the importance of sales forecasting accuracy for business survival and growth.
Demonstrate the value of accurate forecasts using techniques suited to the company’s size and stage.
5. Undefined Forecasting Process
Sales forecasting is an ongoing process, not a one-time task. Companies without a clear process often face challenges in achieving reliable sales projections.
Key components of a defined forecasting process:
Establish data entry standards for sales reps.
Schedule regular forecasting reviews and team meetings.
Deploy appropriate tools, such as sales forecasting software.
Continuously refine forecasting models based on feedback and outcomes.
6. Inappropriate Forecasting Methods
Choosing the wrong sales forecasting method is a common pitfall. Not all methods suit every business or situation, and selecting an inappropriate model can lead to inaccurate sales forecasting.
Examples of mismatches:
Time series forecasting requires sufficient historical data and is unsuitable for companies lacking this.
Sales cycle length forecasting doesn’t work effectively if the cycle is too short to yield meaningful insights.
Opportunity stage forecasting fails if your sales process lacks structure or sufficient data to calculate probabilities.
The solution:
Select the right sales forecasting method that aligns with your current data, process maturity, and goals.
7. Failure to Employ Forecasting Software
Many companies still rely on spreadsheets for sales forecasting, a practice that is both time-consuming and prone to errors. Spreadsheets lack the capabilities to implement advanced sales forecasting models effectively.
Challenges with spreadsheets:
Manual updates are labor-intensive and error-prone.
Limited support for complex forecasting techniques, such as Monte Carlo simulations or multivariable analysis forecasting.
Why use forecasting software:
Modern tools integrate seamlessly with CRM systems, enabling real-time data updates.
They support advanced methods like opportunity stage forecasting, historical forecasting, and lead-driven forecasting.
These tools are increasingly affordable and scalable for businesses of all sizes.
Recommendation:
Invest in sales forecasting software that aligns with your needs, offers robust capabilities, and requires minimal setup or implementation complexity.
Final Words on Sales Forecasting
Sales forecasting has been a cornerstone of my work since I first entered the B2B sales space. I am a strong advocate for the power of sales forecasting, believing it is essential for businesses striving to achieve predictable growth and improve sales performance.
No matter the size of the organization or the sales model it employs, I firmly believe that every company should prioritize sales forecasting. By dedicating time to refining their sales forecasting process and implementing strategies to enhance forecast accuracy, businesses can pave the way for sustainable and efficient growth.
Investing in accurate sales forecasts is not just about predicting revenue—it’s about making informed decisions that drive success.
To learn more about other sales forecasting methods and explore real examples, download the eBook “13 Sales Forecasting Methods.
Share:
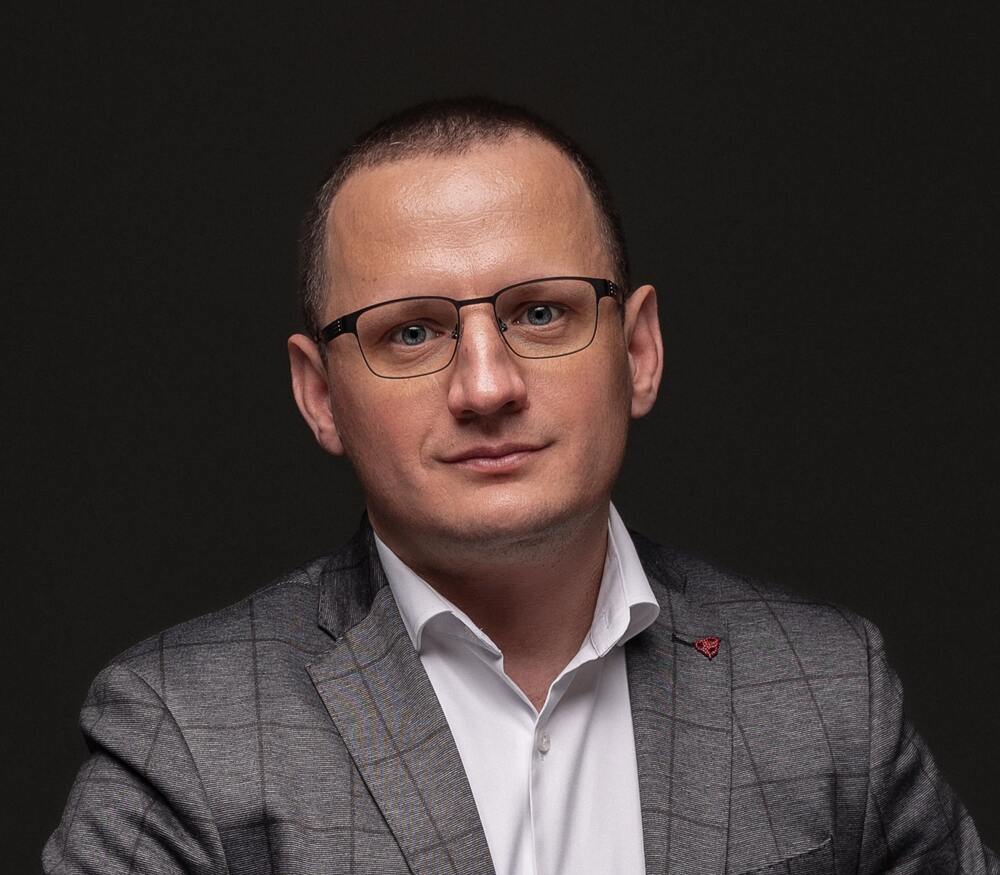
Alex is the CEO at Forecastio, bringing over 15 years of experience as a seasoned B2B sales expert and leader in the tech industry. His expertise lies in streamlining sales operations, developing robust go-to-market strategies, enhancing sales planning and forecasting, and refining sales processes.
Alex is the CEO at Forecastio, bringing over 15 years of experience as a seasoned B2B sales expert and leader in the tech industry. His expertise lies in streamlining sales operations, developing robust go-to-market strategies, enhancing sales planning and forecasting, and refining sales processes.
Related articles
Pipeline Management
Feb 17, 2025
9 min
Pipeline Management
Feb 17, 2025
9 min
Sales Performance
Feb 17, 2025
9 min
Sales Performance
Feb 17, 2025
9 min
Sales Strategies
Feb 17, 2025
15 min
Sales Strategies
Feb 17, 2025
15 min
Pipeline Management
Feb 17, 2025
9 min
Sales Performance
Feb 17, 2025
9 min
Pipeline Management
Feb 17, 2025
9 min
Sales Performance
Feb 17, 2025
9 min
Sales Planning
Sales Forecasting
Sales Performance Insights
Sales Planning
Sales Forecasting
Sales Performance Insights
Sales Planning
Sales Forecasting
Sales Performance Insights
© 2025 Forecastio, All rights reserved.
Sales Planning
Sales Forecasting
Sales Performance Insights
Sales Planning
Sales Forecasting
Sales Performance Insights
Sales Planning
Sales Forecasting
Sales Performance Insights
© 2025 Forecastio, All rights reserved.
Sales Planning
Sales Forecasting
Sales Performance Insights
Sales Planning
Sales Forecasting
Sales Performance Insights
Sales Planning
Sales Forecasting
Sales Performance Insights
© 2025 Forecastio, All rights reserved.
Sales Planning
Sales Forecasting
Sales Performance Insights
Sales Planning
Sales Forecasting
Sales Performance Insights
Sales Planning
Sales Forecasting
Sales Performance Insights
© 2025 Forecastio, All rights reserved.