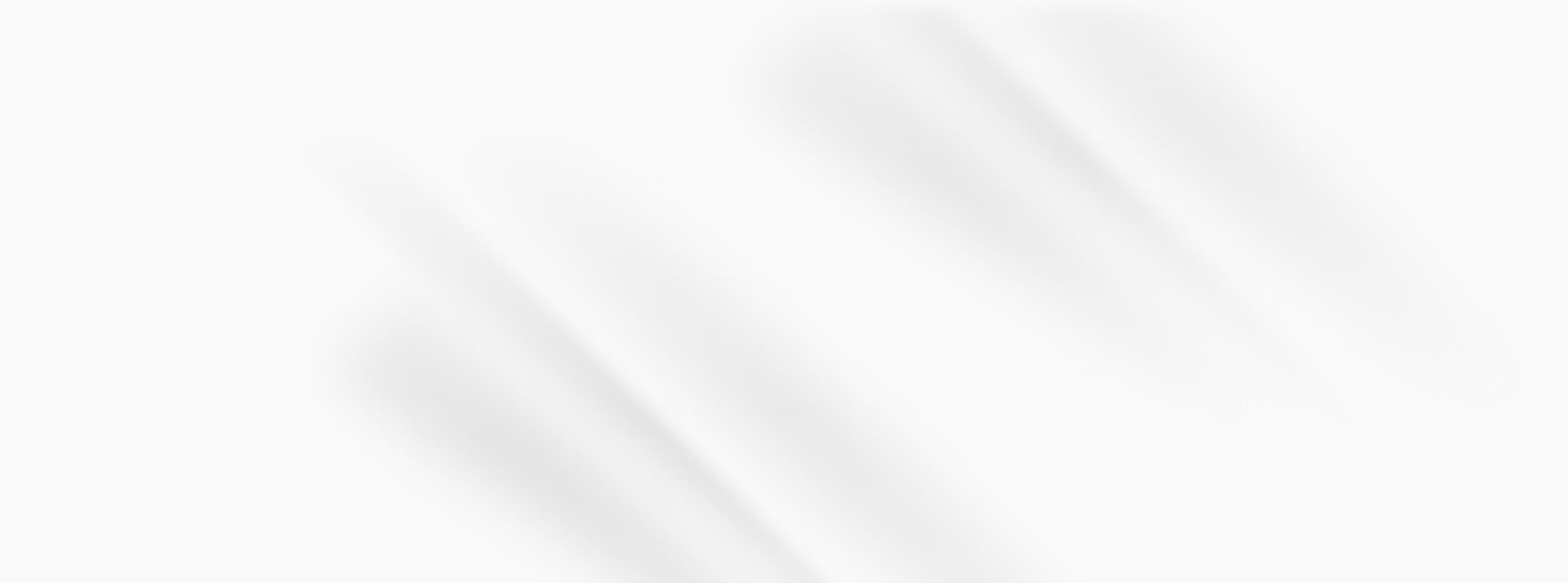
Overcoming Bad Sales Data: How to Forecast Sales Accurately
Feb 17, 2025
Feb 17, 2025
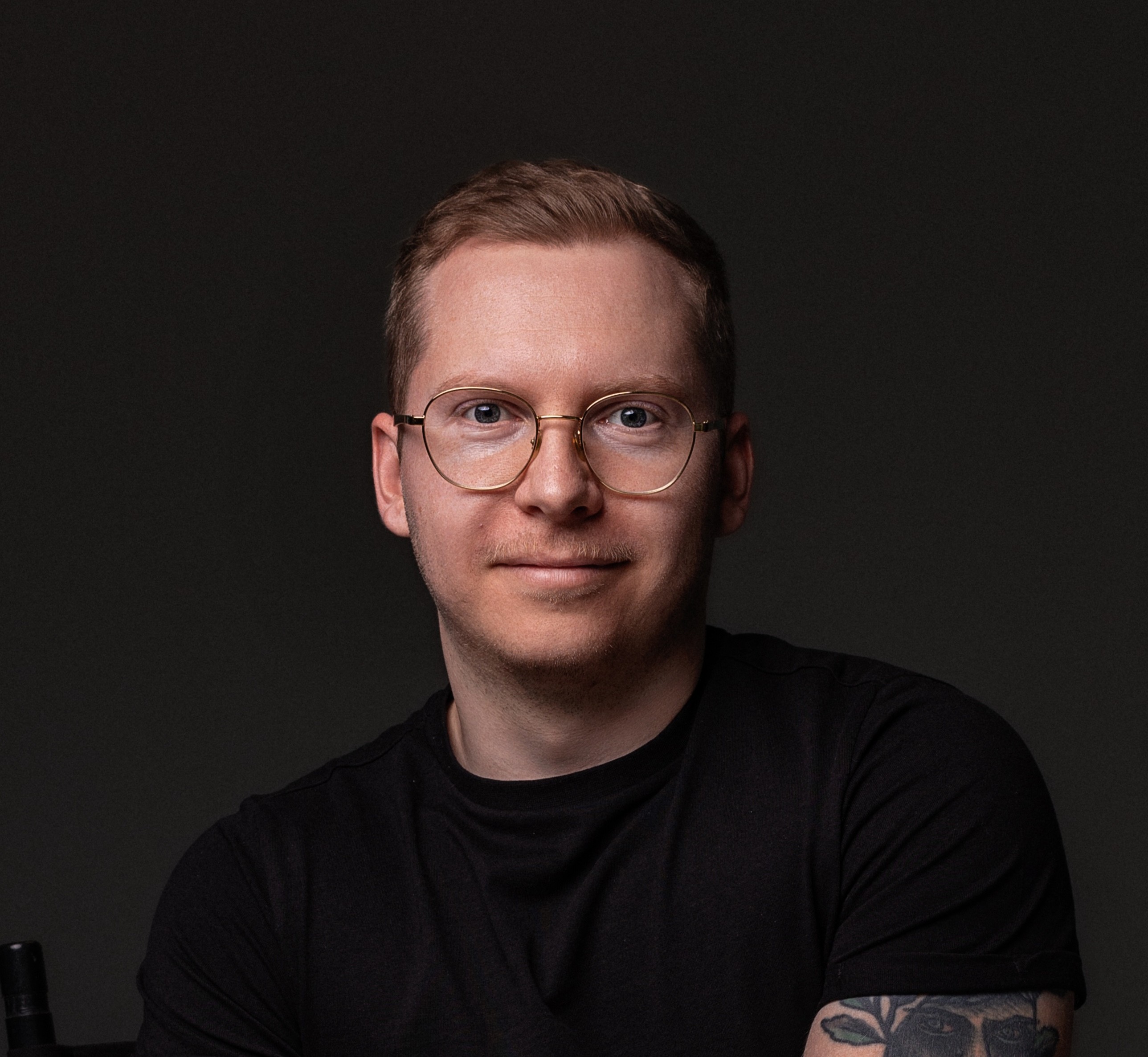
Dmytro Chervonyi
CMO at Forecastio
Last updated
Feb 17, 2025
Reading time
13 min
Share:
Share
Table of Contents
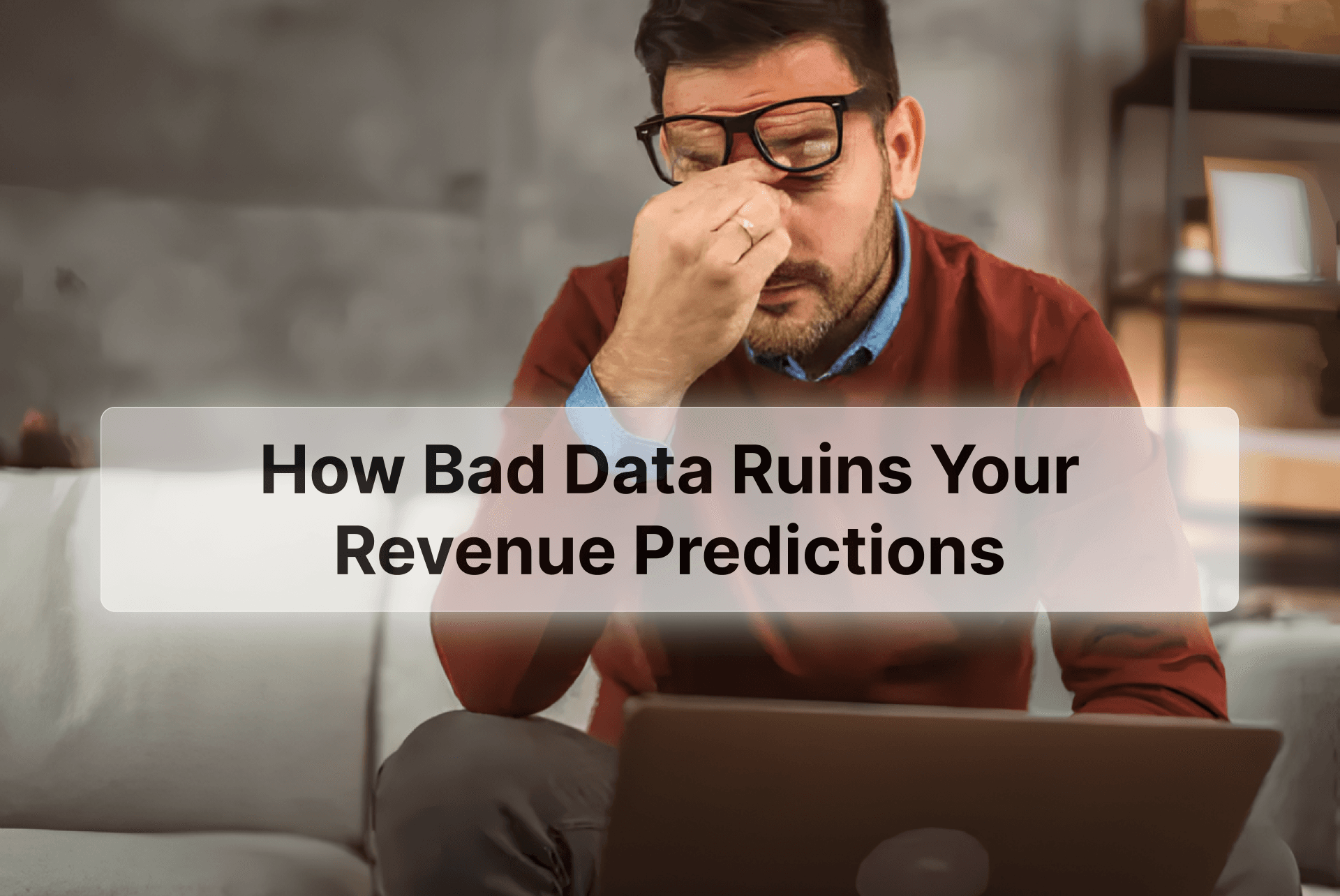
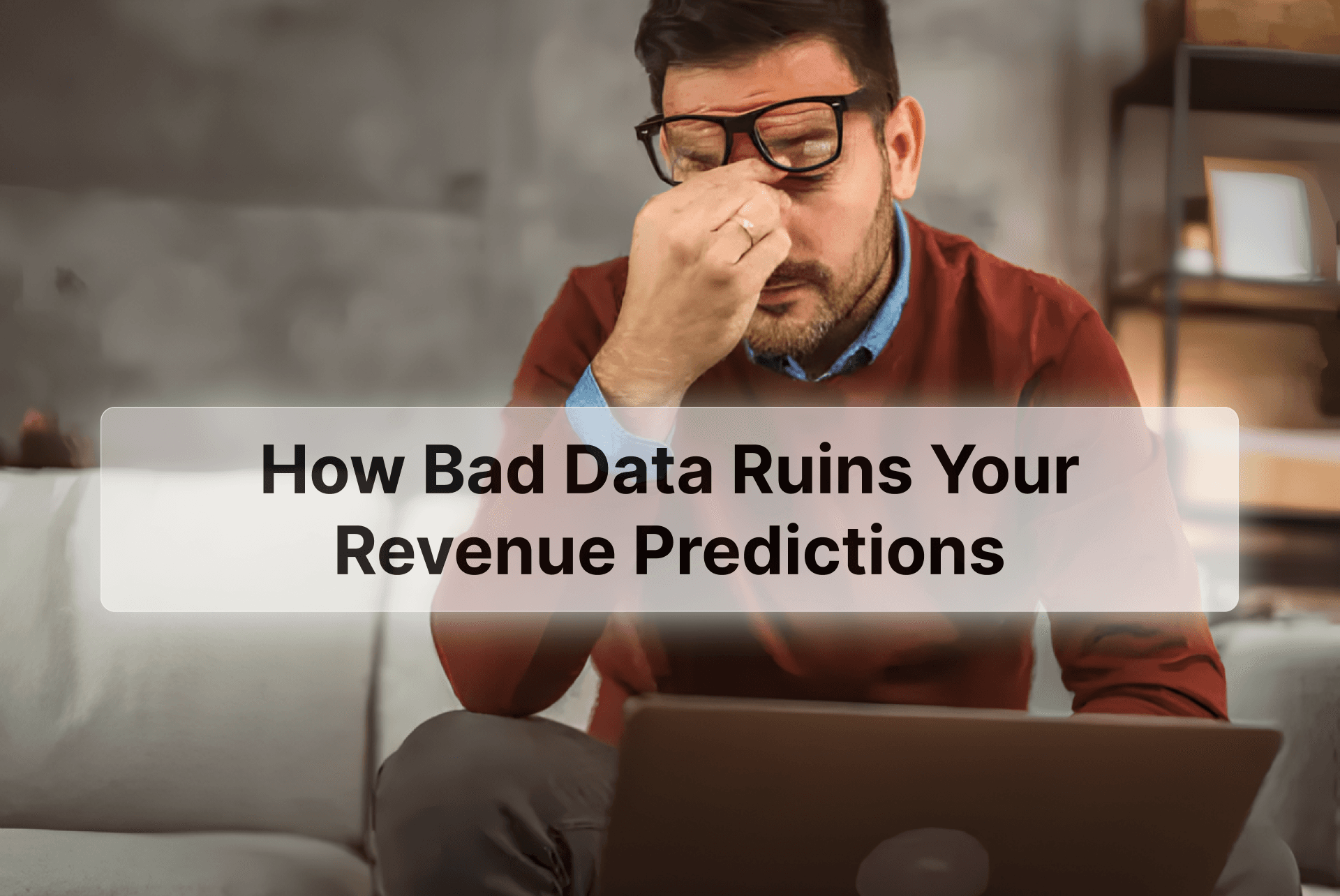
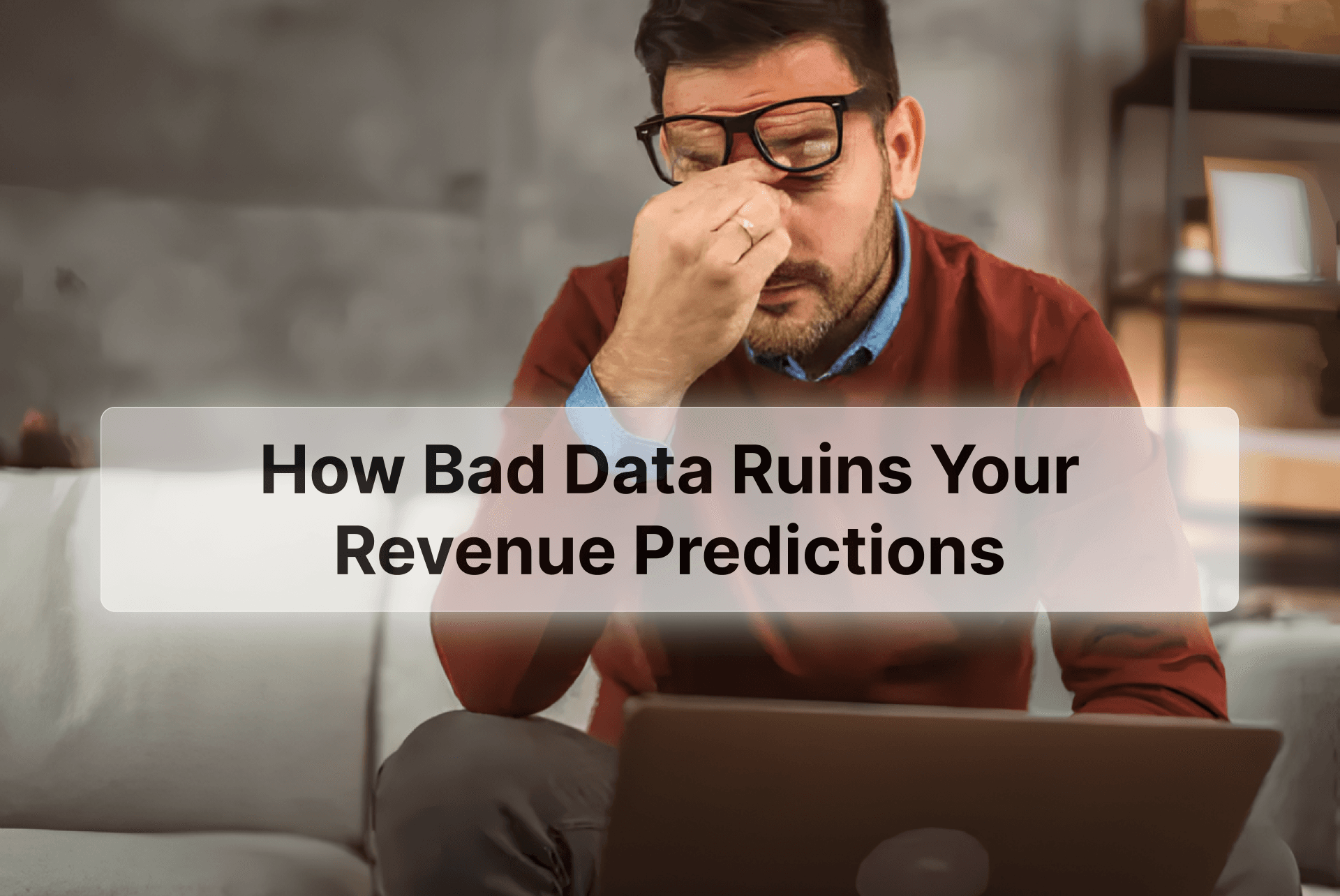
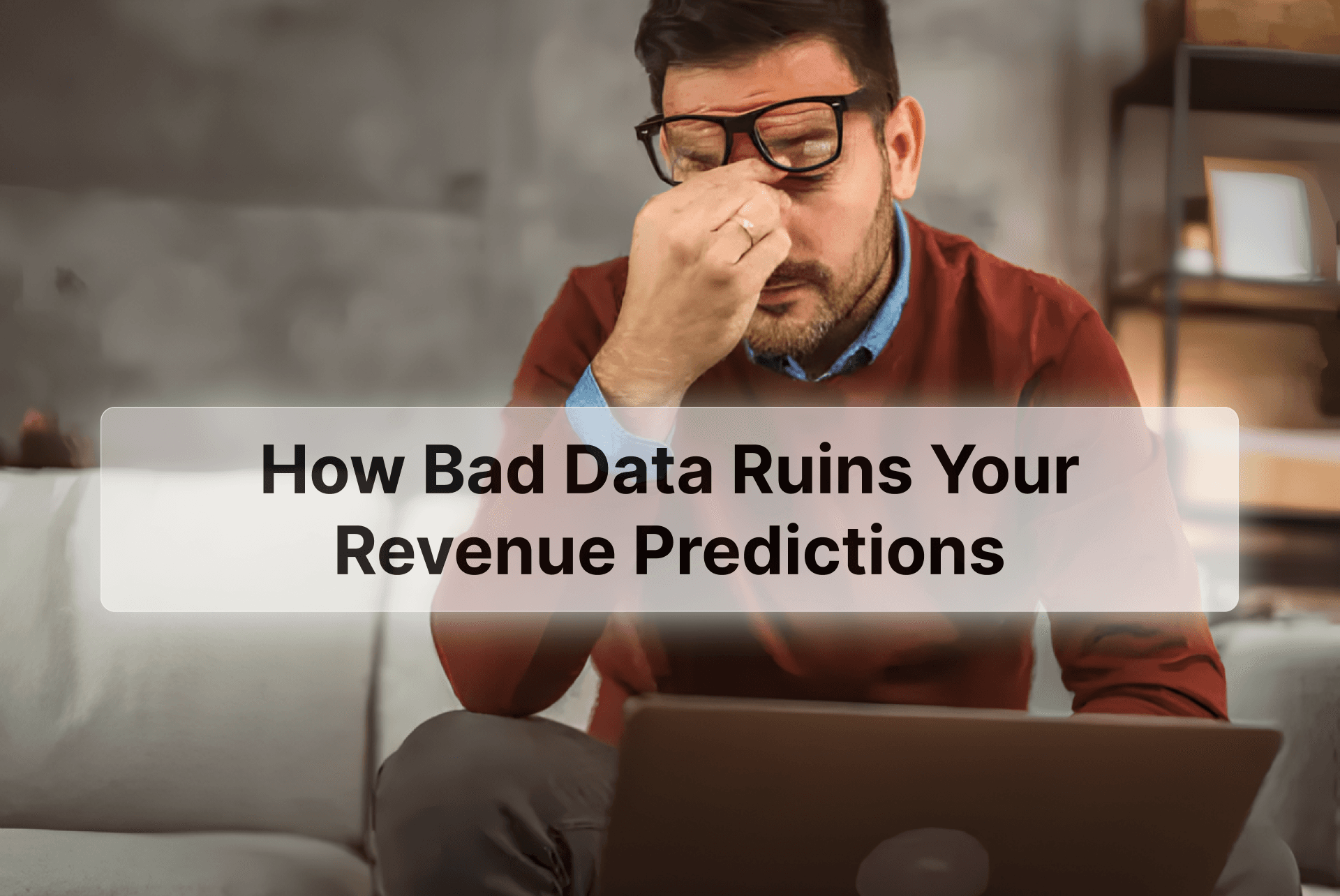
Quick Take
Quick Take
Bad data quality costs U.S. businesses $3.1 trillion yearly.
Five common data problems wreck sales forecasts:
Deals without amounts
Missing close dates
Constantly slipping deals
Stale opportunities
Deals without activities
Fix these by making data quality part of performance reviews, automating data checks, and cleaning your CRM regularly.
When reps skip data entry to focus on closing, it creates bad forecasts that hurt the whole company.
Teams that fix their data quality see 20% more accurate forecasts in the first quarter.
Give reps clear guidelines, use automation to catch errors, and review data weekly.
Clean data means faster closes and better decisions.
Bad data quality costs U.S. businesses $3.1 trillion yearly.
Five common data problems wreck sales forecasts:
Deals without amounts
Missing close dates
Constantly slipping deals
Stale opportunities
Deals without activities
Fix these by making data quality part of performance reviews, automating data checks, and cleaning your CRM regularly.
When reps skip data entry to focus on closing, it creates bad forecasts that hurt the whole company.
Teams that fix their data quality see 20% more accurate forecasts in the first quarter.
Give reps clear guidelines, use automation to catch errors, and review data weekly.
Clean data means faster closes and better decisions.
Sales forecasting is one of those tasks that sales teams perform regularly, but it's so much more important than that. Forecasting is the backbone of your revenue recognition, and it's how executives make strategic decisions about the direction of the company.
But what happens when your forecast is wrong? It's often because your data is bad. And bad data leads to bad forecasts, missed quotas, and wasted time and money.
In this article, we'll explore how accurate data impacts your forecasts, how bad data holds you back, and what you can do to fix this common sales forecasting problem.
What is sales forecasting?
Sales forecasting is the process of estimating future sales revenue over a set period. It's an art and a science, using historical sales data, market trends, and other factors to make an educated guess about future sales. Accurate data is crucial for retaining existing customers and earning their loyalty. Without it, you're sailing a ship without a map or compass.
Using statistical models, machine learning algorithms, and expert judgment, companies can predict the probability of future sales and revenue. This is critical for making decisions about production, inventory, pricing, and resource allocation. Sales forecasting is the foundation of strategic planning, and it guides your company toward success.
Why sales forecasting matters
Why is sales forecasting so important? It's the foundation of all business decision-making. Your sales and marketing teams need accurate data to succeed, so precise forecasts are critical. Accurate sales forecasts give you the ability to:
Set realistic sales goals and quotas: Knowing what to shoot for keeps reps motivated and focused.
Manage resources: From staff to inventory, knowing what to expect in terms of future sales helps you plan.
Make informed product and pricing decisions: If you know what's selling and what's not, you can make strategic decisions about product lines and pricing.
