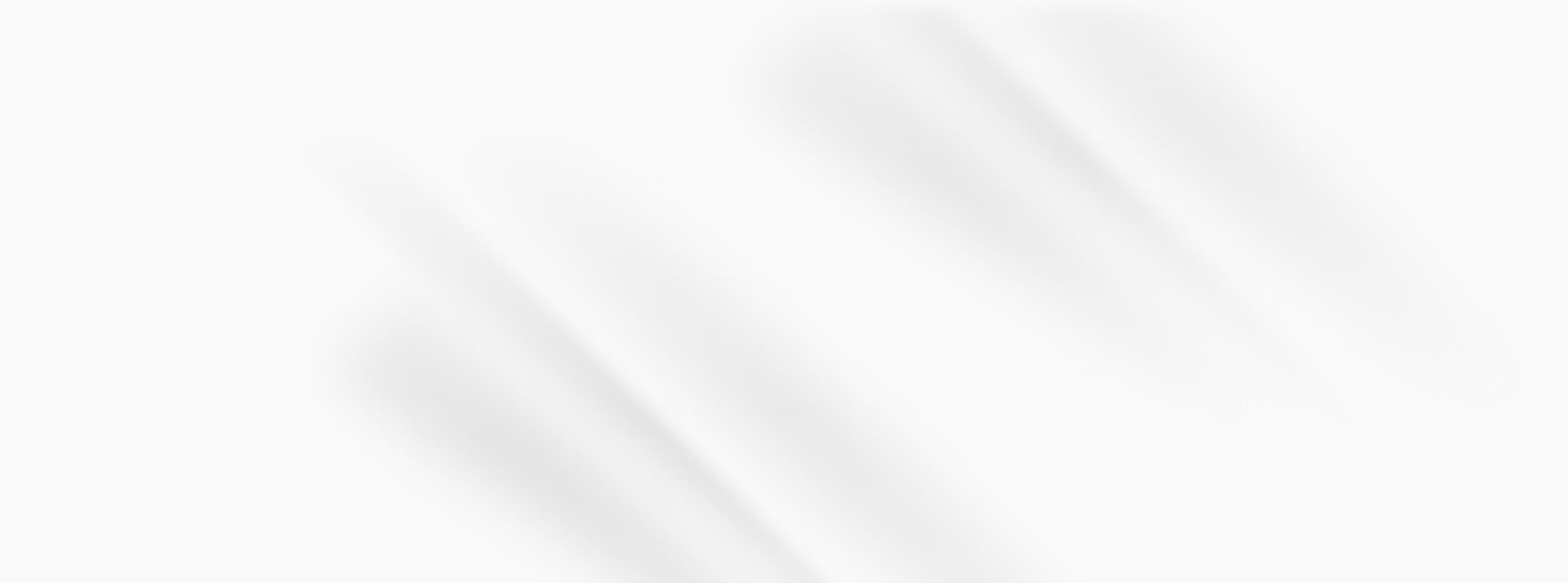
Sales Forecasting Examples That Actually Work
Apr 9, 2025
Apr 9, 2025
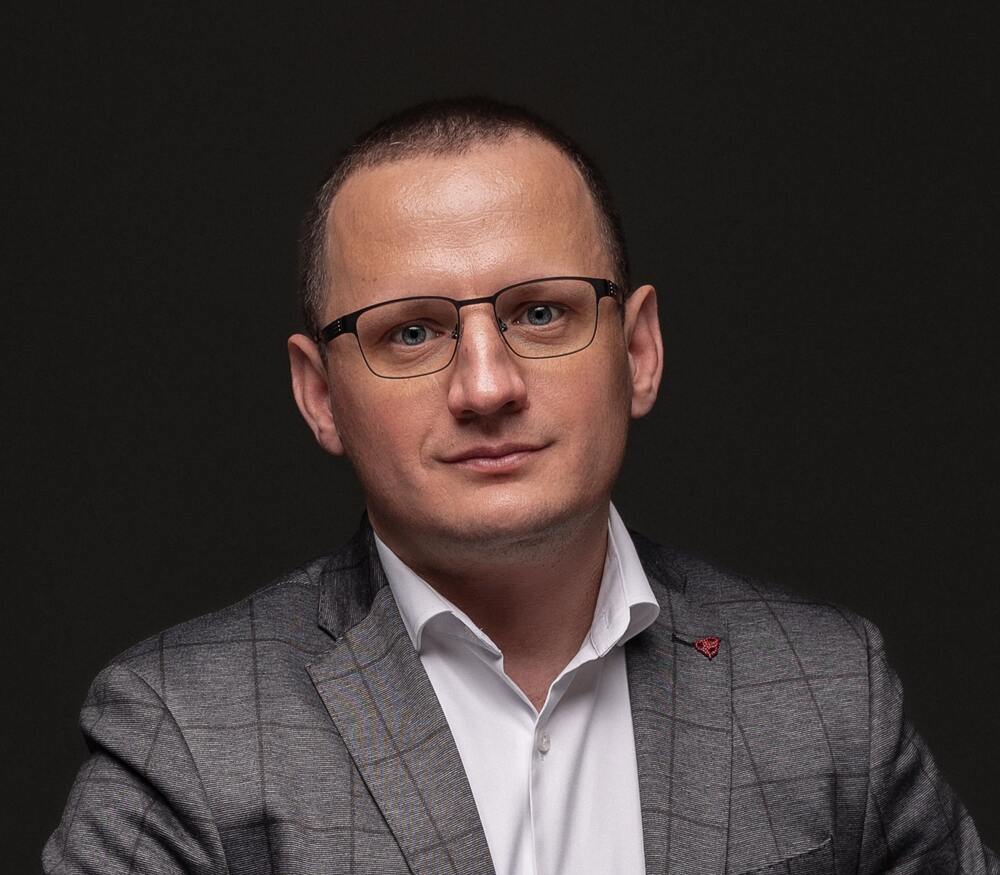
Alex Zlotko
CEO at Forecastio
Last updated
Apr 9, 2025
Reading time
11 min
Share:
Share
Table of Contents




Quick Take
Quick Take
Companies that forecast within 10% of actual results are twice as likely to grow year-over-year.
Calculate stage probabilities based on historical close rates –– if 30 out of 50 deals in Proposal stage historically closed, assign 60% probability.
Discount stalled deals that exceed normal timeframes –– if a deal sits in Proposal for 20 days when 10 is average, cut its probability in half.
Use deal age to predict closings –– a deal that's been open 30 days in a 60-day sales cycle has roughly 50% chance of closing.
For enterprise sales, use machine learning models that factor in multiple variables like stakeholder engagement and email response rates.
Companies that forecast within 10% of actual results are twice as likely to grow year-over-year.
Calculate stage probabilities based on historical close rates –– if 30 out of 50 deals in Proposal stage historically closed, assign 60% probability.
Discount stalled deals that exceed normal timeframes –– if a deal sits in Proposal for 20 days when 10 is average, cut its probability in half.
Use deal age to predict closings –– a deal that's been open 30 days in a 60-day sales cycle has roughly 50% chance of closing.
For enterprise sales, use machine learning models that factor in multiple variables like stakeholder engagement and email response rates.
Why Is Sales Forecasting Important?
Sales forecasting is the backbone of strategic decision-making in B2B companies. An accurate sales forecast empowers sales leaders and executives to predict future sales, allocate resources efficiently, and make confident, data-driven decisions. A reliable sales forecasting process is not just a reporting mechanism—it’s a strategic asset that drives future sales performance, helps you forecast sales with confidence, and fuels business growth.
When you create a sales forecast using reliable sales forecasting methods and historical sales data, you gain critical visibility into future revenue, enabling better planning across hiring, budgeting, and goal-setting. Companies that implement a structured forecasting process can avoid common pitfalls like over-hiring, under-investing, or missing sales targets due to unrealistic assumptions or inaccurate projections.
According to Gartner, companies that are able to forecast within 10% of actuals are more than twice as likely to experience consistent sales growth year over year. This highlights why sales forecasting is important—it gives organizations the clarity needed to navigate changing market conditions, anticipate market demand, and optimize their sales strategy.
Whether you use historical forecasting, sales pipeline insights, or intuitive sales forecasting, having a good sales forecast in place helps you stay aligned with business objectives and adjust to external factors that influence projected revenue. In short: better sales forecasts, better decisions—and better outcomes.
Why Does Sales Forecasting Fail?
Even though sales forecasting is important for strategic planning, many sales forecasts still miss the mark. The problem isn’t the idea of forecasting itself—it’s how the sales forecasting process is executed. Here are some of the most common reasons sales forecasts fail to deliver accurate, actionable insights:
Overreliance on intuition: Too often, sales reps rely on gut feelings instead of using data-driven sales forecasting methods. While intuitive forecasting may feel right, it rarely results in an accurate forecast without the support of solid historical data and sales performance metrics.
Stale or incomplete data: Outdated or missing sales data skews the forecast. Without clean, timely information from your sales pipeline, you can’t reliably predict future sales or estimate future sales revenue.
Poor CRM hygiene: If your sales team doesn’t maintain consistent deal stages or follow a clearly defined sales process, it becomes nearly impossible to forecast sales with precision. Sales forecasting tools can only work if the underlying sales data is trustworthy.
One-size-fits-all approach: Using the same forecasting method across different sales motions—like applying a sales cycle forecasting model built for enterprise sales to a transactional, high-velocity team leads to misaligned sales projections and inaccurate future performance estimates.
Lack of deal-level insights: Without factoring in deal-level risks—such as stalled opportunities, deals stuck in the sales cycle, or single-threaded conversations—the sales forecast becomes overly optimistic and unreliable. This is especially problematic when trying to meet tight sales targets or project future revenue.
In short, sales forecasting fails not because it’s an inherently flawed concept, but because of poor inputs, weak assumptions, and inconsistent execution. To create a sales forecast that drives decisions, it’s essential to choose the right sales forecasting method, ensure clean past sales data, and maintain a disciplined forecasting process that reflects your actual market conditions and sales strategy.
Sales Forecasting Examples
1. Forecasting by Opportunity Stage
This sales forecasting method uses your pipeline’s opportunity stages to generate a sales forecast. Each stage in the sales cycle—such as Discovery, Demo, Proposal, and Contract—is assigned a probability that reflects its historical conversion rate to closed-won deals. These probabilities are then applied to open deals to forecast sales and estimate future revenue.