Plan for inventory and production: If you know what to expect in terms of future sales, you can plan for inventory and production.
Determine revenue recognition: Accurate sales forecasts give you the ability to recognize revenue and make financial projections.
Guide strategic business decisions: Sales forecasting is the backbone of strategic planning, and it guides your company toward success.
Manage cash flow and budgeting: Knowing what to expect in terms of revenue helps you plan for cash flow and budgeting.
Plan for staffing and training: Knowing if you'll experience sales growth helps you hire and train the right people at the right time.
Determine opportunities for growth and expansion: Trends and patterns can reveal new markets or products.
Mitigate risks and uncertainties: Knowing what to expect helps you prepare for downturns.
In short, sales forecasting isn't a nicety; it's a necessity if you want your business to succeed.
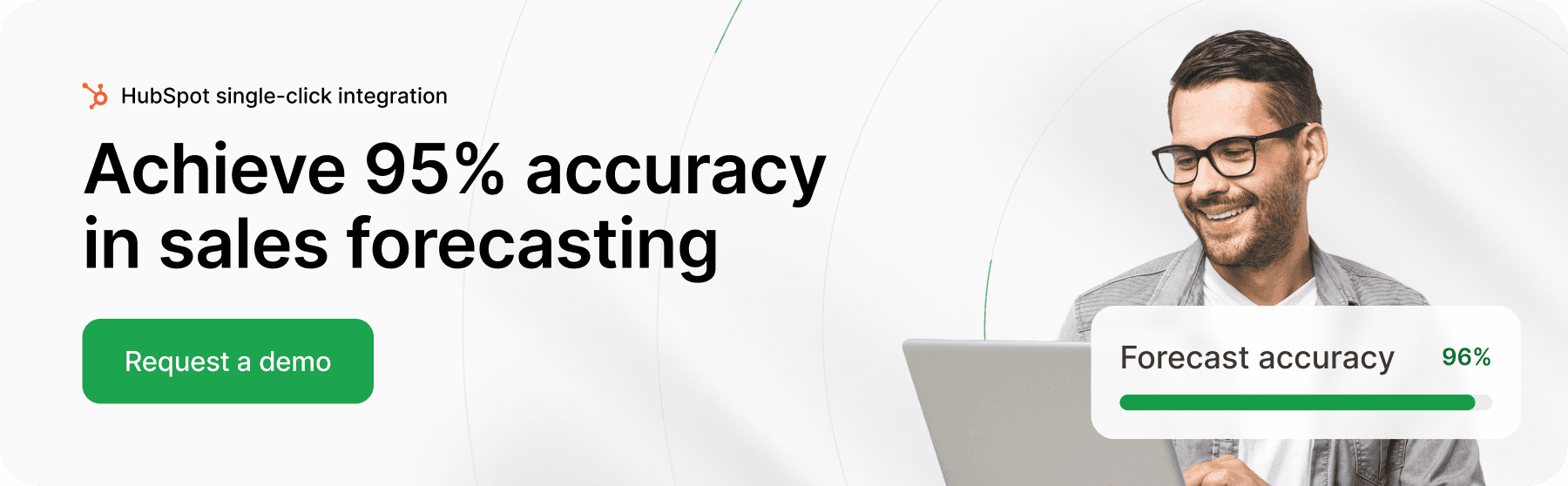
Sales forecasting process overview
The sales forecasting process is a methodology that outlines the steps you should take to forecast sales. Here's an overview of the process:
Data collection: This is where you gather historical sales data, market trends, and other relevant information. This is the fuel for your forecast. It's critical to address duplicate data at this stage to ensure data quality and prevent garbage-in, and garbage-out analysis.
Data analysis: Once you have your data, you need to analyze it to discover trends, patterns, and correlations. This is where you'll learn what drives sales.
Model selection: Select a forecasting model based on your data and business needs. This could be a simple moving average or a complex machine-learning model.
Forecasting: Use your model to forecast sales. This is where the magic happens, and your data becomes a valuable forecast.
Review and revision: Finally, you need to review and revise your forecast regularly. Sales forecasting isn't a one-and-done task; it's an ongoing process.
By following these steps, you can create a solid sales forecast that guides your strategy and drives growth.
The illusion of accuracy: The data quality crisis
The end of the quarter. Your sales team has worked tirelessly, and your CRM is full of numbers. Your forecast looks great. You present it to the board, and at the 11th hour, deals fall through or come in from left field. Sound familiar?
You're not alone. Many sales leaders are tempted by the wealth of data at their fingertips and assume quality when they see quantity.
But the truth is that in sales forecasting, bad data – aka dirty data – is often worse than no data at all. Accurate sales forecasting is critical for business planning and resource allocation, and overcoming common sales forecasting challenges is key to success.
The effects of poor data quality go far beyond inaccurate forecasts. It's a silent killer that erodes trust, misallocates resources and can impact your company's valuation. According to IBM, poor data quality costs the U.S. economy approximately $3.1 trillion each year. For sales teams, it means missed opportunities, wasted time and money, and a cycle of chasing instead of strategizing.
The five horsemen of the data apocalypse
Let's talk about the top five data quality issues that impact your sales forecasts and revenue recognition. We call them the "Five Horsemen of the Data Apocalypse" – dramatic, we know, but they're apocalyptic for your revenue recognition.
One of the biggest problems is simply poor-quality data. We're talking about bad data, but it goes by many names: sloppy data, poor data, lousy data. Whatever you call it, it's a serious problem that can severely impact operational effectiveness in B2B sales and marketing.
1. The ghost deal: Opportunities without amounts
How can you forecast your expenses without knowing how much money you'll make? Ridiculous, right? Yet many sales pipelines are haunted by these "ghost deals" – opportunities that are in the system, but lack one critical detail: the deal amount.
These ethereal deals skew your entire forecast. They could be $10,000 or $100,000 deals, but without an amount, you can't tell. As a result, sales leaders are forced to ignore these opportunities entirely (potentially leaving real revenue on the table) or make educated guesses about the value (introducing huge inaccuracies into the forecast). To forecast future sales accurately, you need detailed records and advanced forecasting methods.
2. The timeless wonder: Deals without close dates
In sales forecasting, timing is everything. A deal that's expected to close this quarter is much different from one that's expected to close next year. Accurate sales forecasting is all about having good data to create solid sales projections. Enter the "Timeless Wonders" – opportunities that are missing a critical piece of information: a close date.
These dateless deals can't be used to create time-based forecasts. They pad your pipeline and can skew your short-term or long-term forecasts. They often indicate a lack of qualification or next steps, exposing deeper issues in your sales process.
3. Walking Dead deals: The deals that constantly slip
We've all been there: a deal that's slipping from quarter to quarter. These "Eternal Sliders" are the bane of accurate forecasting. They make your pipeline look healthy, but your forecast is another story altogether.
Slipping deals often indicate a deeper problem: perhaps your value proposition isn't strong enough, decision-makers aren't engaged, or your timeline isn't aligned with the customer's. Historical sales data can help you identify these sliders, and it's critical for both accurate forecasting and fixing your sales process.
4. The fossil record: Deals that won't budge
Every pipeline has them: opportunities that are fossilized in your pipeline after months (or years). These "Fossil Records" are often the leftovers of overzealous sales reps or changes in the buyer's situation that weren't updated in the system.
Stale deals pad your pipeline and skew your sales metrics, such as average deal size and sales cycle length. They can also mask real issues in your sales process, like poor follow-up or inadequate qualification criteria.
Most importantly, they give a false impression of future revenue potential, introducing huge inaccuracies into your forecast and resource allocation. The right sales forecasting methods are critical – not all methods are right for every business.
5. The lone wolf: Deals without tasks or activities
Sales is an ongoing process. An opportunity without tasks or activities is a plant without water – it's not going anywhere. Using sales forecasting software can help you forecast more accurately and efficiently by leveraging historical data and market trends.
It also provides actionable intelligence and real-time visibility to inform your sales strategies. These "Lone Wolves" are often ignored in your forecast, introducing blind spots into your projections.
A lack of tasks or activities usually indicates the deal isn't being actively pursued. Perhaps the sales rep is overwhelmed, the opportunity was deprioritized, or CRM hygiene is poor. Whatever the reason, these leads introduce huge uncertainty into your forecast, and they're likely wasting away in your pipeline.
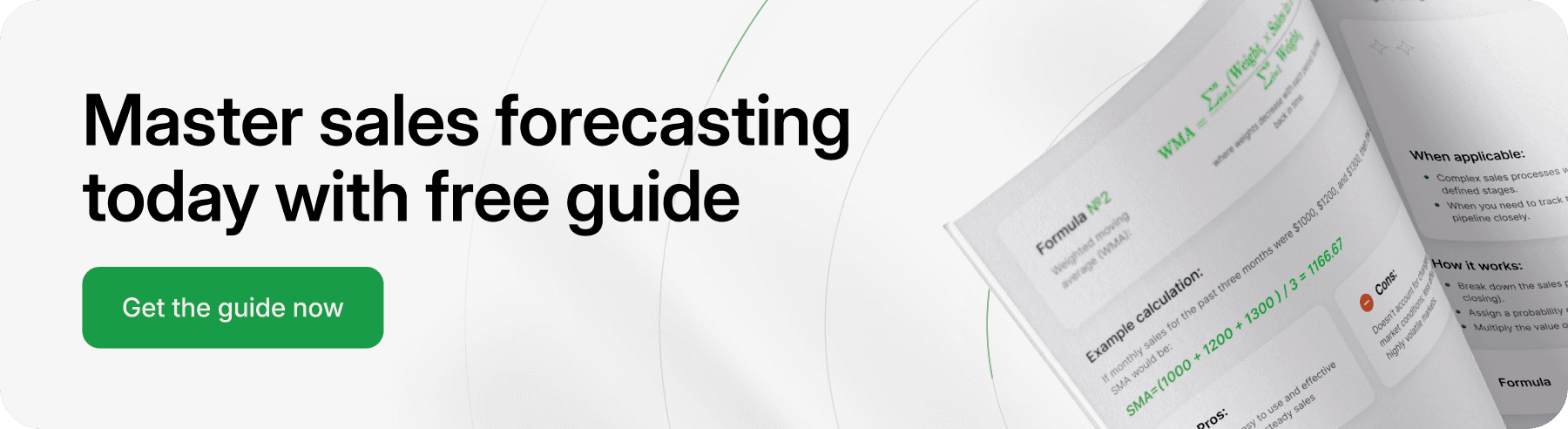
Sales forecast factors
Internal factors: Hires and fires, policy changes, territory shifts
Internal factors can impact sales forecasts in big and small ways. Let's talk about some of those internal factors:
Hires and fires: Changes to the sales team can ripple through sales and forecasting. Bringing in new reps can give you a sales boost while losing a top performer can be hard to recover from.
Policy changes: Changes to company policy, such as pricing strategy or commission structure, can impact sales directly. Aggressive pricing can drive more sales while changing the commission structure can motivate or demotivate your sales team.
Territory shifts: Reassigning territories or changing sales assignments can break relationships and impact sales. A well-planned territory reassignment can help you cover more ground and drive more sales, but a poorly executed change can confuse customers and cost you deals.
These internal factors are impacted by:
Sales team performance and morale: A motivated and supported sales team will hit quota and maintain good data quality.
Training and development programs: Ongoing training and development help sales reps fine-tune their skills and improve forecasting accuracy.
Sales process and methodology: A defined sales process ensures consistency and reliability in data collection and forecasting.
Compensation and incentive structures: Aligning incentives with company goals leads to better performance and more accurate data entry.
Company culture and values: A culture that values data integrity and transparency will give you better data quality and more accurate forecasts.
By considering these internal factors, you can improve forecasting accuracy and make better decisions about your business. Acknowledging and addressing these issues will transform sales forecasting from a problem into a competitive advantage.
Connecting the dots: How data gaps create forecast gaps
We've talked about the "Five Horsemen of the Data Apocalypse," but how do these data quality issues – including missing data – impact your sales forecasts?
The butterfly effect: Small data inconsistencies, big forecast deviations
In chaos theory, the butterfly effect suggests that small changes can have drastic effects. It's the idea that a butterfly flapping its wings can cause a hurricane to avoid New York City. In sales forecasting, the same principle applies. One deal without an amount or close date might not seem like a big deal, but when you multiply it by hundreds or thousands of opportunities, it becomes a perfect storm of inaccuracies.
Here's an example: if 10% of your opportunities are missing amounts and your average deal size is $100,000, a pipeline of 1,000 deals could be undervalued by $10 million. That's not a rounding error; it's a gap that can swallow your quarterly quota whole.
The ripple effect across departments: How bad data spreads across the organization
Your sales forecast isn't an island unto itself. It impacts decisions across the entire business:
Finance relies on it for budgeting and investor relations.
Operations uses it for resource allocation and capacity planning.
Marketing uses it to evaluate campaign effectiveness and plan future initiatives.
When your sales forecast is built on bad data, it creates a domino effect of poor decisions across the company. Marketing may pour more budget into campaigns that fail to produce qualified, high-value opportunities. Operations might overhire or understaff, incurring unnecessary costs or struggling to deliver. Finance could make critical mistakes in cash flow projections, affecting everything from hiring to strategic investments.
The credibility killer: Losing trust with stakeholders due to fluctuating forecasts
Bad data's worst side effect is loss of trust. When forecasts continue to miss the mark, stakeholders from the board of directors to team leaders stop believing what sales is telling them and lose trust in the organization's ability to forecast and hit numbers.
This lack of credibility can have far-reaching consequences:
Increased scrutiny and micromanaging of the sales process.
Trouble getting resources or budget for sales initiatives.
Strained relationships between sales and other teams.
In publicly traded companies, it can impact stock prices and investor confidence.
Trust is tough to regain; it's much easier to fix the root cause – bad data – than to continuously apologize for missed forecasts.
The human factor: Why sales teams can't get data quality right
Before we get to solutions, let's talk about why these data quality issues persist. After all, no sales rep is trying to intentionally run up inaccurate forecasts. One big reason is outdated data, which leads to missed opportunities and poor outcomes. The root causes are surprisingly human:
The time crunch: Data entry vs closing deals
Sales reps are under intense pressure to hit quota. Closing deals takes priority over data entry. It's the urgent versus the important. Reps think, "I'll get to that later," but later never arrives.
The optimism bias: When hope trumps data integrity
Salespeople are naturally optimistic – it's a job requirement. However, that optimism can translate to overly optimistic forecasts. Reps might keep stale deals in the pipeline, thinking "This could still close," or they might assign overly aggressive close dates.
The knowledge gap: Not understanding how individual data hygiene impacts the bigger picture
Many sales reps don't understand how their data hygiene practices impact the overall forecast. They might not know that omitting a deal amount or not updating a close date can add up to millions of dollars in forecast inaccuracies when rolled up from the team level.
Breaking the cycle: How to achieve data quality
Solving these problems requires a multi-pronged approach:
A key component is ensuring your email list quality is top-notch. Poor email list quality can lead to spam filters flagging your emails, damaging your reputation with internet service providers, and missing opportunities to engage with interested prospects.
Data-driven culture: Make quality a team sport
Data quality is everyone's responsibility, from your newest Sales Development Representative (SDR) to your Chief Revenue Officer. This means:
Including data quality metrics in rep performance reviews.
Rewarding good data hygiene as much as closed deals.
Offering regular training on the importance of accurate data.
Gamification of data integrity: Make data entry fun
Why not make data entry fun? Try:
Leaderboards for the most accurate forecasters.
Rewards for teams with the cleanest data.
Challenges or sprints focused on fixing specific data problems.
Empower your sales team — use our Sales Rep Commission Calculator to quickly calculate commissions and ensure fair, transparent payouts.
Automation: Reducing human error with smart systems
This is where technology can be a game-changer. Advanced CRM systems and AI-powered tools can:
Automatically alert you to data inconsistencies.
Predict realistic close dates based on historical trends.
Remind reps to follow up on stale opportunities.
The data integrity report: Your crystal ball for forecast clarity
This is where Forecastio's proprietary Data Integrity Report comes in. Designed to tackle the "Five Horsemen" head-on, it gives you unprecedented visibility into your data quality issues.
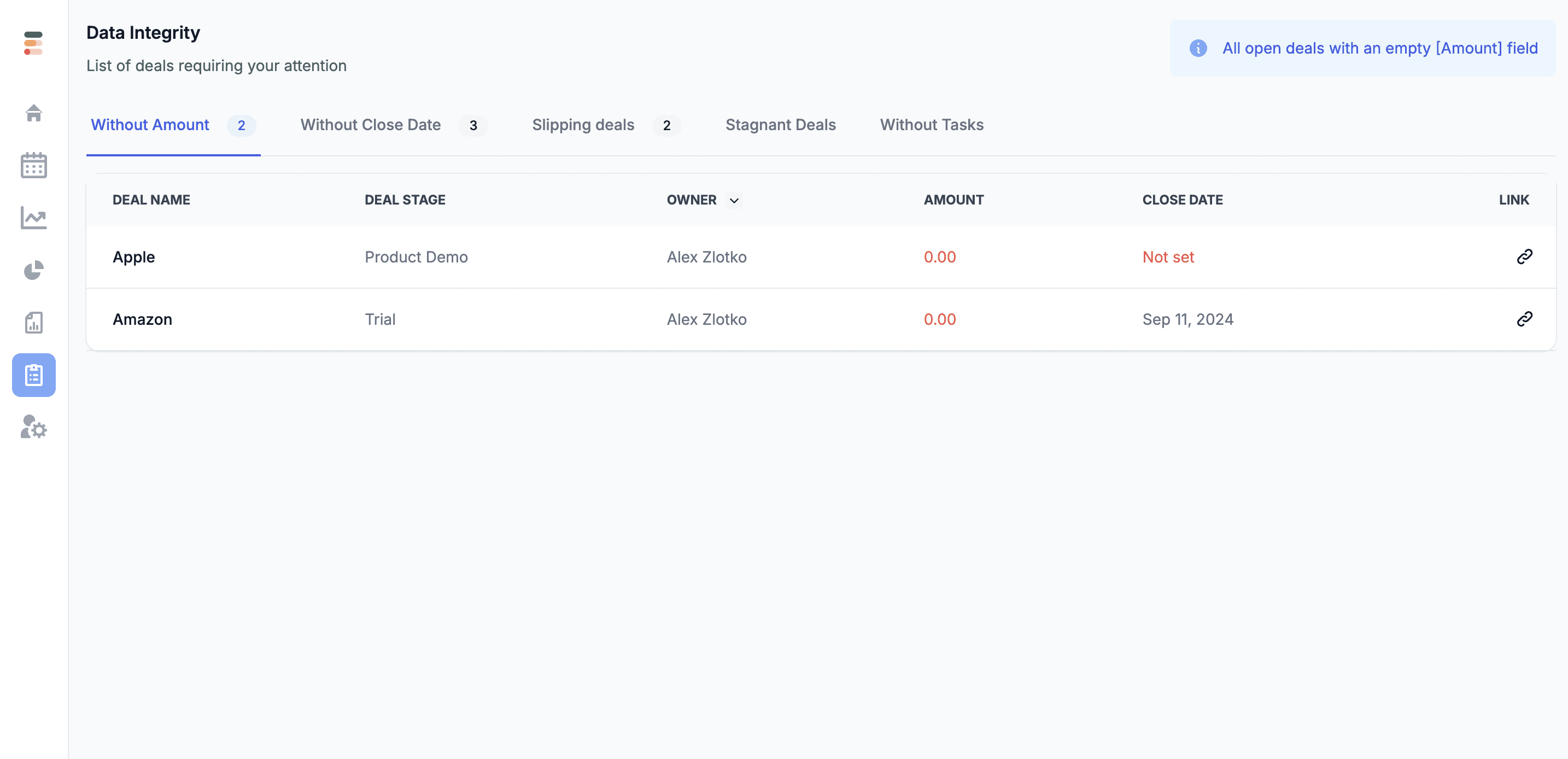
Introducing Forecastio's feature
The Data Integrity Report isn't just another dashboard – it's a diagnostic tool that scans your sales data for critical issues impacting forecast accuracy. It's like having a team of data analysts pour over your CRM, but automated and in real-time.