This method is one of the most common sales forecast examples, especially in companies with structured pipelines and reliable sales data.
How to Calculate Stage Probabilities (Based on Historical Sales Data)
To assign probabilities to each stage, analyze your historical sales data to see what percentage of deals that entered a stage were ultimately won.
Stage | Deals Entered | Deals Won | Conversion Rate |
Discovery | 100 | 20 | 20% |
Demo | 80 | 32 | 40% |
Proposal | 50 | 30 | 60% |
Contract | 30 | 24 | 80% |
Formula: Probability = Deals Won / Deals Entered
Forecasting Example Using Stage Probabilities
Let’s apply the stage probabilities to active deals in your pipeline. This gives you a weighted sales projection—a realistic view of your future sales revenue based on where each deal currently stands.
Open Deals:
Deal | Stage | Deal Value | Stage Probability | Weighted Forecast |
Deal A | Proposal | $20,000 | 60% | $12,000 |
Deal B | Demo | $10,000 | 40% | $4,000 |
Deal C | Discovery | $15,000 | 20% | $3,000 |
Total Forecast = $12,000 + $4,000 + $3,000 = $19,000
This method is ideal when you have a consistent sales process, defined sales pipeline stages, and enough historical data to calculate meaningful stage conversion rates.
Try Forecastio to see how automatically calculated stage probabilities can boost your sales forecasting accuracy.
Pros:
Simple and intuitive
Doesn’t require advanced sales forecasting software
Works well when historical sales performance is consistent
Cons:
May overlook deal quality or sales rep behavior
Doesn’t account for stalled or high-risk deals
Can be skewed if past data is unreliable or incomplete
When to Use
Use this forecasting method when you have:
Clearly defined pipeline stages
Reliable historical sales data
A relatively stable sales cycle
This is a classic sales forecast example that helps sales leaders and sales managers build their first structured forecast based on pipeline health and historical performance. It’s also a useful starting point before moving to more advanced methods like AI-driven sales forecasting or sales cycle forecasting.
2. Advanced Opportunity Stage Forecasting
This advanced sales forecasting method builds upon traditional opportunity stage forecasting, but adds a critical adjustment: the time a deal spends in each stage. Deals that are stuck, stalled, or spend significantly more time than average in a stage have their stage probabilities discounted—making the sales forecast more grounded in reality.
It’s a powerful way to generate more accurate sales forecasts, especially in environments where deals often get delayed or stuck due to external factors, sales cycle length, or customer behavior.
How Probability Discounts Work for Stalled Deals
To make this forecasting process more realistic, you compare how long each deal has been in its current stage to the average sales cycle length for that stage. If a deal has been sitting in a stage for longer than average, you apply a discount to its probability.
Example: Adjusting Probabilities Based on Time-in-Stage
Let’s assume your historical data shows that the average time spent in each stage looks like this:
Stage | Avg Time in Stage |
Discovery | 5 days |
Demo | 7 days |
Proposal | 10 days |
Now let’s say:
Deal A has been in the Proposal stage for 20 days (2× the average).
Deal B has been in the Demo stage for 7 days (on schedule).
You can apply a simple discount rule. For example, reducing the probability by 50% if the time spent on stage exceeds 150% of the average.
Forecasting Example with Adjusted Probabilities
Deal | Stage | Deal Value | Standard Probability | Time in Stage | Adjusted Probability | Weighted Forecast |
Deal A | Proposal | $25,000 | 60% | 20 days | 30% (discounted) | $7,500 |
Deal B | Demo | $10,000 | 40% | 7 days | 40% (on track) | $4,000 |
Total Forecast = $7,500 + $4,000 = $11,500
This more advanced sales forecast example gives sales managers and sales leaders a clearer view of at-risk deals and helps prevent inflated sales projections caused by pipeline stagnation.
Pros:
More accurate than standard pipeline forecasting
Accounts for deal aging and pipeline health
Helps identify stuck deals that need attention
Cons:
Requires consistent CRM hygiene
Not easy to manage manually—benefits from sales forecasting tools
When to Use
Use this forecasting method when:
You already use pipeline stage forecasting
You want to reduce the risk of overforecasting
Your sales cycle includes deals that frequently stall
You aim to predict sales with greater precision by factoring in sales velocity
3. Historical Forecasting
Historical forecasting uses past sales data from previous time periods—typically quarters or years—to predict future sales. This sales forecasting method assumes that sales performance follows consistent patterns over time, making it especially useful for companies with clear seasonality, long sales cycles, or repeatable motions.
This is one of the most straightforward sales forecast examples and is often used as a baseline when other inputs, such as pipeline data, are limited or unreliable.
How It Works
Using historical sales data (e.g., bookings, closed-won revenue, or total sales), you can calculate growth trends and apply them to estimate future sales. A simple way to do this is to calculate a year-over-year growth rate and apply it to the most recent comparable period.
Forecasting Example Based on Historical Performance
Let’s say you’re forecasting for Q2 2024 using previous quarterly performance:
Period | Bookings | Growth Rate |
Q2 2023 | $100,000 | - |
Q2 2024 | $120,000 | +20% |
Q2 2025 | $144,000 | +20% (assumed) |
This sales forecast example is based purely on past sales trends. It assumes that your sales strategy, sales team, market demand, and sales cycle haven’t changed significantly year over year.
Pros:
Extremely easy to implement
Requires only past sales data
Useful for steady, repeatable businesses with consistent future sales revenue
Cons:
Ignores the current sales pipeline and sales reps' activity
Doesn’t reflect external factors, new competitors, or market trends
Risky if your business is scaling rapidly or experiencing disruption
When to Use
Use historical forecasting when:
Your business has steady sales cycles
Seasonality and trends are reliable
You need a fast, high-level forecast based on historical data
You want a baseline forecast before applying more advanced sales forecasting methods
Although this method doesn’t use sales forecasting tools or pipeline forecasting, it still plays a role in generating projected revenue—especially when comparing against more dynamic models. When combined with other approaches, it can help validate the accuracy of more complex sales forecasts.
4. Deal Probabilities Using Machine Learning
This advanced sales forecasting method uses machine learning (ML) to calculate win probabilities for each deal based on dozens (or even hundreds) of variables. Unlike traditional pipeline forecasting, which relies on static probabilities tied to stages, ML models analyze complex patterns in historical data to predict future sales more accurately.
By using sales forecasting tools with embedded ML capabilities, companies can dynamically adjust forecasts in real-time based on actual deal behavior, improving the odds of creating an accurate sales forecast.
ML Models Commonly Used in Sales Forecasting
Model Type | Use Case |
Logistic Regression | Predicts win/loss probability based on weighted deal features |
Random Forest | Analyzes deal attributes via decision trees to classify win/loss outcomes |
Gradient Boosting | Builds complex, layered models for more precise predictions |
Neural Networks | Ideal for large datasets with non-linear relationships |
Each of these models helps sales teams move beyond guesswork and static stage-based probabilities, toward more predictive sales forecasts rooted in behavioral and contextual data.
Example: Attributes Considered in ML Forecasting
ML models calculate deal-level win probabilities using a combination of deal, company, and rep-level features. Here’s a list of typical attributes:
Attribute | Description |
Stage | Current pipeline stage |
Time in Stage | Number of days deal has been in current stage |
Deal Age | Total time deal has been open |
Deal Amount | Monetary value of the opportunity |
Industry | Industry of the prospect/customer |
Company size | Size of the company based on employees or revenue |
Customer Engagement | Email opens, meeting attendance, replies, etc. |
Sales Rep Activities | Calls made, emails sent, follow-ups scheduled |
Previous Interactions | History of past deals with the account or contact |
Lead Source | Origin of the lead (inbound, outbound, referral, etc.) |
Competitors Involved | Whether other vendors are active in the deal |
These variables are fed into a model that’s trained on past sales data to predict future sales performance—providing a probability score that evolves over time.
Forecasting Example Using ML Probabilities
Let’s assume an ML model has already assigned win probabilities to open deals based on their attributes:
Deal | Value | ML Win Probability | Weighted Forecast |
Deal A | $50,000 | 35% | $17,500 |
Deal B | $30,000 | 70% | $21,000 |
Deal C | $40,000 | 15% | $6,000 |
Total Forecast = $17,500 + $21,000 + $6,000 = $44,500
This sales forecast example offers a far more nuanced and responsive estimate of future sales revenue than traditional methods.
Book a demo to see how Forecastio uses time-series analysis to reduce human bias and deliver highly accurate sales forecasts.
Pros:
Produces highly accurate forecasts
Adapts to changing sales performance and market conditions
Detects hidden patterns missed by manual analysis
Cons:
Requires large amounts of clean sales data
Implementation needs technical resources or a modern forecasting platform
Can be a “black box” for sales managers without data expertise
When to Use
Use ML-based sales forecasting when:
Your CRM is well-maintained and rich in historical sales data
You have a large volume of deals for training the model
Your organization is ready to invest in sales forecasting software or internal data science resources
You want to improve forecasting accuracy and reduce human bias
This is one of the most powerful and scalable sales forecast examples, giving sales leaders and revenue operations teams a reliable way to predict future sales with greater precision and confidence.
5. Length of Sales Cycle Forecasting
This sales forecasting method uses the length of the sales cycle to estimate a deal’s likelihood to close. Instead of looking at pipeline stages or historical forecasting alone, it evaluates where each deal is in relation to the average sales cycle length. The further along a deal is, the higher its predicted probability of closing—assuming a consistent sales process.
This model is particularly useful for doing time-based pipeline health checks and forecasting future revenue in high-velocity sales environments.
How It Works
Using past sales data, determine your average sales cycle length (e.g., 60 days). Then calculate the percentage of that cycle a current deal has completed.
Formula: Probability = Days in Sales Cycle / Average Sales Cycle Length
So if a deal has been open for 30 days and your average sales cycle is 60 days, it has completed 50% of the journey—translating to a 50% likelihood, assuming no other influencing factors.
This is a simple but effective sales forecast example for companies with high deal volume and minimal variability across opportunities.
Forecasting Example Based on Sales Cycle Progress
Let’s assume your historical data shows an average sales cycle of 60 days. You’re evaluating two active deals:
Deal | Deal Value | Days in Cycle | Avg Sales Cycle | Probability | Weighted Forecast |
Deal A | $40,000 | 30 days | 60 days | 50% | $20,000 |
Deal B | $20,000 | 15 days | 60 days | 25% | $5,000 |
Total Forecast = $20,000 + $5,000 = $25,000
This method helps sales managers and sales reps quickly forecast sales without requiring deep insights into customer engagement, rep activity, or deal quality.
Pros:
Easy to implement with past sales trends
Works well in high-volume pipelines with similar deal profiles
Ideal for quick health checks on pipeline progress and future sales
Cons:
Doesn’t account for deal quality, external factors, or sales rep performance
May mislead if sales cycle duration varies widely across deals
Not suitable for complex or long-cycle enterprise sales
When to Use
Use length of sales cycle forecasting when:
You have high deal volume and consistent sales cycle durations
Your team follows a uniform sales process
You want to estimate future sales quickly and simply
You’re doing top-level sales projections to guide resource allocation
This is a time-driven sales forecast example that’s especially effective in transactional sales models, giving sales leaders a simple, scalable approach to tracking progress and predicting future sales performance based on momentum.
Which Sales Forecasting Method to Choose?
There’s no universal approach to building an accurate sales forecast—the best sales forecasting method depends on your business model, team structure, and data maturity. In other words, sales forecasting isn’t one-size-fits-all. To forecast sales effectively and support decision-making, you need to choose the method that aligns best with your sales environment and available insights.
Here are the key factors to consider when deciding how to create a sales forecast:
✅ 1. Your Sales Motion
Is your team closing high-volume, fast-moving deals or navigating long, complex sales cycles?
High-velocity sales: Consider stage-based or length-of-sales-cycle forecasting. These models work well for quick, transactional sales processes where deal movement is frequent and predictable.
Enterprise sales: Leverage machine learning forecasting or advanced pipeline stage forecasting that accounts for multiple variables like deal risk, time in stage, and sales rep activity.
✅ 2. Data Availability and Quality
How much historical sales data do you have, and how reliable is it?
If your CRM contains consistent, clean past sales data, you can use more sophisticated sales forecasting tools that analyze market trends, customer behavior, and rep performance to produce more accurate sales forecasts.
If your data is limited or inconsistent, start simple with historical forecasting or stage-based models.
✅ 3. Team Size and CRM Discipline
Do you have a small sales team just getting started, or a mature organization with RevOps support?
Small team, short sales cycle: Start with classic pipeline forecasting using opportunity stage probabilities. It’s easy to implement and can help you calculate a sales forecast with minimal overhead.
Larger team with consistent CRM hygiene: Move toward ML-based forecasting or hybrid models that incorporate multiple data points to predict future sales more reliably.
Growing or hybrid sales org: Combine multiple sales forecasting methods—like weighting historical results alongside current sales pipeline trends and ML insights—for a blended and more accurate view of future revenue.
How to Improve Sales Forecasting Accuracy
Improving sales forecasting accuracy doesn’t require overhauling your entire process but it does demand consistency, clean data, and the right methods. Here’s how to create more accurate sales forecasts step by step:
Audit your CRM hygiene: standardize fields, ensure pipeline stages are clearly defined, and keep sales data updated.
Analyze past forecast accuracy: compare sales forecasts to actual results by rep, team, and method to spot patterns and gaps.
Segment your pipeline: separate enterprise deals from high-velocity deals—each needs its own sales forecasting method.
Use multiple forecasting models: blend historical forecasting, stage-based probabilities, and AI-driven forecasts to improve reliability.
Review and adjust forecasts weekly: make forecasting a routine part of the sales process not just a month-end task.
Incorporate deal-level risk: flag and discount stalled, single-threaded, or aging deals with longer-than-average sales cycles.
Invest in forecasting tools: Platforms like Forecastio automate complex calculations and help sales leaders make faster, data-driven decisions.
Summary
Sales forecasting in B2B is both an art and a science. From simple stage-based projections to advanced, AI-powered models, each sales forecasting method offers unique strengths. The key is selecting the right approach based on your sales motion, data maturity, and business goals.
Most importantly, treat sales forecasting as a dynamic, ongoing process—not just a one-time spreadsheet exercise. Regular updates, better inputs, and continuous learning will drive more accurate sales forecasts over time.
A good sales forecast doesn’t just predict future revenue—it shapes how you lead, plan, and scale your business with confidence.
Why Is Sales Forecasting Important?
Sales forecasting is the backbone of strategic decision-making in B2B companies. An accurate sales forecast empowers sales leaders and executives to predict future sales, allocate resources efficiently, and make confident, data-driven decisions. A reliable sales forecasting process is not just a reporting mechanism—it’s a strategic asset that drives future sales performance, helps you forecast sales with confidence, and fuels business growth.
When you create a sales forecast using reliable sales forecasting methods and historical sales data, you gain critical visibility into future revenue, enabling better planning across hiring, budgeting, and goal-setting. Companies that implement a structured forecasting process can avoid common pitfalls like over-hiring, under-investing, or missing sales targets due to unrealistic assumptions or inaccurate projections.
According to Gartner, companies that are able to forecast within 10% of actuals are more than twice as likely to experience consistent sales growth year over year. This highlights why sales forecasting is important—it gives organizations the clarity needed to navigate changing market conditions, anticipate market demand, and optimize their sales strategy.
Whether you use historical forecasting, sales pipeline insights, or intuitive sales forecasting, having a good sales forecast in place helps you stay aligned with business objectives and adjust to external factors that influence projected revenue. In short: better sales forecasts, better decisions—and better outcomes.
Why Does Sales Forecasting Fail?
Even though sales forecasting is important for strategic planning, many sales forecasts still miss the mark. The problem isn’t the idea of forecasting itself—it’s how the sales forecasting process is executed. Here are some of the most common reasons sales forecasts fail to deliver accurate, actionable insights:
Overreliance on intuition: Too often, sales reps rely on gut feelings instead of using data-driven sales forecasting methods. While intuitive forecasting may feel right, it rarely results in an accurate forecast without the support of solid historical data and sales performance metrics.
Stale or incomplete data: Outdated or missing sales data skews the forecast. Without clean, timely information from your sales pipeline, you can’t reliably predict future sales or estimate future sales revenue.
Poor CRM hygiene: If your sales team doesn’t maintain consistent deal stages or follow a clearly defined sales process, it becomes nearly impossible to forecast sales with precision. Sales forecasting tools can only work if the underlying sales data is trustworthy.
One-size-fits-all approach: Using the same forecasting method across different sales motions—like applying a sales cycle forecasting model built for enterprise sales to a transactional, high-velocity team leads to misaligned sales projections and inaccurate future performance estimates.
Lack of deal-level insights: Without factoring in deal-level risks—such as stalled opportunities, deals stuck in the sales cycle, or single-threaded conversations—the sales forecast becomes overly optimistic and unreliable. This is especially problematic when trying to meet tight sales targets or project future revenue.
In short, sales forecasting fails not because it’s an inherently flawed concept, but because of poor inputs, weak assumptions, and inconsistent execution. To create a sales forecast that drives decisions, it’s essential to choose the right sales forecasting method, ensure clean past sales data, and maintain a disciplined forecasting process that reflects your actual market conditions and sales strategy.
Sales Forecasting Examples
1. Forecasting by Opportunity Stage
This sales forecasting method uses your pipeline’s opportunity stages to generate a sales forecast. Each stage in the sales cycle—such as Discovery, Demo, Proposal, and Contract—is assigned a probability that reflects its historical conversion rate to closed-won deals. These probabilities are then applied to open deals to forecast sales and estimate future revenue.
This method is one of the most common sales forecast examples, especially in companies with structured pipelines and reliable sales data.
How to Calculate Stage Probabilities (Based on Historical Sales Data)
To assign probabilities to each stage, analyze your historical sales data to see what percentage of deals that entered a stage were ultimately won.
Stage | Deals Entered | Deals Won | Conversion Rate |
Discovery | 100 | 20 | 20% |
Demo | 80 | 32 | 40% |
Proposal | 50 | 30 | 60% |
Contract | 30 | 24 | 80% |
Formula: Probability = Deals Won / Deals Entered
Forecasting Example Using Stage Probabilities
Let’s apply the stage probabilities to active deals in your pipeline. This gives you a weighted sales projection—a realistic view of your future sales revenue based on where each deal currently stands.
Open Deals:
Deal | Stage | Deal Value | Stage Probability | Weighted Forecast |
Deal A | Proposal | $20,000 | 60% | $12,000 |
Deal B | Demo | $10,000 | 40% | $4,000 |
Deal C | Discovery | $15,000 | 20% | $3,000 |
Total Forecast = $12,000 + $4,000 + $3,000 = $19,000
This method is ideal when you have a consistent sales process, defined sales pipeline stages, and enough historical data to calculate meaningful stage conversion rates.
Try Forecastio to see how automatically calculated stage probabilities can boost your sales forecasting accuracy.
Pros:
Simple and intuitive
Doesn’t require advanced sales forecasting software
Works well when historical sales performance is consistent
Cons:
May overlook deal quality or sales rep behavior
Doesn’t account for stalled or high-risk deals
Can be skewed if past data is unreliable or incomplete
When to Use
Use this forecasting method when you have:
Clearly defined pipeline stages
Reliable historical sales data
A relatively stable sales cycle
This is a classic sales forecast example that helps sales leaders and sales managers build their first structured forecast based on pipeline health and historical performance. It’s also a useful starting point before moving to more advanced methods like AI-driven sales forecasting or sales cycle forecasting.
2. Advanced Opportunity Stage Forecasting
This advanced sales forecasting method builds upon traditional opportunity stage forecasting, but adds a critical adjustment: the time a deal spends in each stage. Deals that are stuck, stalled, or spend significantly more time than average in a stage have their stage probabilities discounted—making the sales forecast more grounded in reality.
It’s a powerful way to generate more accurate sales forecasts, especially in environments where deals often get delayed or stuck due to external factors, sales cycle length, or customer behavior.
How Probability Discounts Work for Stalled Deals
To make this forecasting process more realistic, you compare how long each deal has been in its current stage to the average sales cycle length for that stage. If a deal has been sitting in a stage for longer than average, you apply a discount to its probability.
Example: Adjusting Probabilities Based on Time-in-Stage
Let’s assume your historical data shows that the average time spent in each stage looks like this:
Stage | Avg Time in Stage |
Discovery | 5 days |
Demo | 7 days |
Proposal | 10 days |
Now let’s say:
Deal A has been in the Proposal stage for 20 days (2× the average).