Drill down into the 5 critical Data Issues it finds
Deals without amounts: The report flags every opportunity without a monetary value, so you can quantify the unquantified and fill in the blanks.
Missing close dates: The report shines a light on deals floating in sales limbo, giving you clarity on your pipeline and enabling period-based forecasting.
Slipping deals: The system flags opportunities that have had their close dates pushed multiple times, so you can address sales momentum issues before it's too late.
Stagnant deals: By surfacing opportunities with no activity within a customizable time frame, it helps you revive stale deals or scrub them from your pipeline to keep it healthy.
Deals without tasks: The report surfaces opportunities without associated activities, so you can ensure every deal in your pipeline has a clear next step and is actively being pursued.
From insight to action: Using the report for immediate forecast improvement
The Data Integrity Report's greatest strength isn't just in identification – it's in action:
One-click updates let reps fix data issues directly from the report.
Bulk actions enable operations to quickly address systemic problems.
Trend analysis helps you spot recurring issues, so you can improve processes and training.
By addressing these issues quickly, sales teams can see forecast accuracy improve by 20% or more in the first quarter.
How to clean data boosts your entire sales operations
Improving data quality isn't just about fine-tuning your forecasts – though that alone is worth it. It's about creating a positive feedback loop that improves every part of your sales operation. Addressing duplicate data is critical in this process, as it can cause a host of issues like flawed analysis and inefficient workflows. Let's talk about how clean data can benefit your entire sales ecosystem.
Forecasting accuracy: Making decisions with accurate sales forecasts
When your data is clean and complete, your forecasts aren't guesses – they're predictions of future performance. This level of accuracy unlocks many benefits:
Resource allocation: With predictions of future revenue, you can make informed decisions about hiring, training, and resource deployment.
Strategic planning: Accurate forecasts let you set realistic goals and develop strategies based on data, not hope.
Investor relations: For public companies or those seeking funding, your ability to predict and hit revenue targets can impact valuation and investor confidence.
Check the accuracy of your sales forecasts with our Forecast Accuracy Calculator — ensure your predictions align with reality using Forecastio.
Sales team productivity: Focus on selling, not data management
Clean data isn't just a boon for leadership; it helps your entire sales team by:
Reduced administrative burden: Automated data integrity checks and alerts free reps from data entry drudgery to focus on what matters – selling.
Enhanced opportunity prioritization: When every deal in the pipeline is current, reps can focus on true sales priorities.
More effective coaching: Managers can coach to pipeline data, not data fantasies.
Cross-functional alignment: Finance, Sales, and Operations in Sync
Clean sales data provides a single source of truth for your entire organization:
Finance: Accurate revenue predictions help with budgeting and cash flow planning.
Marketing: Visible pipeline insights help marketing optimize programs and measure ROI.
Customer success: With visibility into incoming deals, customer success can prepare for onboarding and resource allocation.
Deal Velocity: Clean data as a fuel for faster closes
Believe it or not, clean data can actually help you close deals faster:
Improved follow-up: When every deal has tasks and up-to-date info, nothing falls through the cracks.
Enhanced buyer engagement: Reps with accurate, current information can better engage with prospects and address their needs.
Sales process optimization: By analyzing clean pipeline data, you can spot sales process bottlenecks and optimize for faster conversions.
The data quality imperative: Your path to forecasting success
As we've discussed throughout this post, the quality of your sales data isn't a technical issue – it's a strategic imperative that can make or break your organization. Bad data quality creates inaccurate forecasts, erodes trust with stakeholders, and holds your entire sales operation back from achieving its full potential.
The good news is: that you can turn data quality into a competitive advantage with the right approach and tools. Here's your roadmap to forecasting success:
Admit you have a problem: Identify the "Five Horsemen of the Data Apocalypse" infecting your sales data. Conduct a data quality audit.
Create a data culture: Make data quality a team sport. Train, incentivize, and implement processes to prioritize good data hygiene.
Take advantage of technology: Leverage tools like Forecastio's Data Integrity Report to automate data quality checks and simplify maintaining high standards for your team.
Take action: Don't just diagnose data issues – fix them. Use insights from your data quality tools to make immediate changes and implement long-term process improvements.
Monitor and adjust: Data quality isn't a one-and-done task. Regularly monitor your data health and be prepared to adjust processes as your business changes.
In the age of AI-driven sales, predictive analytics, and machine learning, organizations that master data quality will have a secret sauce. They won't just forecast better – they'll be able to maximize the benefits of advanced technologies to supercharge sales performance.
The question isn't can you afford to invest in data quality? In today's competitive market, it's: can you afford not to?
Your roadmap to forecasting success starts with one simple step: evaluate your current data quality.
Schedule a demo with Forecastio today to assess the quality of your HubSpot data. Make sure yours is conveying the right message.
Sales forecasting is one of those tasks that sales teams perform regularly, but it's so much more important than that. Forecasting is the backbone of your revenue recognition, and it's how executives make strategic decisions about the direction of the company.
But what happens when your forecast is wrong? It's often because your data is bad. And bad data leads to bad forecasts, missed quotas, and wasted time and money.
In this article, we'll explore how accurate data impacts your forecasts, how bad data holds you back, and what you can do to fix this common sales forecasting problem.
What is sales forecasting?
Sales forecasting is the process of estimating future sales revenue over a set period. It's an art and a science, using historical sales data, market trends, and other factors to make an educated guess about future sales. Accurate data is crucial for retaining existing customers and earning their loyalty. Without it, you're sailing a ship without a map or compass.
Using statistical models, machine learning algorithms, and expert judgment, companies can predict the probability of future sales and revenue. This is critical for making decisions about production, inventory, pricing, and resource allocation. Sales forecasting is the foundation of strategic planning, and it guides your company toward success.
Why sales forecasting matters
Why is sales forecasting so important? It's the foundation of all business decision-making. Your sales and marketing teams need accurate data to succeed, so precise forecasts are critical. Accurate sales forecasts give you the ability to:
Set realistic sales goals and quotas: Knowing what to shoot for keeps reps motivated and focused.
Manage resources: From staff to inventory, knowing what to expect in terms of future sales helps you plan.
Make informed product and pricing decisions: If you know what's selling and what's not, you can make strategic decisions about product lines and pricing.
Plan for inventory and production: If you know what to expect in terms of future sales, you can plan for inventory and production.
Determine revenue recognition: Accurate sales forecasts give you the ability to recognize revenue and make financial projections.
Guide strategic business decisions: Sales forecasting is the backbone of strategic planning, and it guides your company toward success.
Manage cash flow and budgeting: Knowing what to expect in terms of revenue helps you plan for cash flow and budgeting.
Plan for staffing and training: Knowing if you'll experience sales growth helps you hire and train the right people at the right time.
Determine opportunities for growth and expansion: Trends and patterns can reveal new markets or products.
Mitigate risks and uncertainties: Knowing what to expect helps you prepare for downturns.
In short, sales forecasting isn't a nicety; it's a necessity if you want your business to succeed.
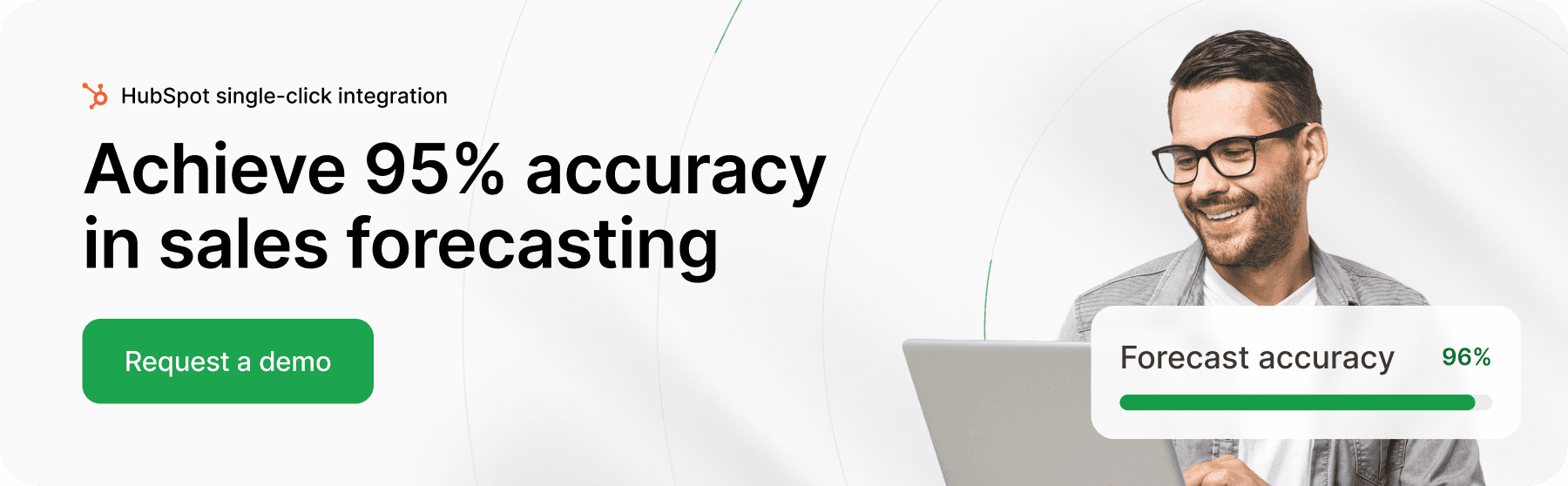
Sales forecasting process overview
The sales forecasting process is a methodology that outlines the steps you should take to forecast sales. Here's an overview of the process:
Data collection: This is where you gather historical sales data, market trends, and other relevant information. This is the fuel for your forecast. It's critical to address duplicate data at this stage to ensure data quality and prevent garbage-in, and garbage-out analysis.
Data analysis: Once you have your data, you need to analyze it to discover trends, patterns, and correlations. This is where you'll learn what drives sales.
Model selection: Select a forecasting model based on your data and business needs. This could be a simple moving average or a complex machine-learning model.
Forecasting: Use your model to forecast sales. This is where the magic happens, and your data becomes a valuable forecast.
Review and revision: Finally, you need to review and revise your forecast regularly. Sales forecasting isn't a one-and-done task; it's an ongoing process.
By following these steps, you can create a solid sales forecast that guides your strategy and drives growth.
The illusion of accuracy: The data quality crisis
The end of the quarter. Your sales team has worked tirelessly, and your CRM is full of numbers. Your forecast looks great. You present it to the board, and at the 11th hour, deals fall through or come in from left field. Sound familiar?
You're not alone. Many sales leaders are tempted by the wealth of data at their fingertips and assume quality when they see quantity.
But the truth is that in sales forecasting, bad data – aka dirty data – is often worse than no data at all. Accurate sales forecasting is critical for business planning and resource allocation, and overcoming common sales forecasting challenges is key to success.
The effects of poor data quality go far beyond inaccurate forecasts. It's a silent killer that erodes trust, misallocates resources and can impact your company's valuation. According to IBM, poor data quality costs the U.S. economy approximately $3.1 trillion each year. For sales teams, it means missed opportunities, wasted time and money, and a cycle of chasing instead of strategizing.
The five horsemen of the data apocalypse
Let's talk about the top five data quality issues that impact your sales forecasts and revenue recognition. We call them the "Five Horsemen of the Data Apocalypse" – dramatic, we know, but they're apocalyptic for your revenue recognition.
One of the biggest problems is simply poor-quality data. We're talking about bad data, but it goes by many names: sloppy data, poor data, lousy data. Whatever you call it, it's a serious problem that can severely impact operational effectiveness in B2B sales and marketing.
1. The ghost deal: Opportunities without amounts
How can you forecast your expenses without knowing how much money you'll make? Ridiculous, right? Yet many sales pipelines are haunted by these "ghost deals" – opportunities that are in the system, but lack one critical detail: the deal amount.
These ethereal deals skew your entire forecast. They could be $10,000 or $100,000 deals, but without an amount, you can't tell. As a result, sales leaders are forced to ignore these opportunities entirely (potentially leaving real revenue on the table) or make educated guesses about the value (introducing huge inaccuracies into the forecast). To forecast future sales accurately, you need detailed records and advanced forecasting methods.
2. The timeless wonder: Deals without close dates
In sales forecasting, timing is everything. A deal that's expected to close this quarter is much different from one that's expected to close next year. Accurate sales forecasting is all about having good data to create solid sales projections. Enter the "Timeless Wonders" – opportunities that are missing a critical piece of information: a close date.
These dateless deals can't be used to create time-based forecasts. They pad your pipeline and can skew your short-term or long-term forecasts. They often indicate a lack of qualification or next steps, exposing deeper issues in your sales process.
3. Walking Dead deals: The deals that constantly slip
We've all been there: a deal that's slipping from quarter to quarter. These "Eternal Sliders" are the bane of accurate forecasting. They make your pipeline look healthy, but your forecast is another story altogether.
Slipping deals often indicate a deeper problem: perhaps your value proposition isn't strong enough, decision-makers aren't engaged, or your timeline isn't aligned with the customer's. Historical sales data can help you identify these sliders, and it's critical for both accurate forecasting and fixing your sales process.
4. The fossil record: Deals that won't budge
Every pipeline has them: opportunities that are fossilized in your pipeline after months (or years). These "Fossil Records" are often the leftovers of overzealous sales reps or changes in the buyer's situation that weren't updated in the system.
Stale deals pad your pipeline and skew your sales metrics, such as average deal size and sales cycle length. They can also mask real issues in your sales process, like poor follow-up or inadequate qualification criteria.
Most importantly, they give a false impression of future revenue potential, introducing huge inaccuracies into your forecast and resource allocation. The right sales forecasting methods are critical – not all methods are right for every business.
5. The lone wolf: Deals without tasks or activities
Sales is an ongoing process. An opportunity without tasks or activities is a plant without water – it's not going anywhere. Using sales forecasting software can help you forecast more accurately and efficiently by leveraging historical data and market trends.
It also provides actionable intelligence and real-time visibility to inform your sales strategies. These "Lone Wolves" are often ignored in your forecast, introducing blind spots into your projections.
A lack of tasks or activities usually indicates the deal isn't being actively pursued. Perhaps the sales rep is overwhelmed, the opportunity was deprioritized, or CRM hygiene is poor. Whatever the reason, these leads introduce huge uncertainty into your forecast, and they're likely wasting away in your pipeline.