Deal B has been in the Demo stage for 7 days (on schedule).
You can apply a simple discount rule. For example, reducing the probability by 50% if the time spent on stage exceeds 150% of the average.
Forecasting Example with Adjusted Probabilities
Deal | Stage | Deal Value | Standard Probability | Time in Stage | Adjusted Probability | Weighted Forecast |
Deal A | Proposal | $25,000 | 60% | 20 days | 30% (discounted) | $7,500 |
Deal B | Demo | $10,000 | 40% | 7 days | 40% (on track) | $4,000 |
Total Forecast = $7,500 + $4,000 = $11,500
This more advanced sales forecast example gives sales managers and sales leaders a clearer view of at-risk deals and helps prevent inflated sales projections caused by pipeline stagnation.
Pros:
More accurate than standard pipeline forecasting
Accounts for deal aging and pipeline health
Helps identify stuck deals that need attention
Cons:
Requires consistent CRM hygiene
Not easy to manage manually—benefits from sales forecasting tools
When to Use
Use this forecasting method when:
You already use pipeline stage forecasting
You want to reduce the risk of overforecasting
Your sales cycle includes deals that frequently stall
You aim to predict sales with greater precision by factoring in sales velocity
3. Historical Forecasting
Historical forecasting uses past sales data from previous time periods—typically quarters or years—to predict future sales. This sales forecasting method assumes that sales performance follows consistent patterns over time, making it especially useful for companies with clear seasonality, long sales cycles, or repeatable motions.
This is one of the most straightforward sales forecast examples and is often used as a baseline when other inputs, such as pipeline data, are limited or unreliable.
How It Works
Using historical sales data (e.g., bookings, closed-won revenue, or total sales), you can calculate growth trends and apply them to estimate future sales. A simple way to do this is to calculate a year-over-year growth rate and apply it to the most recent comparable period.
Forecasting Example Based on Historical Performance
Let’s say you’re forecasting for Q2 2024 using previous quarterly performance:
Period | Bookings | Growth Rate |
Q2 2023 | $100,000 | - |
Q2 2024 | $120,000 | +20% |
Q2 2025 | $144,000 | +20% (assumed) |
This sales forecast example is based purely on past sales trends. It assumes that your sales strategy, sales team, market demand, and sales cycle haven’t changed significantly year over year.
Pros:
Extremely easy to implement
Requires only past sales data
Useful for steady, repeatable businesses with consistent future sales revenue
Cons:
Ignores the current sales pipeline and sales reps' activity
Doesn’t reflect external factors, new competitors, or market trends
Risky if your business is scaling rapidly or experiencing disruption
When to Use
Use historical forecasting when:
Your business has steady sales cycles
Seasonality and trends are reliable
You need a fast, high-level forecast based on historical data
You want a baseline forecast before applying more advanced sales forecasting methods
Although this method doesn’t use sales forecasting tools or pipeline forecasting, it still plays a role in generating projected revenue—especially when comparing against more dynamic models. When combined with other approaches, it can help validate the accuracy of more complex sales forecasts.
4. Deal Probabilities Using Machine Learning
This advanced sales forecasting method uses machine learning (ML) to calculate win probabilities for each deal based on dozens (or even hundreds) of variables. Unlike traditional pipeline forecasting, which relies on static probabilities tied to stages, ML models analyze complex patterns in historical data to predict future sales more accurately.
By using sales forecasting tools with embedded ML capabilities, companies can dynamically adjust forecasts in real-time based on actual deal behavior, improving the odds of creating an accurate sales forecast.
ML Models Commonly Used in Sales Forecasting
Model Type | Use Case |
Logistic Regression | Predicts win/loss probability based on weighted deal features |
Random Forest | Analyzes deal attributes via decision trees to classify win/loss outcomes |
Gradient Boosting | Builds complex, layered models for more precise predictions |
Neural Networks | Ideal for large datasets with non-linear relationships |
Each of these models helps sales teams move beyond guesswork and static stage-based probabilities, toward more predictive sales forecasts rooted in behavioral and contextual data.
Example: Attributes Considered in ML Forecasting
ML models calculate deal-level win probabilities using a combination of deal, company, and rep-level features. Here’s a list of typical attributes:
Attribute | Description |
Stage | Current pipeline stage |
Time in Stage | Number of days deal has been in current stage |
Deal Age | Total time deal has been open |
Deal Amount | Monetary value of the opportunity |
Industry | Industry of the prospect/customer |
Company size | Size of the company based on employees or revenue |
Customer Engagement | Email opens, meeting attendance, replies, etc. |
Sales Rep Activities | Calls made, emails sent, follow-ups scheduled |
Previous Interactions | History of past deals with the account or contact |
Lead Source | Origin of the lead (inbound, outbound, referral, etc.) |
Competitors Involved | Whether other vendors are active in the deal |
These variables are fed into a model that’s trained on past sales data to predict future sales performance—providing a probability score that evolves over time.
Forecasting Example Using ML Probabilities
Let’s assume an ML model has already assigned win probabilities to open deals based on their attributes:
Deal | Value | ML Win Probability | Weighted Forecast |
Deal A | $50,000 | 35% | $17,500 |
Deal B | $30,000 | 70% | $21,000 |
Deal C | $40,000 | 15% | $6,000 |
Total Forecast = $17,500 + $21,000 + $6,000 = $44,500
This sales forecast example offers a far more nuanced and responsive estimate of future sales revenue than traditional methods.
Book a demo to see how Forecastio uses time-series analysis to reduce human bias and deliver highly accurate sales forecasts.
Pros:
Produces highly accurate forecasts
Adapts to changing sales performance and market conditions
Detects hidden patterns missed by manual analysis
Cons:
Requires large amounts of clean sales data
Implementation needs technical resources or a modern forecasting platform
Can be a “black box” for sales managers without data expertise
When to Use
Use ML-based sales forecasting when:
Your CRM is well-maintained and rich in historical sales data
You have a large volume of deals for training the model
Your organization is ready to invest in sales forecasting software or internal data science resources
You want to improve forecasting accuracy and reduce human bias
This is one of the most powerful and scalable sales forecast examples, giving sales leaders and revenue operations teams a reliable way to predict future sales with greater precision and confidence.
5. Length of Sales Cycle Forecasting
This sales forecasting method uses the length of the sales cycle to estimate a deal’s likelihood to close. Instead of looking at pipeline stages or historical forecasting alone, it evaluates where each deal is in relation to the average sales cycle length. The further along a deal is, the higher its predicted probability of closing—assuming a consistent sales process.
This model is particularly useful for doing time-based pipeline health checks and forecasting future revenue in high-velocity sales environments.
How It Works
Using past sales data, determine your average sales cycle length (e.g., 60 days). Then calculate the percentage of that cycle a current deal has completed.
Formula: Probability = Days in Sales Cycle / Average Sales Cycle Length
So if a deal has been open for 30 days and your average sales cycle is 60 days, it has completed 50% of the journey—translating to a 50% likelihood, assuming no other influencing factors.
This is a simple but effective sales forecast example for companies with high deal volume and minimal variability across opportunities.
Forecasting Example Based on Sales Cycle Progress
Let’s assume your historical data shows an average sales cycle of 60 days. You’re evaluating two active deals:
Deal | Deal Value | Days in Cycle | Avg Sales Cycle | Probability | Weighted Forecast |
Deal A | $40,000 | 30 days | 60 days | 50% | $20,000 |
Deal B | $20,000 | 15 days | 60 days | 25% | $5,000 |
Total Forecast = $20,000 + $5,000 = $25,000
This method helps sales managers and sales reps quickly forecast sales without requiring deep insights into customer engagement, rep activity, or deal quality.
Pros:
Easy to implement with past sales trends
Works well in high-volume pipelines with similar deal profiles
Ideal for quick health checks on pipeline progress and future sales
Cons:
Doesn’t account for deal quality, external factors, or sales rep performance
May mislead if sales cycle duration varies widely across deals
Not suitable for complex or long-cycle enterprise sales
When to Use
Use length of sales cycle forecasting when:
You have high deal volume and consistent sales cycle durations
Your team follows a uniform sales process
You want to estimate future sales quickly and simply
You’re doing top-level sales projections to guide resource allocation
This is a time-driven sales forecast example that’s especially effective in transactional sales models, giving sales leaders a simple, scalable approach to tracking progress and predicting future sales performance based on momentum.
Which Sales Forecasting Method to Choose?
There’s no universal approach to building an accurate sales forecast—the best sales forecasting method depends on your business model, team structure, and data maturity. In other words, sales forecasting isn’t one-size-fits-all. To forecast sales effectively and support decision-making, you need to choose the method that aligns best with your sales environment and available insights.
Here are the key factors to consider when deciding how to create a sales forecast:
✅ 1. Your Sales Motion
Is your team closing high-volume, fast-moving deals or navigating long, complex sales cycles?
High-velocity sales: Consider stage-based or length-of-sales-cycle forecasting. These models work well for quick, transactional sales processes where deal movement is frequent and predictable.
Enterprise sales: Leverage machine learning forecasting or advanced pipeline stage forecasting that accounts for multiple variables like deal risk, time in stage, and sales rep activity.
✅ 2. Data Availability and Quality
How much historical sales data do you have, and how reliable is it?
If your CRM contains consistent, clean past sales data, you can use more sophisticated sales forecasting tools that analyze market trends, customer behavior, and rep performance to produce more accurate sales forecasts.
If your data is limited or inconsistent, start simple with historical forecasting or stage-based models.
✅ 3. Team Size and CRM Discipline
Do you have a small sales team just getting started, or a mature organization with RevOps support?
Small team, short sales cycle: Start with classic pipeline forecasting using opportunity stage probabilities. It’s easy to implement and can help you calculate a sales forecast with minimal overhead.
Larger team with consistent CRM hygiene: Move toward ML-based forecasting or hybrid models that incorporate multiple data points to predict future sales more reliably.
Growing or hybrid sales org: Combine multiple sales forecasting methods—like weighting historical results alongside current sales pipeline trends and ML insights—for a blended and more accurate view of future revenue.
How to Improve Sales Forecasting Accuracy
Improving sales forecasting accuracy doesn’t require overhauling your entire process but it does demand consistency, clean data, and the right methods. Here’s how to create more accurate sales forecasts step by step:
Audit your CRM hygiene: standardize fields, ensure pipeline stages are clearly defined, and keep sales data updated.
Analyze past forecast accuracy: compare sales forecasts to actual results by rep, team, and method to spot patterns and gaps.
Segment your pipeline: separate enterprise deals from high-velocity deals—each needs its own sales forecasting method.
Use multiple forecasting models: blend historical forecasting, stage-based probabilities, and AI-driven forecasts to improve reliability.
Review and adjust forecasts weekly: make forecasting a routine part of the sales process not just a month-end task.
Incorporate deal-level risk: flag and discount stalled, single-threaded, or aging deals with longer-than-average sales cycles.
Invest in forecasting tools: Platforms like Forecastio automate complex calculations and help sales leaders make faster, data-driven decisions.
Summary
Sales forecasting in B2B is both an art and a science. From simple stage-based projections to advanced, AI-powered models, each sales forecasting method offers unique strengths. The key is selecting the right approach based on your sales motion, data maturity, and business goals.
Most importantly, treat sales forecasting as a dynamic, ongoing process—not just a one-time spreadsheet exercise. Regular updates, better inputs, and continuous learning will drive more accurate sales forecasts over time.
A good sales forecast doesn’t just predict future revenue—it shapes how you lead, plan, and scale your business with confidence.
Why Is Sales Forecasting Important?
Sales forecasting is the backbone of strategic decision-making in B2B companies. An accurate sales forecast empowers sales leaders and executives to predict future sales, allocate resources efficiently, and make confident, data-driven decisions. A reliable sales forecasting process is not just a reporting mechanism—it’s a strategic asset that drives future sales performance, helps you forecast sales with confidence, and fuels business growth.
When you create a sales forecast using reliable sales forecasting methods and historical sales data, you gain critical visibility into future revenue, enabling better planning across hiring, budgeting, and goal-setting. Companies that implement a structured forecasting process can avoid common pitfalls like over-hiring, under-investing, or missing sales targets due to unrealistic assumptions or inaccurate projections.
According to Gartner, companies that are able to forecast within 10% of actuals are more than twice as likely to experience consistent sales growth year over year. This highlights why sales forecasting is important—it gives organizations the clarity needed to navigate changing market conditions, anticipate market demand, and optimize their sales strategy.
Whether you use historical forecasting, sales pipeline insights, or intuitive sales forecasting, having a good sales forecast in place helps you stay aligned with business objectives and adjust to external factors that influence projected revenue. In short: better sales forecasts, better decisions—and better outcomes.
Why Does Sales Forecasting Fail?
Even though sales forecasting is important for strategic planning, many sales forecasts still miss the mark. The problem isn’t the idea of forecasting itself—it’s how the sales forecasting process is executed. Here are some of the most common reasons sales forecasts fail to deliver accurate, actionable insights:
Overreliance on intuition: Too often, sales reps rely on gut feelings instead of using data-driven sales forecasting methods. While intuitive forecasting may feel right, it rarely results in an accurate forecast without the support of solid historical data and sales performance metrics.
Stale or incomplete data: Outdated or missing sales data skews the forecast. Without clean, timely information from your sales pipeline, you can’t reliably predict future sales or estimate future sales revenue.
Poor CRM hygiene: If your sales team doesn’t maintain consistent deal stages or follow a clearly defined sales process, it becomes nearly impossible to forecast sales with precision. Sales forecasting tools can only work if the underlying sales data is trustworthy.
One-size-fits-all approach: Using the same forecasting method across different sales motions—like applying a sales cycle forecasting model built for enterprise sales to a transactional, high-velocity team leads to misaligned sales projections and inaccurate future performance estimates.
Lack of deal-level insights: Without factoring in deal-level risks—such as stalled opportunities, deals stuck in the sales cycle, or single-threaded conversations—the sales forecast becomes overly optimistic and unreliable. This is especially problematic when trying to meet tight sales targets or project future revenue.
In short, sales forecasting fails not because it’s an inherently flawed concept, but because of poor inputs, weak assumptions, and inconsistent execution. To create a sales forecast that drives decisions, it’s essential to choose the right sales forecasting method, ensure clean past sales data, and maintain a disciplined forecasting process that reflects your actual market conditions and sales strategy.
Sales Forecasting Examples
1. Forecasting by Opportunity Stage
This sales forecasting method uses your pipeline’s opportunity stages to generate a sales forecast. Each stage in the sales cycle—such as Discovery, Demo, Proposal, and Contract—is assigned a probability that reflects its historical conversion rate to closed-won deals. These probabilities are then applied to open deals to forecast sales and estimate future revenue.
This method is one of the most common sales forecast examples, especially in companies with structured pipelines and reliable sales data.
How to Calculate Stage Probabilities (Based on Historical Sales Data)
To assign probabilities to each stage, analyze your historical sales data to see what percentage of deals that entered a stage were ultimately won.
Stage | Deals Entered | Deals Won | Conversion Rate |
Discovery | 100 | 20 | 20% |
Demo | 80 | 32 | 40% |
Proposal | 50 | 30 | 60% |
Contract | 30 | 24 | 80% |
Formula: Probability = Deals Won / Deals Entered
Forecasting Example Using Stage Probabilities
Let’s apply the stage probabilities to active deals in your pipeline. This gives you a weighted sales projection—a realistic view of your future sales revenue based on where each deal currently stands.
Open Deals:
Deal | Stage | Deal Value | Stage Probability | Weighted Forecast |
Deal A | Proposal | $20,000 | 60% | $12,000 |
Deal B | Demo | $10,000 | 40% | $4,000 |
Deal C | Discovery | $15,000 | 20% | $3,000 |
Total Forecast = $12,000 + $4,000 + $3,000 = $19,000
This method is ideal when you have a consistent sales process, defined sales pipeline stages, and enough historical data to calculate meaningful stage conversion rates.
Try Forecastio to see how automatically calculated stage probabilities can boost your sales forecasting accuracy.
Pros:
Simple and intuitive
Doesn’t require advanced sales forecasting software
Works well when historical sales performance is consistent
Cons:
May overlook deal quality or sales rep behavior
Doesn’t account for stalled or high-risk deals
Can be skewed if past data is unreliable or incomplete
When to Use
Use this forecasting method when you have:
Clearly defined pipeline stages
Reliable historical sales data
A relatively stable sales cycle
This is a classic sales forecast example that helps sales leaders and sales managers build their first structured forecast based on pipeline health and historical performance. It’s also a useful starting point before moving to more advanced methods like AI-driven sales forecasting or sales cycle forecasting.
2. Advanced Opportunity Stage Forecasting
This advanced sales forecasting method builds upon traditional opportunity stage forecasting, but adds a critical adjustment: the time a deal spends in each stage. Deals that are stuck, stalled, or spend significantly more time than average in a stage have their stage probabilities discounted—making the sales forecast more grounded in reality.
It’s a powerful way to generate more accurate sales forecasts, especially in environments where deals often get delayed or stuck due to external factors, sales cycle length, or customer behavior.
How Probability Discounts Work for Stalled Deals
To make this forecasting process more realistic, you compare how long each deal has been in its current stage to the average sales cycle length for that stage. If a deal has been sitting in a stage for longer than average, you apply a discount to its probability.
Example: Adjusting Probabilities Based on Time-in-Stage
Let’s assume your historical data shows that the average time spent in each stage looks like this:
Stage | Avg Time in Stage |
Discovery | 5 days |
Demo | 7 days |
Proposal | 10 days |
Now let’s say:
Deal A has been in the Proposal stage for 20 days (2× the average).
Deal B has been in the Demo stage for 7 days (on schedule).
You can apply a simple discount rule. For example, reducing the probability by 50% if the time spent on stage exceeds 150% of the average.
Forecasting Example with Adjusted Probabilities
Deal | Stage | Deal Value | Standard Probability | Time in Stage | Adjusted Probability | Weighted Forecast |
Deal A | Proposal | $25,000 | 60% | 20 days | 30% (discounted) | $7,500 |
Deal B | Demo | $10,000 | 40% | 7 days | 40% (on track) | $4,000 |
Total Forecast = $7,500 + $4,000 = $11,500
This more advanced sales forecast example gives sales managers and sales leaders a clearer view of at-risk deals and helps prevent inflated sales projections caused by pipeline stagnation.
Pros:
More accurate than standard pipeline forecasting
Accounts for deal aging and pipeline health
Helps identify stuck deals that need attention
Cons:
Requires consistent CRM hygiene
Not easy to manage manually—benefits from sales forecasting tools
When to Use
Use this forecasting method when:
You already use pipeline stage forecasting
You want to reduce the risk of overforecasting
Your sales cycle includes deals that frequently stall
You aim to predict sales with greater precision by factoring in sales velocity
3. Historical Forecasting
Historical forecasting uses past sales data from previous time periods—typically quarters or years—to predict future sales. This sales forecasting method assumes that sales performance follows consistent patterns over time, making it especially useful for companies with clear seasonality, long sales cycles, or repeatable motions.
This is one of the most straightforward sales forecast examples and is often used as a baseline when other inputs, such as pipeline data, are limited or unreliable.
How It Works
Using historical sales data (e.g., bookings, closed-won revenue, or total sales), you can calculate growth trends and apply them to estimate future sales. A simple way to do this is to calculate a year-over-year growth rate and apply it to the most recent comparable period.
Forecasting Example Based on Historical Performance
Let’s say you’re forecasting for Q2 2024 using previous quarterly performance:
Period | Bookings | Growth Rate |
Q2 2023 | $100,000 | - |
Q2 2024 | $120,000 | +20% |
Q2 2025 | $144,000 | +20% (assumed) |
This sales forecast example is based purely on past sales trends. It assumes that your sales strategy, sales team, market demand, and sales cycle haven’t changed significantly year over year.
Pros:
Extremely easy to implement
Requires only past sales data
Useful for steady, repeatable businesses with consistent future sales revenue
Cons:
Ignores the current sales pipeline and sales reps' activity
Doesn’t reflect external factors, new competitors, or market trends
Risky if your business is scaling rapidly or experiencing disruption
When to Use
Use historical forecasting when:
Your business has steady sales cycles
Seasonality and trends are reliable
You need a fast, high-level forecast based on historical data
You want a baseline forecast before applying more advanced sales forecasting methods
Although this method doesn’t use sales forecasting tools or pipeline forecasting, it still plays a role in generating projected revenue—especially when comparing against more dynamic models. When combined with other approaches, it can help validate the accuracy of more complex sales forecasts.