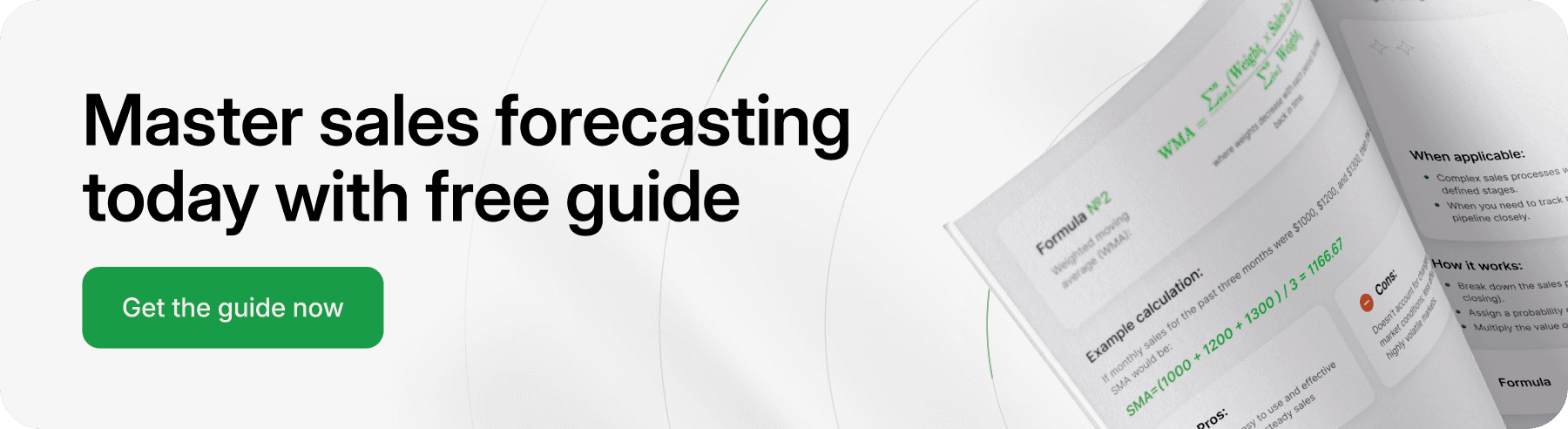
Sales forecast factors
Internal factors: Hires and fires, policy changes, territory shifts
Internal factors can impact sales forecasts in big and small ways. Let's talk about some of those internal factors:
Hires and fires: Changes to the sales team can ripple through sales and forecasting. Bringing in new reps can give you a sales boost while losing a top performer can be hard to recover from.
Policy changes: Changes to company policy, such as pricing strategy or commission structure, can impact sales directly. Aggressive pricing can drive more sales while changing the commission structure can motivate or demotivate your sales team.
Territory shifts: Reassigning territories or changing sales assignments can break relationships and impact sales. A well-planned territory reassignment can help you cover more ground and drive more sales, but a poorly executed change can confuse customers and cost you deals.
These internal factors are impacted by:
Sales team performance and morale: A motivated and supported sales team will hit quota and maintain good data quality.
Training and development programs: Ongoing training and development help sales reps fine-tune their skills and improve forecasting accuracy.
Sales process and methodology: A defined sales process ensures consistency and reliability in data collection and forecasting.
Compensation and incentive structures: Aligning incentives with company goals leads to better performance and more accurate data entry.
Company culture and values: A culture that values data integrity and transparency will give you better data quality and more accurate forecasts.
By considering these internal factors, you can improve forecasting accuracy and make better decisions about your business. Acknowledging and addressing these issues will transform sales forecasting from a problem into a competitive advantage.
Connecting the dots: How data gaps create forecast gaps
We've talked about the "Five Horsemen of the Data Apocalypse," but how do these data quality issues – including missing data – impact your sales forecasts?
The butterfly effect: Small data inconsistencies, big forecast deviations
In chaos theory, the butterfly effect suggests that small changes can have drastic effects. It's the idea that a butterfly flapping its wings can cause a hurricane to avoid New York City. In sales forecasting, the same principle applies. One deal without an amount or close date might not seem like a big deal, but when you multiply it by hundreds or thousands of opportunities, it becomes a perfect storm of inaccuracies.
Here's an example: if 10% of your opportunities are missing amounts and your average deal size is $100,000, a pipeline of 1,000 deals could be undervalued by $10 million. That's not a rounding error; it's a gap that can swallow your quarterly quota whole.
The ripple effect across departments: How bad data spreads across the organization
Your sales forecast isn't an island unto itself. It impacts decisions across the entire business:
Finance relies on it for budgeting and investor relations.
Operations uses it for resource allocation and capacity planning.
Marketing uses it to evaluate campaign effectiveness and plan future initiatives.
When your sales forecast is built on bad data, it creates a domino effect of poor decisions across the company. Marketing may pour more budget into campaigns that fail to produce qualified, high-value opportunities. Operations might overhire or understaff, incurring unnecessary costs or struggling to deliver. Finance could make critical mistakes in cash flow projections, affecting everything from hiring to strategic investments.
The credibility killer: Losing trust with stakeholders due to fluctuating forecasts
Bad data's worst side effect is loss of trust. When forecasts continue to miss the mark, stakeholders from the board of directors to team leaders stop believing what sales is telling them and lose trust in the organization's ability to forecast and hit numbers.
This lack of credibility can have far-reaching consequences:
Increased scrutiny and micromanaging of the sales process.
Trouble getting resources or budget for sales initiatives.
Strained relationships between sales and other teams.
In publicly traded companies, it can impact stock prices and investor confidence.
Trust is tough to regain; it's much easier to fix the root cause – bad data – than to continuously apologize for missed forecasts.
The human factor: Why sales teams can't get data quality right
Before we get to solutions, let's talk about why these data quality issues persist. After all, no sales rep is trying to intentionally run up inaccurate forecasts. One big reason is outdated data, which leads to missed opportunities and poor outcomes. The root causes are surprisingly human:
The time crunch: Data entry vs closing deals
Sales reps are under intense pressure to hit quota. Closing deals takes priority over data entry. It's the urgent versus the important. Reps think, "I'll get to that later," but later never arrives.
The optimism bias: When hope trumps data integrity
Salespeople are naturally optimistic – it's a job requirement. However, that optimism can translate to overly optimistic forecasts. Reps might keep stale deals in the pipeline, thinking "This could still close," or they might assign overly aggressive close dates.
The knowledge gap: Not understanding how individual data hygiene impacts the bigger picture
Many sales reps don't understand how their data hygiene practices impact the overall forecast. They might not know that omitting a deal amount or not updating a close date can add up to millions of dollars in forecast inaccuracies when rolled up from the team level.
Breaking the cycle: How to achieve data quality
Solving these problems requires a multi-pronged approach:
A key component is ensuring your email list quality is top-notch. Poor email list quality can lead to spam filters flagging your emails, damaging your reputation with internet service providers, and missing opportunities to engage with interested prospects.
Data-driven culture: Make quality a team sport
Data quality is everyone's responsibility, from your newest Sales Development Representative (SDR) to your Chief Revenue Officer. This means:
Including data quality metrics in rep performance reviews.
Rewarding good data hygiene as much as closed deals.
Offering regular training on the importance of accurate data.
Gamification of data integrity: Make data entry fun
Why not make data entry fun? Try:
Leaderboards for the most accurate forecasters.
Rewards for teams with the cleanest data.
Challenges or sprints focused on fixing specific data problems.
Empower your sales team — use our Sales Rep Commission Calculator to quickly calculate commissions and ensure fair, transparent payouts.
Automation: Reducing human error with smart systems
This is where technology can be a game-changer. Advanced CRM systems and AI-powered tools can:
Automatically alert you to data inconsistencies.
Predict realistic close dates based on historical trends.
Remind reps to follow up on stale opportunities.
The data integrity report: Your crystal ball for forecast clarity
This is where Forecastio's proprietary Data Integrity Report comes in. Designed to tackle the "Five Horsemen" head-on, it gives you unprecedented visibility into your data quality issues.
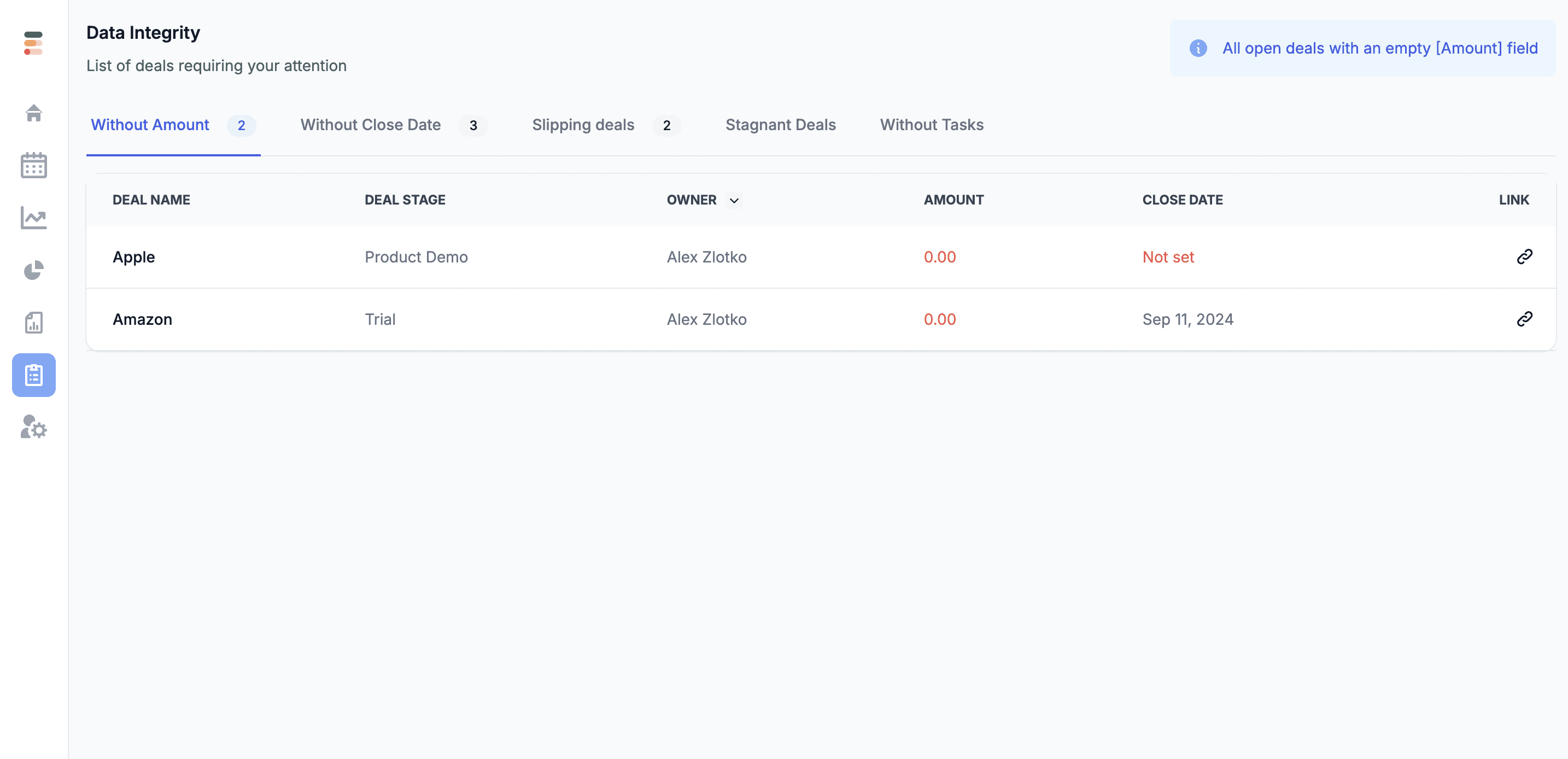
Introducing Forecastio's feature
The Data Integrity Report isn't just another dashboard – it's a diagnostic tool that scans your sales data for critical issues impacting forecast accuracy. It's like having a team of data analysts pour over your CRM, but automated and in real-time.
Drill down into the 5 critical Data Issues it finds
Deals without amounts: The report flags every opportunity without a monetary value, so you can quantify the unquantified and fill in the blanks.
Missing close dates: The report shines a light on deals floating in sales limbo, giving you clarity on your pipeline and enabling period-based forecasting.
Slipping deals: The system flags opportunities that have had their close dates pushed multiple times, so you can address sales momentum issues before it's too late.
Stagnant deals: By surfacing opportunities with no activity within a customizable time frame, it helps you revive stale deals or scrub them from your pipeline to keep it healthy.
Deals without tasks: The report surfaces opportunities without associated activities, so you can ensure every deal in your pipeline has a clear next step and is actively being pursued.
From insight to action: Using the report for immediate forecast improvement
The Data Integrity Report's greatest strength isn't just in identification – it's in action:
One-click updates let reps fix data issues directly from the report.
Bulk actions enable operations to quickly address systemic problems.
Trend analysis helps you spot recurring issues, so you can improve processes and training.
By addressing these issues quickly, sales teams can see forecast accuracy improve by 20% or more in the first quarter.
How to clean data boosts your entire sales operations
Improving data quality isn't just about fine-tuning your forecasts – though that alone is worth it. It's about creating a positive feedback loop that improves every part of your sales operation. Addressing duplicate data is critical in this process, as it can cause a host of issues like flawed analysis and inefficient workflows. Let's talk about how clean data can benefit your entire sales ecosystem.
Forecasting accuracy: Making decisions with accurate sales forecasts
When your data is clean and complete, your forecasts aren't guesses – they're predictions of future performance. This level of accuracy unlocks many benefits:
Resource allocation: With predictions of future revenue, you can make informed decisions about hiring, training, and resource deployment.
Strategic planning: Accurate forecasts let you set realistic goals and develop strategies based on data, not hope.
Investor relations: For public companies or those seeking funding, your ability to predict and hit revenue targets can impact valuation and investor confidence.
Check the accuracy of your sales forecasts with our Forecast Accuracy Calculator — ensure your predictions align with reality using Forecastio.
Sales team productivity: Focus on selling, not data management
Clean data isn't just a boon for leadership; it helps your entire sales team by:
Reduced administrative burden: Automated data integrity checks and alerts free reps from data entry drudgery to focus on what matters – selling.
Enhanced opportunity prioritization: When every deal in the pipeline is current, reps can focus on true sales priorities.
More effective coaching: Managers can coach to pipeline data, not data fantasies.
Cross-functional alignment: Finance, Sales, and Operations in Sync
Clean sales data provides a single source of truth for your entire organization:
Finance: Accurate revenue predictions help with budgeting and cash flow planning.
Marketing: Visible pipeline insights help marketing optimize programs and measure ROI.
Customer success: With visibility into incoming deals, customer success can prepare for onboarding and resource allocation.
Deal Velocity: Clean data as a fuel for faster closes
Believe it or not, clean data can actually help you close deals faster:
Improved follow-up: When every deal has tasks and up-to-date info, nothing falls through the cracks.
Enhanced buyer engagement: Reps with accurate, current information can better engage with prospects and address their needs.
Sales process optimization: By analyzing clean pipeline data, you can spot sales process bottlenecks and optimize for faster conversions.
The data quality imperative: Your path to forecasting success
As we've discussed throughout this post, the quality of your sales data isn't a technical issue – it's a strategic imperative that can make or break your organization. Bad data quality creates inaccurate forecasts, erodes trust with stakeholders, and holds your entire sales operation back from achieving its full potential.
The good news is: that you can turn data quality into a competitive advantage with the right approach and tools. Here's your roadmap to forecasting success:
Admit you have a problem: Identify the "Five Horsemen of the Data Apocalypse" infecting your sales data. Conduct a data quality audit.