4. Deal Probabilities Using Machine Learning
This advanced sales forecasting method uses machine learning (ML) to calculate win probabilities for each deal based on dozens (or even hundreds) of variables. Unlike traditional pipeline forecasting, which relies on static probabilities tied to stages, ML models analyze complex patterns in historical data to predict future sales more accurately.
By using sales forecasting tools with embedded ML capabilities, companies can dynamically adjust forecasts in real-time based on actual deal behavior, improving the odds of creating an accurate sales forecast.
ML Models Commonly Used in Sales Forecasting
Model Type | Use Case |
Logistic Regression | Predicts win/loss probability based on weighted deal features |
Random Forest | Analyzes deal attributes via decision trees to classify win/loss outcomes |
Gradient Boosting | Builds complex, layered models for more precise predictions |
Neural Networks | Ideal for large datasets with non-linear relationships |
Each of these models helps sales teams move beyond guesswork and static stage-based probabilities, toward more predictive sales forecasts rooted in behavioral and contextual data.
Example: Attributes Considered in ML Forecasting
ML models calculate deal-level win probabilities using a combination of deal, company, and rep-level features. Here’s a list of typical attributes:
Attribute | Description |
Stage | Current pipeline stage |
Time in Stage | Number of days deal has been in current stage |
Deal Age | Total time deal has been open |
Deal Amount | Monetary value of the opportunity |
Industry | Industry of the prospect/customer |
Company size | Size of the company based on employees or revenue |
Customer Engagement | Email opens, meeting attendance, replies, etc. |
Sales Rep Activities | Calls made, emails sent, follow-ups scheduled |
Previous Interactions | History of past deals with the account or contact |
Lead Source | Origin of the lead (inbound, outbound, referral, etc.) |
Competitors Involved | Whether other vendors are active in the deal |
These variables are fed into a model that’s trained on past sales data to predict future sales performance—providing a probability score that evolves over time.
Forecasting Example Using ML Probabilities
Let’s assume an ML model has already assigned win probabilities to open deals based on their attributes:
Deal | Value | ML Win Probability | Weighted Forecast |
Deal A | $50,000 | 35% | $17,500 |
Deal B | $30,000 | 70% | $21,000 |
Deal C | $40,000 | 15% | $6,000 |
Total Forecast = $17,500 + $21,000 + $6,000 = $44,500
This sales forecast example offers a far more nuanced and responsive estimate of future sales revenue than traditional methods.
Book a demo to see how Forecastio uses time-series analysis to reduce human bias and deliver highly accurate sales forecasts.
Pros:
Produces highly accurate forecasts
Adapts to changing sales performance and market conditions
Detects hidden patterns missed by manual analysis
Cons:
Requires large amounts of clean sales data
Implementation needs technical resources or a modern forecasting platform
Can be a “black box” for sales managers without data expertise
When to Use
Use ML-based sales forecasting when:
Your CRM is well-maintained and rich in historical sales data
You have a large volume of deals for training the model
Your organization is ready to invest in sales forecasting software or internal data science resources
You want to improve forecasting accuracy and reduce human bias
This is one of the most powerful and scalable sales forecast examples, giving sales leaders and revenue operations teams a reliable way to predict future sales with greater precision and confidence.
5. Length of Sales Cycle Forecasting
This sales forecasting method uses the length of the sales cycle to estimate a deal’s likelihood to close. Instead of looking at pipeline stages or historical forecasting alone, it evaluates where each deal is in relation to the average sales cycle length. The further along a deal is, the higher its predicted probability of closing—assuming a consistent sales process.
This model is particularly useful for doing time-based pipeline health checks and forecasting future revenue in high-velocity sales environments.
How It Works
Using past sales data, determine your average sales cycle length (e.g., 60 days). Then calculate the percentage of that cycle a current deal has completed.
Formula: Probability = Days in Sales Cycle / Average Sales Cycle Length
So if a deal has been open for 30 days and your average sales cycle is 60 days, it has completed 50% of the journey—translating to a 50% likelihood, assuming no other influencing factors.
This is a simple but effective sales forecast example for companies with high deal volume and minimal variability across opportunities.
Forecasting Example Based on Sales Cycle Progress
Let’s assume your historical data shows an average sales cycle of 60 days. You’re evaluating two active deals:
Deal | Deal Value | Days in Cycle | Avg Sales Cycle | Probability | Weighted Forecast |
Deal A | $40,000 | 30 days | 60 days | 50% | $20,000 |
Deal B | $20,000 | 15 days | 60 days | 25% | $5,000 |
Total Forecast = $20,000 + $5,000 = $25,000
This method helps sales managers and sales reps quickly forecast sales without requiring deep insights into customer engagement, rep activity, or deal quality.
Pros:
Easy to implement with past sales trends
Works well in high-volume pipelines with similar deal profiles
Ideal for quick health checks on pipeline progress and future sales
Cons:
Doesn’t account for deal quality, external factors, or sales rep performance
May mislead if sales cycle duration varies widely across deals
Not suitable for complex or long-cycle enterprise sales
When to Use
Use length of sales cycle forecasting when:
You have high deal volume and consistent sales cycle durations
Your team follows a uniform sales process
You want to estimate future sales quickly and simply
You’re doing top-level sales projections to guide resource allocation
This is a time-driven sales forecast example that’s especially effective in transactional sales models, giving sales leaders a simple, scalable approach to tracking progress and predicting future sales performance based on momentum.
Which Sales Forecasting Method to Choose?
There’s no universal approach to building an accurate sales forecast—the best sales forecasting method depends on your business model, team structure, and data maturity. In other words, sales forecasting isn’t one-size-fits-all. To forecast sales effectively and support decision-making, you need to choose the method that aligns best with your sales environment and available insights.
Here are the key factors to consider when deciding how to create a sales forecast:
✅ 1. Your Sales Motion
Is your team closing high-volume, fast-moving deals or navigating long, complex sales cycles?
High-velocity sales: Consider stage-based or length-of-sales-cycle forecasting. These models work well for quick, transactional sales processes where deal movement is frequent and predictable.
Enterprise sales: Leverage machine learning forecasting or advanced pipeline stage forecasting that accounts for multiple variables like deal risk, time in stage, and sales rep activity.
✅ 2. Data Availability and Quality
How much historical sales data do you have, and how reliable is it?
If your CRM contains consistent, clean past sales data, you can use more sophisticated sales forecasting tools that analyze market trends, customer behavior, and rep performance to produce more accurate sales forecasts.
If your data is limited or inconsistent, start simple with historical forecasting or stage-based models.
✅ 3. Team Size and CRM Discipline
Do you have a small sales team just getting started, or a mature organization with RevOps support?
Small team, short sales cycle: Start with classic pipeline forecasting using opportunity stage probabilities. It’s easy to implement and can help you calculate a sales forecast with minimal overhead.
Larger team with consistent CRM hygiene: Move toward ML-based forecasting or hybrid models that incorporate multiple data points to predict future sales more reliably.
Growing or hybrid sales org: Combine multiple sales forecasting methods—like weighting historical results alongside current sales pipeline trends and ML insights—for a blended and more accurate view of future revenue.
How to Improve Sales Forecasting Accuracy
Improving sales forecasting accuracy doesn’t require overhauling your entire process but it does demand consistency, clean data, and the right methods. Here’s how to create more accurate sales forecasts step by step:
Audit your CRM hygiene: standardize fields, ensure pipeline stages are clearly defined, and keep sales data updated.
Analyze past forecast accuracy: compare sales forecasts to actual results by rep, team, and method to spot patterns and gaps.
Segment your pipeline: separate enterprise deals from high-velocity deals—each needs its own sales forecasting method.
Use multiple forecasting models: blend historical forecasting, stage-based probabilities, and AI-driven forecasts to improve reliability.
Review and adjust forecasts weekly: make forecasting a routine part of the sales process not just a month-end task.
Incorporate deal-level risk: flag and discount stalled, single-threaded, or aging deals with longer-than-average sales cycles.
Invest in forecasting tools: Platforms like Forecastio automate complex calculations and help sales leaders make faster, data-driven decisions.
Summary
Sales forecasting in B2B is both an art and a science. From simple stage-based projections to advanced, AI-powered models, each sales forecasting method offers unique strengths. The key is selecting the right approach based on your sales motion, data maturity, and business goals.
Most importantly, treat sales forecasting as a dynamic, ongoing process—not just a one-time spreadsheet exercise. Regular updates, better inputs, and continuous learning will drive more accurate sales forecasts over time.
A good sales forecast doesn’t just predict future revenue—it shapes how you lead, plan, and scale your business with confidence.
Why Is Sales Forecasting Important?
Sales forecasting is the backbone of strategic decision-making in B2B companies. An accurate sales forecast empowers sales leaders and executives to predict future sales, allocate resources efficiently, and make confident, data-driven decisions. A reliable sales forecasting process is not just a reporting mechanism—it’s a strategic asset that drives future sales performance, helps you forecast sales with confidence, and fuels business growth.
When you create a sales forecast using reliable sales forecasting methods and historical sales data, you gain critical visibility into future revenue, enabling better planning across hiring, budgeting, and goal-setting. Companies that implement a structured forecasting process can avoid common pitfalls like over-hiring, under-investing, or missing sales targets due to unrealistic assumptions or inaccurate projections.
According to Gartner, companies that are able to forecast within 10% of actuals are more than twice as likely to experience consistent sales growth year over year. This highlights why sales forecasting is important—it gives organizations the clarity needed to navigate changing market conditions, anticipate market demand, and optimize their sales strategy.
Whether you use historical forecasting, sales pipeline insights, or intuitive sales forecasting, having a good sales forecast in place helps you stay aligned with business objectives and adjust to external factors that influence projected revenue. In short: better sales forecasts, better decisions—and better outcomes.
Why Does Sales Forecasting Fail?
Even though sales forecasting is important for strategic planning, many sales forecasts still miss the mark. The problem isn’t the idea of forecasting itself—it’s how the sales forecasting process is executed. Here are some of the most common reasons sales forecasts fail to deliver accurate, actionable insights:
Overreliance on intuition: Too often, sales reps rely on gut feelings instead of using data-driven sales forecasting methods. While intuitive forecasting may feel right, it rarely results in an accurate forecast without the support of solid historical data and sales performance metrics.
Stale or incomplete data: Outdated or missing sales data skews the forecast. Without clean, timely information from your sales pipeline, you can’t reliably predict future sales or estimate future sales revenue.