Create a data culture: Make data quality a team sport. Train, incentivize, and implement processes to prioritize good data hygiene.
Take advantage of technology: Leverage tools like Forecastio's Data Integrity Report to automate data quality checks and simplify maintaining high standards for your team.
Take action: Don't just diagnose data issues – fix them. Use insights from your data quality tools to make immediate changes and implement long-term process improvements.
Monitor and adjust: Data quality isn't a one-and-done task. Regularly monitor your data health and be prepared to adjust processes as your business changes.
In the age of AI-driven sales, predictive analytics, and machine learning, organizations that master data quality will have a secret sauce. They won't just forecast better – they'll be able to maximize the benefits of advanced technologies to supercharge sales performance.
The question isn't can you afford to invest in data quality? In today's competitive market, it's: can you afford not to?
Your roadmap to forecasting success starts with one simple step: evaluate your current data quality.
Schedule a demo with Forecastio today to assess the quality of your HubSpot data. Make sure yours is conveying the right message.
Sales forecasting is one of those tasks that sales teams perform regularly, but it's so much more important than that. Forecasting is the backbone of your revenue recognition, and it's how executives make strategic decisions about the direction of the company.
But what happens when your forecast is wrong? It's often because your data is bad. And bad data leads to bad forecasts, missed quotas, and wasted time and money.
In this article, we'll explore how accurate data impacts your forecasts, how bad data holds you back, and what you can do to fix this common sales forecasting problem.
What is sales forecasting?
Sales forecasting is the process of estimating future sales revenue over a set period. It's an art and a science, using historical sales data, market trends, and other factors to make an educated guess about future sales. Accurate data is crucial for retaining existing customers and earning their loyalty. Without it, you're sailing a ship without a map or compass.
Using statistical models, machine learning algorithms, and expert judgment, companies can predict the probability of future sales and revenue. This is critical for making decisions about production, inventory, pricing, and resource allocation. Sales forecasting is the foundation of strategic planning, and it guides your company toward success.
Why sales forecasting matters
Why is sales forecasting so important? It's the foundation of all business decision-making. Your sales and marketing teams need accurate data to succeed, so precise forecasts are critical. Accurate sales forecasts give you the ability to:
Set realistic sales goals and quotas: Knowing what to shoot for keeps reps motivated and focused.
Manage resources: From staff to inventory, knowing what to expect in terms of future sales helps you plan.
Make informed product and pricing decisions: If you know what's selling and what's not, you can make strategic decisions about product lines and pricing.
Plan for inventory and production: If you know what to expect in terms of future sales, you can plan for inventory and production.
Determine revenue recognition: Accurate sales forecasts give you the ability to recognize revenue and make financial projections.
Guide strategic business decisions: Sales forecasting is the backbone of strategic planning, and it guides your company toward success.
Manage cash flow and budgeting: Knowing what to expect in terms of revenue helps you plan for cash flow and budgeting.
Plan for staffing and training: Knowing if you'll experience sales growth helps you hire and train the right people at the right time.
Determine opportunities for growth and expansion: Trends and patterns can reveal new markets or products.
Mitigate risks and uncertainties: Knowing what to expect helps you prepare for downturns.
In short, sales forecasting isn't a nicety; it's a necessity if you want your business to succeed.
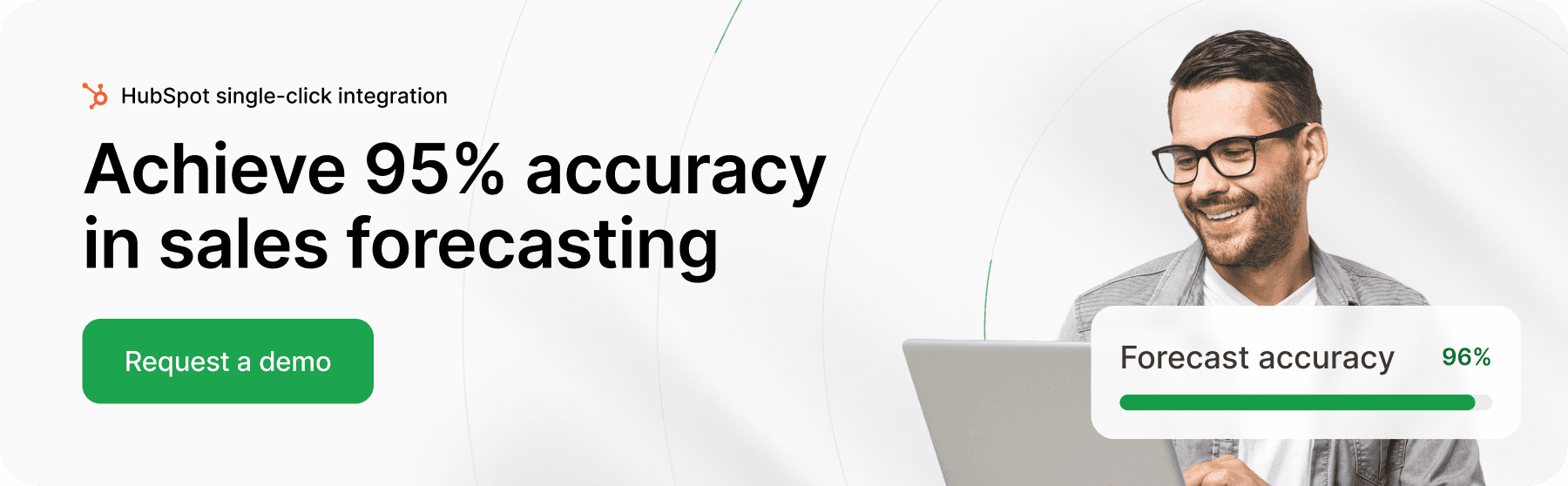
Sales forecasting process overview
The sales forecasting process is a methodology that outlines the steps you should take to forecast sales. Here's an overview of the process:
Data collection: This is where you gather historical sales data, market trends, and other relevant information. This is the fuel for your forecast. It's critical to address duplicate data at this stage to ensure data quality and prevent garbage-in, and garbage-out analysis.
Data analysis: Once you have your data, you need to analyze it to discover trends, patterns, and correlations. This is where you'll learn what drives sales.
Model selection: Select a forecasting model based on your data and business needs. This could be a simple moving average or a complex machine-learning model.
Forecasting: Use your model to forecast sales. This is where the magic happens, and your data becomes a valuable forecast.
Review and revision: Finally, you need to review and revise your forecast regularly. Sales forecasting isn't a one-and-done task; it's an ongoing process.
By following these steps, you can create a solid sales forecast that guides your strategy and drives growth.
The illusion of accuracy: The data quality crisis
The end of the quarter. Your sales team has worked tirelessly, and your CRM is full of numbers. Your forecast looks great. You present it to the board, and at the 11th hour, deals fall through or come in from left field. Sound familiar?
You're not alone. Many sales leaders are tempted by the wealth of data at their fingertips and assume quality when they see quantity.
But the truth is that in sales forecasting, bad data – aka dirty data – is often worse than no data at all. Accurate sales forecasting is critical for business planning and resource allocation, and overcoming common sales forecasting challenges is key to success.
The effects of poor data quality go far beyond inaccurate forecasts. It's a silent killer that erodes trust, misallocates resources and can impact your company's valuation. According to IBM, poor data quality costs the U.S. economy approximately $3.1 trillion each year. For sales teams, it means missed opportunities, wasted time and money, and a cycle of chasing instead of strategizing.
The five horsemen of the data apocalypse
Let's talk about the top five data quality issues that impact your sales forecasts and revenue recognition. We call them the "Five Horsemen of the Data Apocalypse" – dramatic, we know, but they're apocalyptic for your revenue recognition.
One of the biggest problems is simply poor-quality data. We're talking about bad data, but it goes by many names: sloppy data, poor data, lousy data. Whatever you call it, it's a serious problem that can severely impact operational effectiveness in B2B sales and marketing.
1. The ghost deal: Opportunities without amounts
How can you forecast your expenses without knowing how much money you'll make? Ridiculous, right? Yet many sales pipelines are haunted by these "ghost deals" – opportunities that are in the system, but lack one critical detail: the deal amount.
These ethereal deals skew your entire forecast. They could be $10,000 or $100,000 deals, but without an amount, you can't tell. As a result, sales leaders are forced to ignore these opportunities entirely (potentially leaving real revenue on the table) or make educated guesses about the value (introducing huge inaccuracies into the forecast). To forecast future sales accurately, you need detailed records and advanced forecasting methods.
2. The timeless wonder: Deals without close dates
In sales forecasting, timing is everything. A deal that's expected to close this quarter is much different from one that's expected to close next year. Accurate sales forecasting is all about having good data to create solid sales projections. Enter the "Timeless Wonders" – opportunities that are missing a critical piece of information: a close date.
These dateless deals can't be used to create time-based forecasts. They pad your pipeline and can skew your short-term or long-term forecasts. They often indicate a lack of qualification or next steps, exposing deeper issues in your sales process.
3. Walking Dead deals: The deals that constantly slip
We've all been there: a deal that's slipping from quarter to quarter. These "Eternal Sliders" are the bane of accurate forecasting. They make your pipeline look healthy, but your forecast is another story altogether.
Slipping deals often indicate a deeper problem: perhaps your value proposition isn't strong enough, decision-makers aren't engaged, or your timeline isn't aligned with the customer's. Historical sales data can help you identify these sliders, and it's critical for both accurate forecasting and fixing your sales process.
4. The fossil record: Deals that won't budge
Every pipeline has them: opportunities that are fossilized in your pipeline after months (or years). These "Fossil Records" are often the leftovers of overzealous sales reps or changes in the buyer's situation that weren't updated in the system.
Stale deals pad your pipeline and skew your sales metrics, such as average deal size and sales cycle length. They can also mask real issues in your sales process, like poor follow-up or inadequate qualification criteria.
Most importantly, they give a false impression of future revenue potential, introducing huge inaccuracies into your forecast and resource allocation. The right sales forecasting methods are critical – not all methods are right for every business.
5. The lone wolf: Deals without tasks or activities
Sales is an ongoing process. An opportunity without tasks or activities is a plant without water – it's not going anywhere. Using sales forecasting software can help you forecast more accurately and efficiently by leveraging historical data and market trends.
It also provides actionable intelligence and real-time visibility to inform your sales strategies. These "Lone Wolves" are often ignored in your forecast, introducing blind spots into your projections.
A lack of tasks or activities usually indicates the deal isn't being actively pursued. Perhaps the sales rep is overwhelmed, the opportunity was deprioritized, or CRM hygiene is poor. Whatever the reason, these leads introduce huge uncertainty into your forecast, and they're likely wasting away in your pipeline.
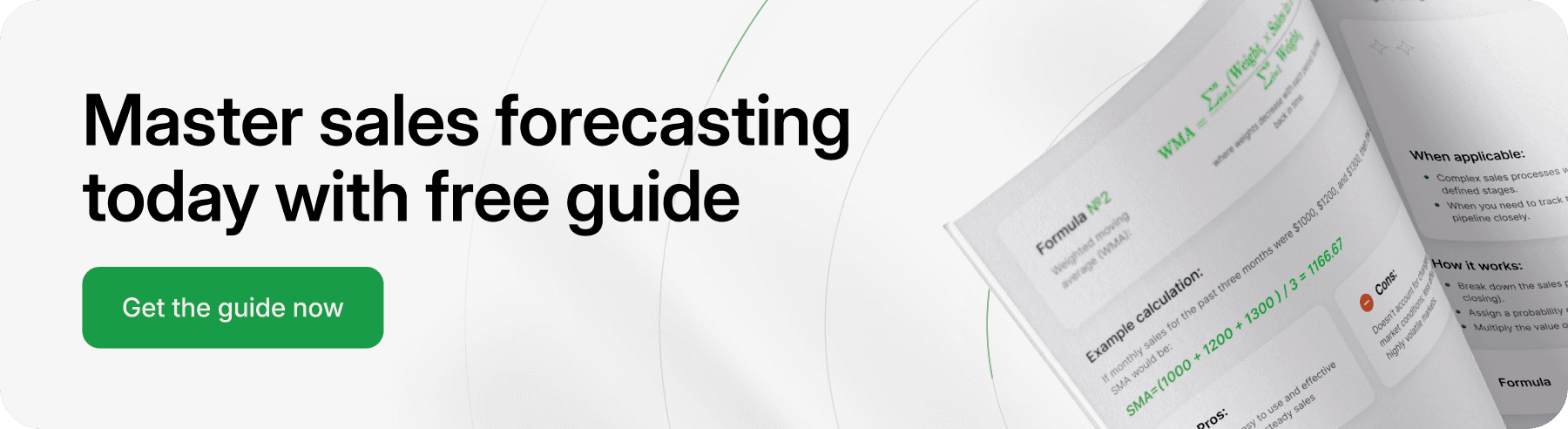
Sales forecast factors
Internal factors: Hires and fires, policy changes, territory shifts
Internal factors can impact sales forecasts in big and small ways. Let's talk about some of those internal factors:
Hires and fires: Changes to the sales team can ripple through sales and forecasting. Bringing in new reps can give you a sales boost while losing a top performer can be hard to recover from.
Policy changes: Changes to company policy, such as pricing strategy or commission structure, can impact sales directly. Aggressive pricing can drive more sales while changing the commission structure can motivate or demotivate your sales team.
Territory shifts: Reassigning territories or changing sales assignments can break relationships and impact sales. A well-planned territory reassignment can help you cover more ground and drive more sales, but a poorly executed change can confuse customers and cost you deals.
These internal factors are impacted by:
Sales team performance and morale: A motivated and supported sales team will hit quota and maintain good data quality.
Training and development programs: Ongoing training and development help sales reps fine-tune their skills and improve forecasting accuracy.
Sales process and methodology: A defined sales process ensures consistency and reliability in data collection and forecasting.
Compensation and incentive structures: Aligning incentives with company goals leads to better performance and more accurate data entry.
Company culture and values: A culture that values data integrity and transparency will give you better data quality and more accurate forecasts.
By considering these internal factors, you can improve forecasting accuracy and make better decisions about your business. Acknowledging and addressing these issues will transform sales forecasting from a problem into a competitive advantage.
Connecting the dots: How data gaps create forecast gaps
We've talked about the "Five Horsemen of the Data Apocalypse," but how do these data quality issues – including missing data – impact your sales forecasts?
The butterfly effect: Small data inconsistencies, big forecast deviations
In chaos theory, the butterfly effect suggests that small changes can have drastic effects. It's the idea that a butterfly flapping its wings can cause a hurricane to avoid New York City. In sales forecasting, the same principle applies. One deal without an amount or close date might not seem like a big deal, but when you multiply it by hundreds or thousands of opportunities, it becomes a perfect storm of inaccuracies.
Here's an example: if 10% of your opportunities are missing amounts and your average deal size is $100,000, a pipeline of 1,000 deals could be undervalued by $10 million. That's not a rounding error; it's a gap that can swallow your quarterly quota whole.