Poor CRM hygiene: If your sales team doesn’t maintain consistent deal stages or follow a clearly defined sales process, it becomes nearly impossible to forecast sales with precision. Sales forecasting tools can only work if the underlying sales data is trustworthy.
One-size-fits-all approach: Using the same forecasting method across different sales motions—like applying a sales cycle forecasting model built for enterprise sales to a transactional, high-velocity team leads to misaligned sales projections and inaccurate future performance estimates.
Lack of deal-level insights: Without factoring in deal-level risks—such as stalled opportunities, deals stuck in the sales cycle, or single-threaded conversations—the sales forecast becomes overly optimistic and unreliable. This is especially problematic when trying to meet tight sales targets or project future revenue.
In short, sales forecasting fails not because it’s an inherently flawed concept, but because of poor inputs, weak assumptions, and inconsistent execution. To create a sales forecast that drives decisions, it’s essential to choose the right sales forecasting method, ensure clean past sales data, and maintain a disciplined forecasting process that reflects your actual market conditions and sales strategy.
Sales Forecasting Examples
1. Forecasting by Opportunity Stage
This sales forecasting method uses your pipeline’s opportunity stages to generate a sales forecast. Each stage in the sales cycle—such as Discovery, Demo, Proposal, and Contract—is assigned a probability that reflects its historical conversion rate to closed-won deals. These probabilities are then applied to open deals to forecast sales and estimate future revenue.
This method is one of the most common sales forecast examples, especially in companies with structured pipelines and reliable sales data.
How to Calculate Stage Probabilities (Based on Historical Sales Data)
To assign probabilities to each stage, analyze your historical sales data to see what percentage of deals that entered a stage were ultimately won.
Stage | Deals Entered | Deals Won | Conversion Rate |
Discovery | 100 | 20 | 20% |
Demo | 80 | 32 | 40% |
Proposal | 50 | 30 | 60% |
Contract | 30 | 24 | 80% |
Formula: Probability = Deals Won / Deals Entered
Forecasting Example Using Stage Probabilities
Let’s apply the stage probabilities to active deals in your pipeline. This gives you a weighted sales projection—a realistic view of your future sales revenue based on where each deal currently stands.
Open Deals:
Deal | Stage | Deal Value | Stage Probability | Weighted Forecast |
Deal A | Proposal | $20,000 | 60% | $12,000 |
Deal B | Demo | $10,000 | 40% | $4,000 |
Deal C | Discovery | $15,000 | 20% | $3,000 |
Total Forecast = $12,000 + $4,000 + $3,000 = $19,000
This method is ideal when you have a consistent sales process, defined sales pipeline stages, and enough historical data to calculate meaningful stage conversion rates.
Try Forecastio to see how automatically calculated stage probabilities can boost your sales forecasting accuracy.
Pros:
Simple and intuitive
Doesn’t require advanced sales forecasting software
Works well when historical sales performance is consistent
Cons:
May overlook deal quality or sales rep behavior
Doesn’t account for stalled or high-risk deals
Can be skewed if past data is unreliable or incomplete
When to Use
Use this forecasting method when you have:
Clearly defined pipeline stages
Reliable historical sales data
A relatively stable sales cycle
This is a classic sales forecast example that helps sales leaders and sales managers build their first structured forecast based on pipeline health and historical performance. It’s also a useful starting point before moving to more advanced methods like AI-driven sales forecasting or sales cycle forecasting.
2. Advanced Opportunity Stage Forecasting
This advanced sales forecasting method builds upon traditional opportunity stage forecasting, but adds a critical adjustment: the time a deal spends in each stage. Deals that are stuck, stalled, or spend significantly more time than average in a stage have their stage probabilities discounted—making the sales forecast more grounded in reality.
It’s a powerful way to generate more accurate sales forecasts, especially in environments where deals often get delayed or stuck due to external factors, sales cycle length, or customer behavior.
How Probability Discounts Work for Stalled Deals
To make this forecasting process more realistic, you compare how long each deal has been in its current stage to the average sales cycle length for that stage. If a deal has been sitting in a stage for longer than average, you apply a discount to its probability.
Example: Adjusting Probabilities Based on Time-in-Stage
Let’s assume your historical data shows that the average time spent in each stage looks like this:
Stage | Avg Time in Stage |
Discovery | 5 days |
Demo | 7 days |
Proposal | 10 days |
Now let’s say:
Deal A has been in the Proposal stage for 20 days (2× the average).
Deal B has been in the Demo stage for 7 days (on schedule).
You can apply a simple discount rule. For example, reducing the probability by 50% if the time spent on stage exceeds 150% of the average.
Forecasting Example with Adjusted Probabilities
Deal | Stage | Deal Value | Standard Probability | Time in Stage | Adjusted Probability | Weighted Forecast |
Deal A | Proposal | $25,000 | 60% | 20 days | 30% (discounted) | $7,500 |
Deal B | Demo | $10,000 | 40% | 7 days | 40% (on track) | $4,000 |
Total Forecast = $7,500 + $4,000 = $11,500
This more advanced sales forecast example gives sales managers and sales leaders a clearer view of at-risk deals and helps prevent inflated sales projections caused by pipeline stagnation.
Pros:
More accurate than standard pipeline forecasting
Accounts for deal aging and pipeline health
Helps identify stuck deals that need attention
Cons:
Requires consistent CRM hygiene
Not easy to manage manually—benefits from sales forecasting tools
When to Use
Use this forecasting method when:
You already use pipeline stage forecasting
You want to reduce the risk of overforecasting
Your sales cycle includes deals that frequently stall
You aim to predict sales with greater precision by factoring in sales velocity
3. Historical Forecasting
Historical forecasting uses past sales data from previous time periods—typically quarters or years—to predict future sales. This sales forecasting method assumes that sales performance follows consistent patterns over time, making it especially useful for companies with clear seasonality, long sales cycles, or repeatable motions.
This is one of the most straightforward sales forecast examples and is often used as a baseline when other inputs, such as pipeline data, are limited or unreliable.
How It Works
Using historical sales data (e.g., bookings, closed-won revenue, or total sales), you can calculate growth trends and apply them to estimate future sales. A simple way to do this is to calculate a year-over-year growth rate and apply it to the most recent comparable period.
Forecasting Example Based on Historical Performance
Let’s say you’re forecasting for Q2 2024 using previous quarterly performance:
Period | Bookings | Growth Rate |
Q2 2023 | $100,000 | - |
Q2 2024 | $120,000 | +20% |
Q2 2025 | $144,000 | +20% (assumed) |
This sales forecast example is based purely on past sales trends. It assumes that your sales strategy, sales team, market demand, and sales cycle haven’t changed significantly year over year.
Pros:
Extremely easy to implement
Requires only past sales data
Useful for steady, repeatable businesses with consistent future sales revenue
Cons:
Ignores the current sales pipeline and sales reps' activity
Doesn’t reflect external factors, new competitors, or market trends
Risky if your business is scaling rapidly or experiencing disruption
When to Use
Use historical forecasting when:
Your business has steady sales cycles
Seasonality and trends are reliable
You need a fast, high-level forecast based on historical data
You want a baseline forecast before applying more advanced sales forecasting methods
Although this method doesn’t use sales forecasting tools or pipeline forecasting, it still plays a role in generating projected revenue—especially when comparing against more dynamic models. When combined with other approaches, it can help validate the accuracy of more complex sales forecasts.
4. Deal Probabilities Using Machine Learning
This advanced sales forecasting method uses machine learning (ML) to calculate win probabilities for each deal based on dozens (or even hundreds) of variables. Unlike traditional pipeline forecasting, which relies on static probabilities tied to stages, ML models analyze complex patterns in historical data to predict future sales more accurately.
By using sales forecasting tools with embedded ML capabilities, companies can dynamically adjust forecasts in real-time based on actual deal behavior, improving the odds of creating an accurate sales forecast.
ML Models Commonly Used in Sales Forecasting
Model Type | Use Case |
Logistic Regression | Predicts win/loss probability based on weighted deal features |
Random Forest | Analyzes deal attributes via decision trees to classify win/loss outcomes |
Gradient Boosting | Builds complex, layered models for more precise predictions |
Neural Networks | Ideal for large datasets with non-linear relationships |
Each of these models helps sales teams move beyond guesswork and static stage-based probabilities, toward more predictive sales forecasts rooted in behavioral and contextual data.
Example: Attributes Considered in ML Forecasting
ML models calculate deal-level win probabilities using a combination of deal, company, and rep-level features. Here’s a list of typical attributes:
Attribute | Description |
Stage | Current pipeline stage |
Time in Stage | Number of days deal has been in current stage |
Deal Age | Total time deal has been open |
Deal Amount | Monetary value of the opportunity |
Industry | Industry of the prospect/customer |
Company size | Size of the company based on employees or revenue |
Customer Engagement | Email opens, meeting attendance, replies, etc. |
Sales Rep Activities | Calls made, emails sent, follow-ups scheduled |
Previous Interactions | History of past deals with the account or contact |
Lead Source | Origin of the lead (inbound, outbound, referral, etc.) |
Competitors Involved | Whether other vendors are active in the deal |
These variables are fed into a model that’s trained on past sales data to predict future sales performance—providing a probability score that evolves over time.
Forecasting Example Using ML Probabilities
Let’s assume an ML model has already assigned win probabilities to open deals based on their attributes:
Deal | Value | ML Win Probability | Weighted Forecast |
Deal A | $50,000 | 35% | $17,500 |
Deal B | $30,000 | 70% | $21,000 |
Deal C | $40,000 | 15% | $6,000 |
Total Forecast = $17,500 + $21,000 + $6,000 = $44,500
This sales forecast example offers a far more nuanced and responsive estimate of future sales revenue than traditional methods.
Book a demo to see how Forecastio uses time-series analysis to reduce human bias and deliver highly accurate sales forecasts.
Pros:
Produces highly accurate forecasts
Adapts to changing sales performance and market conditions
Detects hidden patterns missed by manual analysis
Cons:
Requires large amounts of clean sales data
Implementation needs technical resources or a modern forecasting platform
Can be a “black box” for sales managers without data expertise
When to Use
Use ML-based sales forecasting when:
Your CRM is well-maintained and rich in historical sales data
You have a large volume of deals for training the model
Your organization is ready to invest in sales forecasting software or internal data science resources
You want to improve forecasting accuracy and reduce human bias
This is one of the most powerful and scalable sales forecast examples, giving sales leaders and revenue operations teams a reliable way to predict future sales with greater precision and confidence.