The ripple effect across departments: How bad data spreads across the organization
Your sales forecast isn't an island unto itself. It impacts decisions across the entire business:
Finance relies on it for budgeting and investor relations.
Operations uses it for resource allocation and capacity planning.
Marketing uses it to evaluate campaign effectiveness and plan future initiatives.
When your sales forecast is built on bad data, it creates a domino effect of poor decisions across the company. Marketing may pour more budget into campaigns that fail to produce qualified, high-value opportunities. Operations might overhire or understaff, incurring unnecessary costs or struggling to deliver. Finance could make critical mistakes in cash flow projections, affecting everything from hiring to strategic investments.
The credibility killer: Losing trust with stakeholders due to fluctuating forecasts
Bad data's worst side effect is loss of trust. When forecasts continue to miss the mark, stakeholders from the board of directors to team leaders stop believing what sales is telling them and lose trust in the organization's ability to forecast and hit numbers.
This lack of credibility can have far-reaching consequences:
Increased scrutiny and micromanaging of the sales process.
Trouble getting resources or budget for sales initiatives.
Strained relationships between sales and other teams.
In publicly traded companies, it can impact stock prices and investor confidence.
Trust is tough to regain; it's much easier to fix the root cause – bad data – than to continuously apologize for missed forecasts.
The human factor: Why sales teams can't get data quality right
Before we get to solutions, let's talk about why these data quality issues persist. After all, no sales rep is trying to intentionally run up inaccurate forecasts. One big reason is outdated data, which leads to missed opportunities and poor outcomes. The root causes are surprisingly human:
The time crunch: Data entry vs closing deals
Sales reps are under intense pressure to hit quota. Closing deals takes priority over data entry. It's the urgent versus the important. Reps think, "I'll get to that later," but later never arrives.
The optimism bias: When hope trumps data integrity
Salespeople are naturally optimistic – it's a job requirement. However, that optimism can translate to overly optimistic forecasts. Reps might keep stale deals in the pipeline, thinking "This could still close," or they might assign overly aggressive close dates.
The knowledge gap: Not understanding how individual data hygiene impacts the bigger picture
Many sales reps don't understand how their data hygiene practices impact the overall forecast. They might not know that omitting a deal amount or not updating a close date can add up to millions of dollars in forecast inaccuracies when rolled up from the team level.
Breaking the cycle: How to achieve data quality
Solving these problems requires a multi-pronged approach:
A key component is ensuring your email list quality is top-notch. Poor email list quality can lead to spam filters flagging your emails, damaging your reputation with internet service providers, and missing opportunities to engage with interested prospects.
Data-driven culture: Make quality a team sport
Data quality is everyone's responsibility, from your newest Sales Development Representative (SDR) to your Chief Revenue Officer. This means:
Including data quality metrics in rep performance reviews.
Rewarding good data hygiene as much as closed deals.
Offering regular training on the importance of accurate data.
Gamification of data integrity: Make data entry fun
Why not make data entry fun? Try:
Leaderboards for the most accurate forecasters.
Rewards for teams with the cleanest data.
Challenges or sprints focused on fixing specific data problems.
Empower your sales team — use our Sales Rep Commission Calculator to quickly calculate commissions and ensure fair, transparent payouts.
Automation: Reducing human error with smart systems
This is where technology can be a game-changer. Advanced CRM systems and AI-powered tools can:
Automatically alert you to data inconsistencies.
Predict realistic close dates based on historical trends.
Remind reps to follow up on stale opportunities.
The data integrity report: Your crystal ball for forecast clarity
This is where Forecastio's proprietary Data Integrity Report comes in. Designed to tackle the "Five Horsemen" head-on, it gives you unprecedented visibility into your data quality issues.
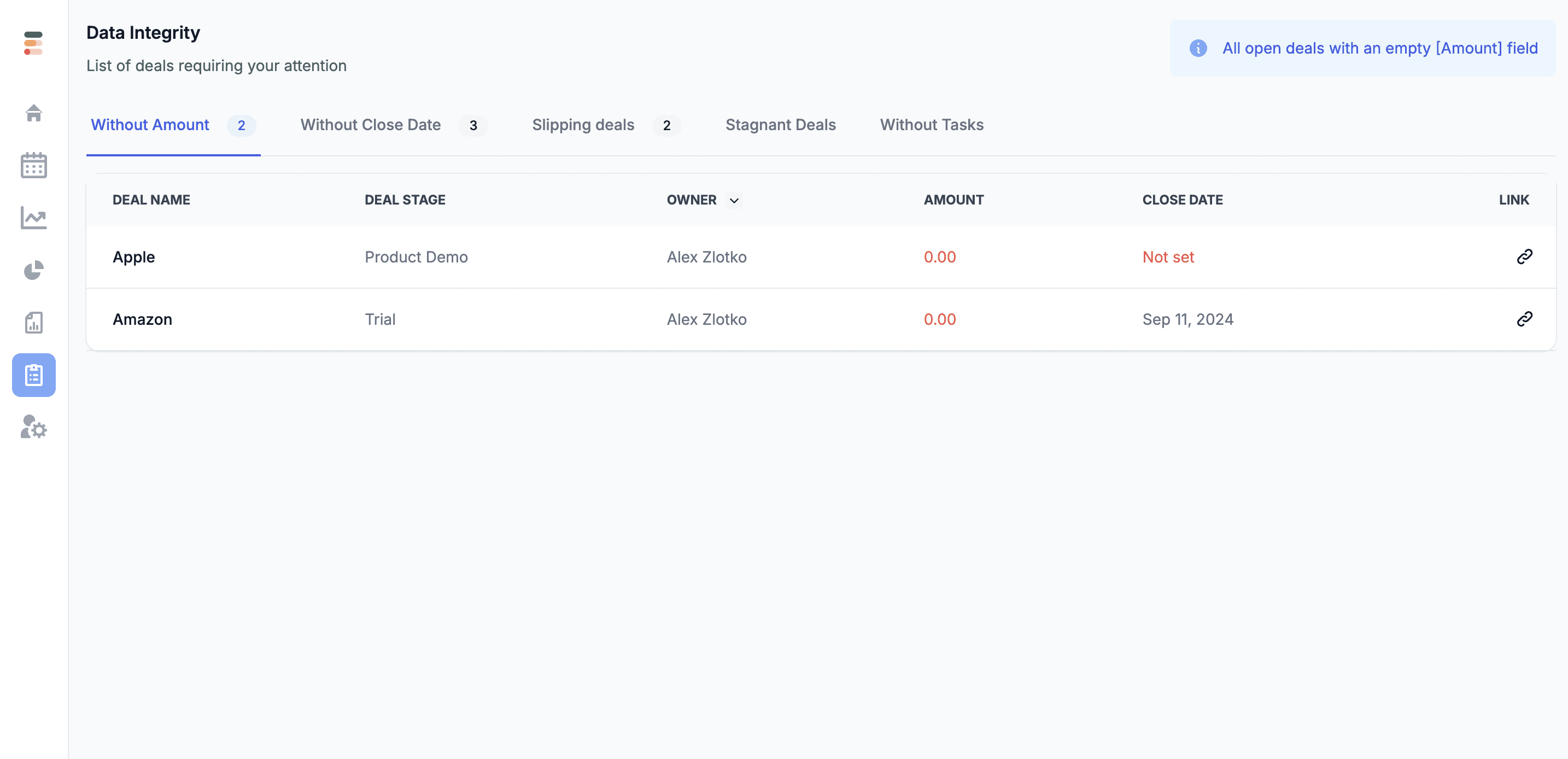
Introducing Forecastio's feature
The Data Integrity Report isn't just another dashboard – it's a diagnostic tool that scans your sales data for critical issues impacting forecast accuracy. It's like having a team of data analysts pour over your CRM, but automated and in real-time.
Drill down into the 5 critical Data Issues it finds
Deals without amounts: The report flags every opportunity without a monetary value, so you can quantify the unquantified and fill in the blanks.
Missing close dates: The report shines a light on deals floating in sales limbo, giving you clarity on your pipeline and enabling period-based forecasting.
Slipping deals: The system flags opportunities that have had their close dates pushed multiple times, so you can address sales momentum issues before it's too late.
Stagnant deals: By surfacing opportunities with no activity within a customizable time frame, it helps you revive stale deals or scrub them from your pipeline to keep it healthy.
Deals without tasks: The report surfaces opportunities without associated activities, so you can ensure every deal in your pipeline has a clear next step and is actively being pursued.
From insight to action: Using the report for immediate forecast improvement
The Data Integrity Report's greatest strength isn't just in identification – it's in action:
One-click updates let reps fix data issues directly from the report.
Bulk actions enable operations to quickly address systemic problems.
Trend analysis helps you spot recurring issues, so you can improve processes and training.
By addressing these issues quickly, sales teams can see forecast accuracy improve by 20% or more in the first quarter.
How to clean data boosts your entire sales operations
Improving data quality isn't just about fine-tuning your forecasts – though that alone is worth it. It's about creating a positive feedback loop that improves every part of your sales operation. Addressing duplicate data is critical in this process, as it can cause a host of issues like flawed analysis and inefficient workflows. Let's talk about how clean data can benefit your entire sales ecosystem.
Forecasting accuracy: Making decisions with accurate sales forecasts
When your data is clean and complete, your forecasts aren't guesses – they're predictions of future performance. This level of accuracy unlocks many benefits:
Resource allocation: With predictions of future revenue, you can make informed decisions about hiring, training, and resource deployment.
Strategic planning: Accurate forecasts let you set realistic goals and develop strategies based on data, not hope.
Investor relations: For public companies or those seeking funding, your ability to predict and hit revenue targets can impact valuation and investor confidence.
Check the accuracy of your sales forecasts with our Forecast Accuracy Calculator — ensure your predictions align with reality using Forecastio.
Sales team productivity: Focus on selling, not data management
Clean data isn't just a boon for leadership; it helps your entire sales team by:
Reduced administrative burden: Automated data integrity checks and alerts free reps from data entry drudgery to focus on what matters – selling.
Enhanced opportunity prioritization: When every deal in the pipeline is current, reps can focus on true sales priorities.
More effective coaching: Managers can coach to pipeline data, not data fantasies.
Cross-functional alignment: Finance, Sales, and Operations in Sync
Clean sales data provides a single source of truth for your entire organization:
Finance: Accurate revenue predictions help with budgeting and cash flow planning.
Marketing: Visible pipeline insights help marketing optimize programs and measure ROI.
Customer success: With visibility into incoming deals, customer success can prepare for onboarding and resource allocation.
Deal Velocity: Clean data as a fuel for faster closes
Believe it or not, clean data can actually help you close deals faster:
Improved follow-up: When every deal has tasks and up-to-date info, nothing falls through the cracks.
Enhanced buyer engagement: Reps with accurate, current information can better engage with prospects and address their needs.
Sales process optimization: By analyzing clean pipeline data, you can spot sales process bottlenecks and optimize for faster conversions.
The data quality imperative: Your path to forecasting success
As we've discussed throughout this post, the quality of your sales data isn't a technical issue – it's a strategic imperative that can make or break your organization. Bad data quality creates inaccurate forecasts, erodes trust with stakeholders, and holds your entire sales operation back from achieving its full potential.
The good news is: that you can turn data quality into a competitive advantage with the right approach and tools. Here's your roadmap to forecasting success:
Admit you have a problem: Identify the "Five Horsemen of the Data Apocalypse" infecting your sales data. Conduct a data quality audit.
Create a data culture: Make data quality a team sport. Train, incentivize, and implement processes to prioritize good data hygiene.
Take advantage of technology: Leverage tools like Forecastio's Data Integrity Report to automate data quality checks and simplify maintaining high standards for your team.
Take action: Don't just diagnose data issues – fix them. Use insights from your data quality tools to make immediate changes and implement long-term process improvements.
Monitor and adjust: Data quality isn't a one-and-done task. Regularly monitor your data health and be prepared to adjust processes as your business changes.
In the age of AI-driven sales, predictive analytics, and machine learning, organizations that master data quality will have a secret sauce. They won't just forecast better – they'll be able to maximize the benefits of advanced technologies to supercharge sales performance.
The question isn't can you afford to invest in data quality? In today's competitive market, it's: can you afford not to?
Your roadmap to forecasting success starts with one simple step: evaluate your current data quality.
Schedule a demo with Forecastio today to assess the quality of your HubSpot data. Make sure yours is conveying the right message.
Sales forecasting is one of those tasks that sales teams perform regularly, but it's so much more important than that. Forecasting is the backbone of your revenue recognition, and it's how executives make strategic decisions about the direction of the company.
But what happens when your forecast is wrong? It's often because your data is bad. And bad data leads to bad forecasts, missed quotas, and wasted time and money.
In this article, we'll explore how accurate data impacts your forecasts, how bad data holds you back, and what you can do to fix this common sales forecasting problem.
What is sales forecasting?
Sales forecasting is the process of estimating future sales revenue over a set period. It's an art and a science, using historical sales data, market trends, and other factors to make an educated guess about future sales. Accurate data is crucial for retaining existing customers and earning their loyalty. Without it, you're sailing a ship without a map or compass.
Using statistical models, machine learning algorithms, and expert judgment, companies can predict the probability of future sales and revenue. This is critical for making decisions about production, inventory, pricing, and resource allocation. Sales forecasting is the foundation of strategic planning, and it guides your company toward success.
Why sales forecasting matters
Why is sales forecasting so important? It's the foundation of all business decision-making. Your sales and marketing teams need accurate data to succeed, so precise forecasts are critical. Accurate sales forecasts give you the ability to:
Set realistic sales goals and quotas: Knowing what to shoot for keeps reps motivated and focused.
Manage resources: From staff to inventory, knowing what to expect in terms of future sales helps you plan.
Make informed product and pricing decisions: If you know what's selling and what's not, you can make strategic decisions about product lines and pricing.
Plan for inventory and production: If you know what to expect in terms of future sales, you can plan for inventory and production.
Determine revenue recognition: Accurate sales forecasts give you the ability to recognize revenue and make financial projections.
Guide strategic business decisions: Sales forecasting is the backbone of strategic planning, and it guides your company toward success.
Manage cash flow and budgeting: Knowing what to expect in terms of revenue helps you plan for cash flow and budgeting.
Plan for staffing and training: Knowing if you'll experience sales growth helps you hire and train the right people at the right time.
Determine opportunities for growth and expansion: Trends and patterns can reveal new markets or products.
Mitigate risks and uncertainties: Knowing what to expect helps you prepare for downturns.
In short, sales forecasting isn't a nicety; it's a necessity if you want your business to succeed.