5. Length of Sales Cycle Forecasting
This sales forecasting method uses the length of the sales cycle to estimate a deal’s likelihood to close. Instead of looking at pipeline stages or historical forecasting alone, it evaluates where each deal is in relation to the average sales cycle length. The further along a deal is, the higher its predicted probability of closing—assuming a consistent sales process.
This model is particularly useful for doing time-based pipeline health checks and forecasting future revenue in high-velocity sales environments.
How It Works
Using past sales data, determine your average sales cycle length (e.g., 60 days). Then calculate the percentage of that cycle a current deal has completed.
Formula: Probability = Days in Sales Cycle / Average Sales Cycle Length
So if a deal has been open for 30 days and your average sales cycle is 60 days, it has completed 50% of the journey—translating to a 50% likelihood, assuming no other influencing factors.
This is a simple but effective sales forecast example for companies with high deal volume and minimal variability across opportunities.
Forecasting Example Based on Sales Cycle Progress
Let’s assume your historical data shows an average sales cycle of 60 days. You’re evaluating two active deals:
Deal | Deal Value | Days in Cycle | Avg Sales Cycle | Probability | Weighted Forecast |
Deal A | $40,000 | 30 days | 60 days | 50% | $20,000 |
Deal B | $20,000 | 15 days | 60 days | 25% | $5,000 |
Total Forecast = $20,000 + $5,000 = $25,000
This method helps sales managers and sales reps quickly forecast sales without requiring deep insights into customer engagement, rep activity, or deal quality.
Pros:
Easy to implement with past sales trends
Works well in high-volume pipelines with similar deal profiles
Ideal for quick health checks on pipeline progress and future sales
Cons:
Doesn’t account for deal quality, external factors, or sales rep performance
May mislead if sales cycle duration varies widely across deals
Not suitable for complex or long-cycle enterprise sales
When to Use
Use length of sales cycle forecasting when:
You have high deal volume and consistent sales cycle durations
Your team follows a uniform sales process
You want to estimate future sales quickly and simply
You’re doing top-level sales projections to guide resource allocation
This is a time-driven sales forecast example that’s especially effective in transactional sales models, giving sales leaders a simple, scalable approach to tracking progress and predicting future sales performance based on momentum.
Which Sales Forecasting Method to Choose?
There’s no universal approach to building an accurate sales forecast—the best sales forecasting method depends on your business model, team structure, and data maturity. In other words, sales forecasting isn’t one-size-fits-all. To forecast sales effectively and support decision-making, you need to choose the method that aligns best with your sales environment and available insights.
Here are the key factors to consider when deciding how to create a sales forecast:
✅ 1. Your Sales Motion
Is your team closing high-volume, fast-moving deals or navigating long, complex sales cycles?
High-velocity sales: Consider stage-based or length-of-sales-cycle forecasting. These models work well for quick, transactional sales processes where deal movement is frequent and predictable.
Enterprise sales: Leverage machine learning forecasting or advanced pipeline stage forecasting that accounts for multiple variables like deal risk, time in stage, and sales rep activity.
✅ 2. Data Availability and Quality
How much historical sales data do you have, and how reliable is it?
If your CRM contains consistent, clean past sales data, you can use more sophisticated sales forecasting tools that analyze market trends, customer behavior, and rep performance to produce more accurate sales forecasts.
If your data is limited or inconsistent, start simple with historical forecasting or stage-based models.
✅ 3. Team Size and CRM Discipline
Do you have a small sales team just getting started, or a mature organization with RevOps support?
Small team, short sales cycle: Start with classic pipeline forecasting using opportunity stage probabilities. It’s easy to implement and can help you calculate a sales forecast with minimal overhead.
Larger team with consistent CRM hygiene: Move toward ML-based forecasting or hybrid models that incorporate multiple data points to predict future sales more reliably.
Growing or hybrid sales org: Combine multiple sales forecasting methods—like weighting historical results alongside current sales pipeline trends and ML insights—for a blended and more accurate view of future revenue.
How to Improve Sales Forecasting Accuracy
Improving sales forecasting accuracy doesn’t require overhauling your entire process but it does demand consistency, clean data, and the right methods. Here’s how to create more accurate sales forecasts step by step:
Audit your CRM hygiene: standardize fields, ensure pipeline stages are clearly defined, and keep sales data updated.
Analyze past forecast accuracy: compare sales forecasts to actual results by rep, team, and method to spot patterns and gaps.
Segment your pipeline: separate enterprise deals from high-velocity deals—each needs its own sales forecasting method.
Use multiple forecasting models: blend historical forecasting, stage-based probabilities, and AI-driven forecasts to improve reliability.
Review and adjust forecasts weekly: make forecasting a routine part of the sales process not just a month-end task.
Incorporate deal-level risk: flag and discount stalled, single-threaded, or aging deals with longer-than-average sales cycles.
Invest in forecasting tools: Platforms like Forecastio automate complex calculations and help sales leaders make faster, data-driven decisions.
Summary
Sales forecasting in B2B is both an art and a science. From simple stage-based projections to advanced, AI-powered models, each sales forecasting method offers unique strengths. The key is selecting the right approach based on your sales motion, data maturity, and business goals.
Most importantly, treat sales forecasting as a dynamic, ongoing process—not just a one-time spreadsheet exercise. Regular updates, better inputs, and continuous learning will drive more accurate sales forecasts over time.
A good sales forecast doesn’t just predict future revenue—it shapes how you lead, plan, and scale your business with confidence.
Share:
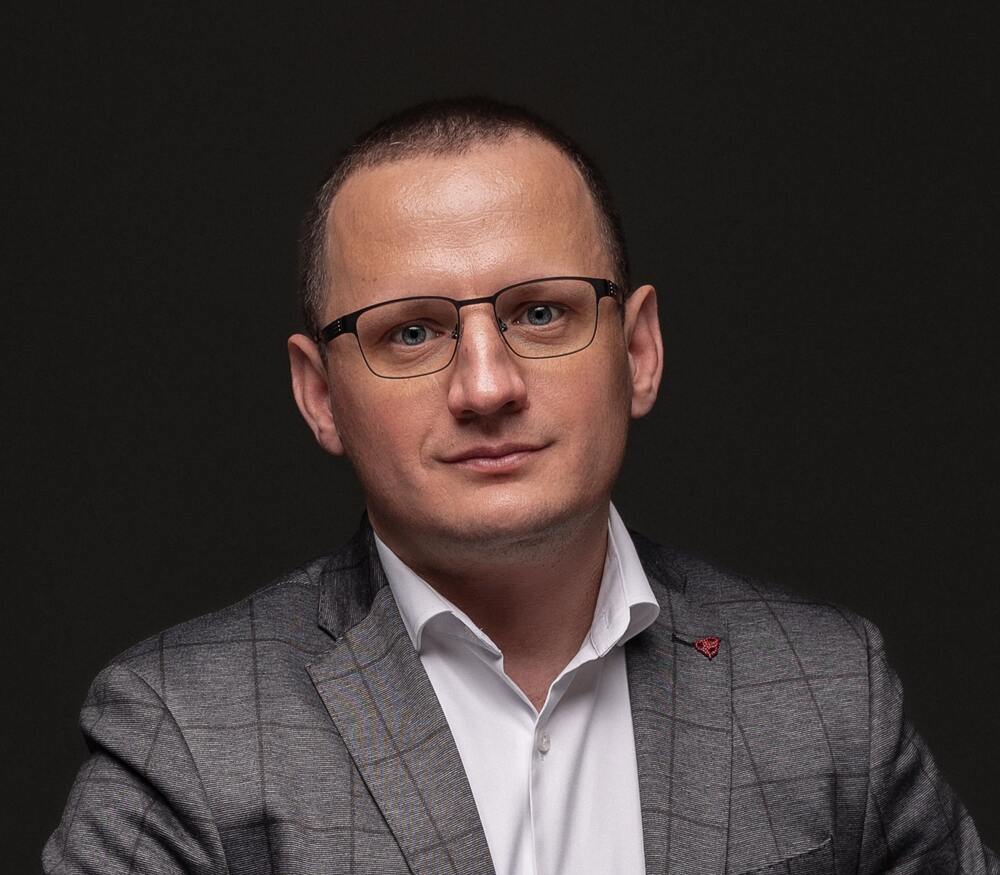
Alex is the CEO at Forecastio, bringing over 15 years of experience as a seasoned B2B sales expert and leader in the tech industry. His expertise lies in streamlining sales operations, developing robust go-to-market strategies, enhancing sales planning and forecasting, and refining sales processes.
Alex is the CEO at Forecastio, bringing over 15 years of experience as a seasoned B2B sales expert and leader in the tech industry. His expertise lies in streamlining sales operations, developing robust go-to-market strategies, enhancing sales planning and forecasting, and refining sales processes.
Related articles
Sales Leadership
Apr 3, 2025
10 min
Sales Leadership
Apr 3, 2025
10 min
Sales Forecasting
Mar 28, 2025
10 min
Sales Forecasting
Mar 28, 2025
10 min
Sales Strategies
Mar 24, 2025
9 min
Sales Strategies
Mar 24, 2025
9 min
Sales Leadership
Apr 3, 2025
10 min
Sales Forecasting
Mar 28, 2025
10 min
Sales Leadership
Apr 3, 2025
10 min
Sales Forecasting
Mar 28, 2025
10 min
Sales Planning
Sales Forecasting
Sales Performance Insights
Sales Planning
Sales Forecasting
Sales Performance Insights
Sales Planning
Sales Forecasting
Sales Performance Insights
© 2025 Forecastio, All rights reserved.
Sales Planning
Sales Forecasting
Sales Performance Insights
Sales Planning
Sales Forecasting
Sales Performance Insights
Sales Planning
Sales Forecasting
Sales Performance Insights
© 2025 Forecastio, All rights reserved.
Sales Planning
Sales Forecasting
Sales Performance Insights
Sales Planning
Sales Forecasting
Sales Performance Insights
Sales Planning
Sales Forecasting
Sales Performance Insights
© 2025 Forecastio, All rights reserved.
Sales Planning
Sales Forecasting
Sales Performance Insights
Sales Planning
Sales Forecasting
Sales Performance Insights
Sales Planning
Sales Forecasting
Sales Performance Insights
© 2025 Forecastio, All rights reserved.