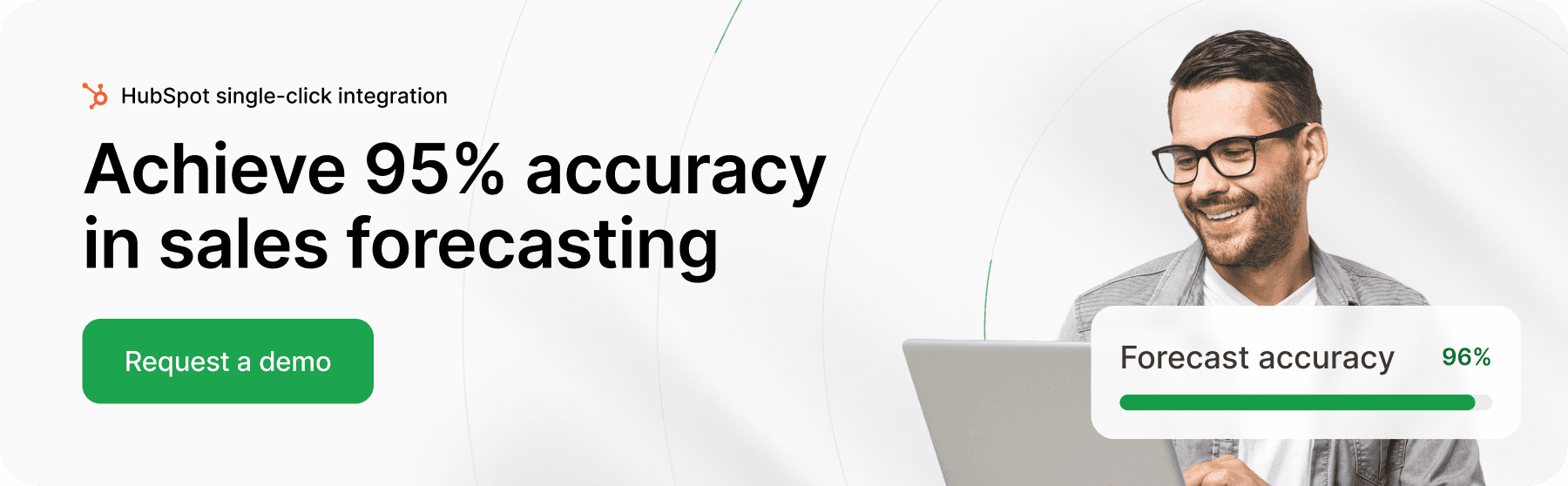
Sales forecasting process overview
The sales forecasting process is a methodology that outlines the steps you should take to forecast sales. Here's an overview of the process:
Data collection: This is where you gather historical sales data, market trends, and other relevant information. This is the fuel for your forecast. It's critical to address duplicate data at this stage to ensure data quality and prevent garbage-in, and garbage-out analysis.
Data analysis: Once you have your data, you need to analyze it to discover trends, patterns, and correlations. This is where you'll learn what drives sales.
Model selection: Select a forecasting model based on your data and business needs. This could be a simple moving average or a complex machine-learning model.
Forecasting: Use your model to forecast sales. This is where the magic happens, and your data becomes a valuable forecast.
Review and revision: Finally, you need to review and revise your forecast regularly. Sales forecasting isn't a one-and-done task; it's an ongoing process.
By following these steps, you can create a solid sales forecast that guides your strategy and drives growth.
The illusion of accuracy: The data quality crisis
The end of the quarter. Your sales team has worked tirelessly, and your CRM is full of numbers. Your forecast looks great. You present it to the board, and at the 11th hour, deals fall through or come in from left field. Sound familiar?
You're not alone. Many sales leaders are tempted by the wealth of data at their fingertips and assume quality when they see quantity.
But the truth is that in sales forecasting, bad data – aka dirty data – is often worse than no data at all. Accurate sales forecasting is critical for business planning and resource allocation, and overcoming common sales forecasting challenges is key to success.
The effects of poor data quality go far beyond inaccurate forecasts. It's a silent killer that erodes trust, misallocates resources and can impact your company's valuation. According to IBM, poor data quality costs the U.S. economy approximately $3.1 trillion each year. For sales teams, it means missed opportunities, wasted time and money, and a cycle of chasing instead of strategizing.
The five horsemen of the data apocalypse
Let's talk about the top five data quality issues that impact your sales forecasts and revenue recognition. We call them the "Five Horsemen of the Data Apocalypse" – dramatic, we know, but they're apocalyptic for your revenue recognition.
One of the biggest problems is simply poor-quality data. We're talking about bad data, but it goes by many names: sloppy data, poor data, lousy data. Whatever you call it, it's a serious problem that can severely impact operational effectiveness in B2B sales and marketing.
1. The ghost deal: Opportunities without amounts
How can you forecast your expenses without knowing how much money you'll make? Ridiculous, right? Yet many sales pipelines are haunted by these "ghost deals" – opportunities that are in the system, but lack one critical detail: the deal amount.
These ethereal deals skew your entire forecast. They could be $10,000 or $100,000 deals, but without an amount, you can't tell. As a result, sales leaders are forced to ignore these opportunities entirely (potentially leaving real revenue on the table) or make educated guesses about the value (introducing huge inaccuracies into the forecast). To forecast future sales accurately, you need detailed records and advanced forecasting methods.
2. The timeless wonder: Deals without close dates
In sales forecasting, timing is everything. A deal that's expected to close this quarter is much different from one that's expected to close next year. Accurate sales forecasting is all about having good data to create solid sales projections. Enter the "Timeless Wonders" – opportunities that are missing a critical piece of information: a close date.
These dateless deals can't be used to create time-based forecasts. They pad your pipeline and can skew your short-term or long-term forecasts. They often indicate a lack of qualification or next steps, exposing deeper issues in your sales process.
3. Walking Dead deals: The deals that constantly slip
We've all been there: a deal that's slipping from quarter to quarter. These "Eternal Sliders" are the bane of accurate forecasting. They make your pipeline look healthy, but your forecast is another story altogether.
Slipping deals often indicate a deeper problem: perhaps your value proposition isn't strong enough, decision-makers aren't engaged, or your timeline isn't aligned with the customer's. Historical sales data can help you identify these sliders, and it's critical for both accurate forecasting and fixing your sales process.
4. The fossil record: Deals that won't budge
Every pipeline has them: opportunities that are fossilized in your pipeline after months (or years). These "Fossil Records" are often the leftovers of overzealous sales reps or changes in the buyer's situation that weren't updated in the system.
Stale deals pad your pipeline and skew your sales metrics, such as average deal size and sales cycle length. They can also mask real issues in your sales process, like poor follow-up or inadequate qualification criteria.
Most importantly, they give a false impression of future revenue potential, introducing huge inaccuracies into your forecast and resource allocation. The right sales forecasting methods are critical – not all methods are right for every business.
5. The lone wolf: Deals without tasks or activities
Sales is an ongoing process. An opportunity without tasks or activities is a plant without water – it's not going anywhere. Using sales forecasting software can help you forecast more accurately and efficiently by leveraging historical data and market trends.
It also provides actionable intelligence and real-time visibility to inform your sales strategies. These "Lone Wolves" are often ignored in your forecast, introducing blind spots into your projections.
A lack of tasks or activities usually indicates the deal isn't being actively pursued. Perhaps the sales rep is overwhelmed, the opportunity was deprioritized, or CRM hygiene is poor. Whatever the reason, these leads introduce huge uncertainty into your forecast, and they're likely wasting away in your pipeline.
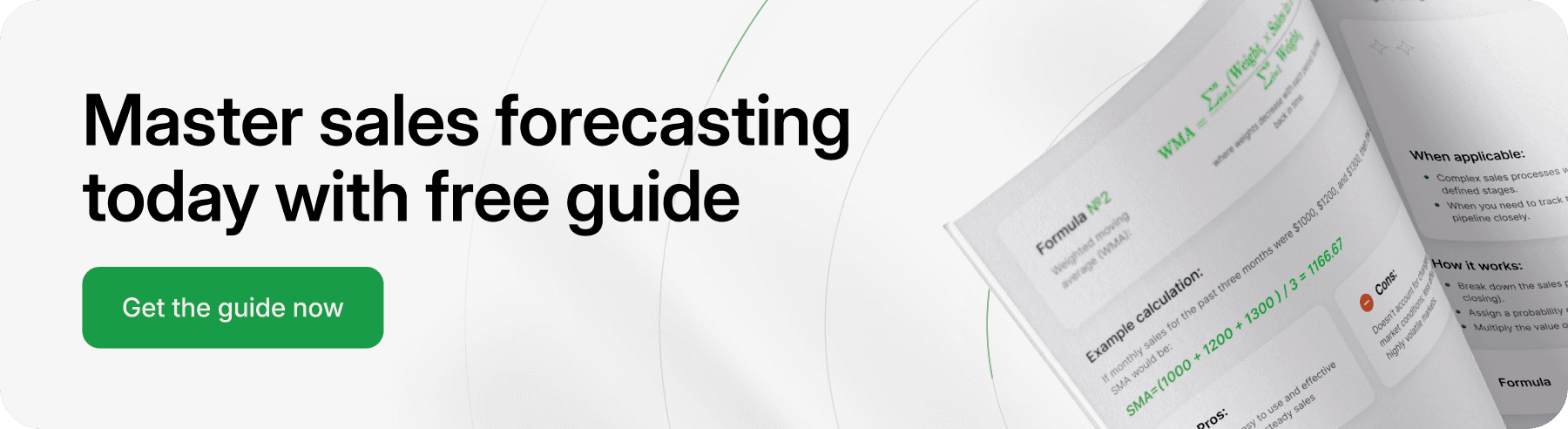
Sales forecast factors
Internal factors: Hires and fires, policy changes, territory shifts
Internal factors can impact sales forecasts in big and small ways. Let's talk about some of those internal factors:
Hires and fires: Changes to the sales team can ripple through sales and forecasting. Bringing in new reps can give you a sales boost while losing a top performer can be hard to recover from.
Policy changes: Changes to company policy, such as pricing strategy or commission structure, can impact sales directly. Aggressive pricing can drive more sales while changing the commission structure can motivate or demotivate your sales team.
Territory shifts: Reassigning territories or changing sales assignments can break relationships and impact sales. A well-planned territory reassignment can help you cover more ground and drive more sales, but a poorly executed change can confuse customers and cost you deals.
These internal factors are impacted by:
Sales team performance and morale: A motivated and supported sales team will hit quota and maintain good data quality.
Training and development programs: Ongoing training and development help sales reps fine-tune their skills and improve forecasting accuracy.
Sales process and methodology: A defined sales process ensures consistency and reliability in data collection and forecasting.
Compensation and incentive structures: Aligning incentives with company goals leads to better performance and more accurate data entry.
Company culture and values: A culture that values data integrity and transparency will give you better data quality and more accurate forecasts.
By considering these internal factors, you can improve forecasting accuracy and make better decisions about your business. Acknowledging and addressing these issues will transform sales forecasting from a problem into a competitive advantage.
Connecting the dots: How data gaps create forecast gaps
We've talked about the "Five Horsemen of the Data Apocalypse," but how do these data quality issues – including missing data – impact your sales forecasts?
The butterfly effect: Small data inconsistencies, big forecast deviations
In chaos theory, the butterfly effect suggests that small changes can have drastic effects. It's the idea that a butterfly flapping its wings can cause a hurricane to avoid New York City. In sales forecasting, the same principle applies. One deal without an amount or close date might not seem like a big deal, but when you multiply it by hundreds or thousands of opportunities, it becomes a perfect storm of inaccuracies.
Here's an example: if 10% of your opportunities are missing amounts and your average deal size is $100,000, a pipeline of 1,000 deals could be undervalued by $10 million. That's not a rounding error; it's a gap that can swallow your quarterly quota whole.
The ripple effect across departments: How bad data spreads across the organization
Your sales forecast isn't an island unto itself. It impacts decisions across the entire business:
Finance relies on it for budgeting and investor relations.
Operations uses it for resource allocation and capacity planning.
Marketing uses it to evaluate campaign effectiveness and plan future initiatives.
When your sales forecast is built on bad data, it creates a domino effect of poor decisions across the company. Marketing may pour more budget into campaigns that fail to produce qualified, high-value opportunities. Operations might overhire or understaff, incurring unnecessary costs or struggling to deliver. Finance could make critical mistakes in cash flow projections, affecting everything from hiring to strategic investments.
The credibility killer: Losing trust with stakeholders due to fluctuating forecasts
Bad data's worst side effect is loss of trust. When forecasts continue to miss the mark, stakeholders from the board of directors to team leaders stop believing what sales is telling them and lose trust in the organization's ability to forecast and hit numbers.
This lack of credibility can have far-reaching consequences:
Increased scrutiny and micromanaging of the sales process.
Trouble getting resources or budget for sales initiatives.
Strained relationships between sales and other teams.
In publicly traded companies, it can impact stock prices and investor confidence.
Trust is tough to regain; it's much easier to fix the root cause – bad data – than to continuously apologize for missed forecasts.
The human factor: Why sales teams can't get data quality right
Before we get to solutions, let's talk about why these data quality issues persist. After all, no sales rep is trying to intentionally run up inaccurate forecasts. One big reason is outdated data, which leads to missed opportunities and poor outcomes. The root causes are surprisingly human:
The time crunch: Data entry vs closing deals
Sales reps are under intense pressure to hit quota. Closing deals takes priority over data entry. It's the urgent versus the important. Reps think, "I'll get to that later," but later never arrives.
The optimism bias: When hope trumps data integrity
Salespeople are naturally optimistic – it's a job requirement. However, that optimism can translate to overly optimistic forecasts. Reps might keep stale deals in the pipeline, thinking "This could still close," or they might assign overly aggressive close dates.
The knowledge gap: Not understanding how individual data hygiene impacts the bigger picture
Many sales reps don't understand how their data hygiene practices impact the overall forecast. They might not know that omitting a deal amount or not updating a close date can add up to millions of dollars in forecast inaccuracies when rolled up from the team level.
Breaking the cycle: How to achieve data quality
Solving these problems requires a multi-pronged approach:
A key component is ensuring your email list quality is top-notch. Poor email list quality can lead to spam filters flagging your emails, damaging your reputation with internet service providers, and missing opportunities to engage with interested prospects.
Data-driven culture: Make quality a team sport
Data quality is everyone's responsibility, from your newest Sales Development Representative (SDR) to your Chief Revenue Officer. This means:
Including data quality metrics in rep performance reviews.
Rewarding good data hygiene as much as closed deals.
Offering regular training on the importance of accurate data.
Gamification of data integrity: Make data entry fun
Why not make data entry fun? Try:
Leaderboards for the most accurate forecasters.
Rewards for teams with the cleanest data.
Challenges or sprints focused on fixing specific data problems.
Empower your sales team — use our Sales Rep Commission Calculator to quickly calculate commissions and ensure fair, transparent payouts.
Automation: Reducing human error with smart systems
This is where technology can be a game-changer. Advanced CRM systems and AI-powered tools can:
Automatically alert you to data inconsistencies.
Predict realistic close dates based on historical trends.
Remind reps to follow up on stale opportunities.
The data integrity report: Your crystal ball for forecast clarity
This is where Forecastio's proprietary Data Integrity Report comes in. Designed to tackle the "Five Horsemen" head-on, it gives you unprecedented visibility into your data quality issues.
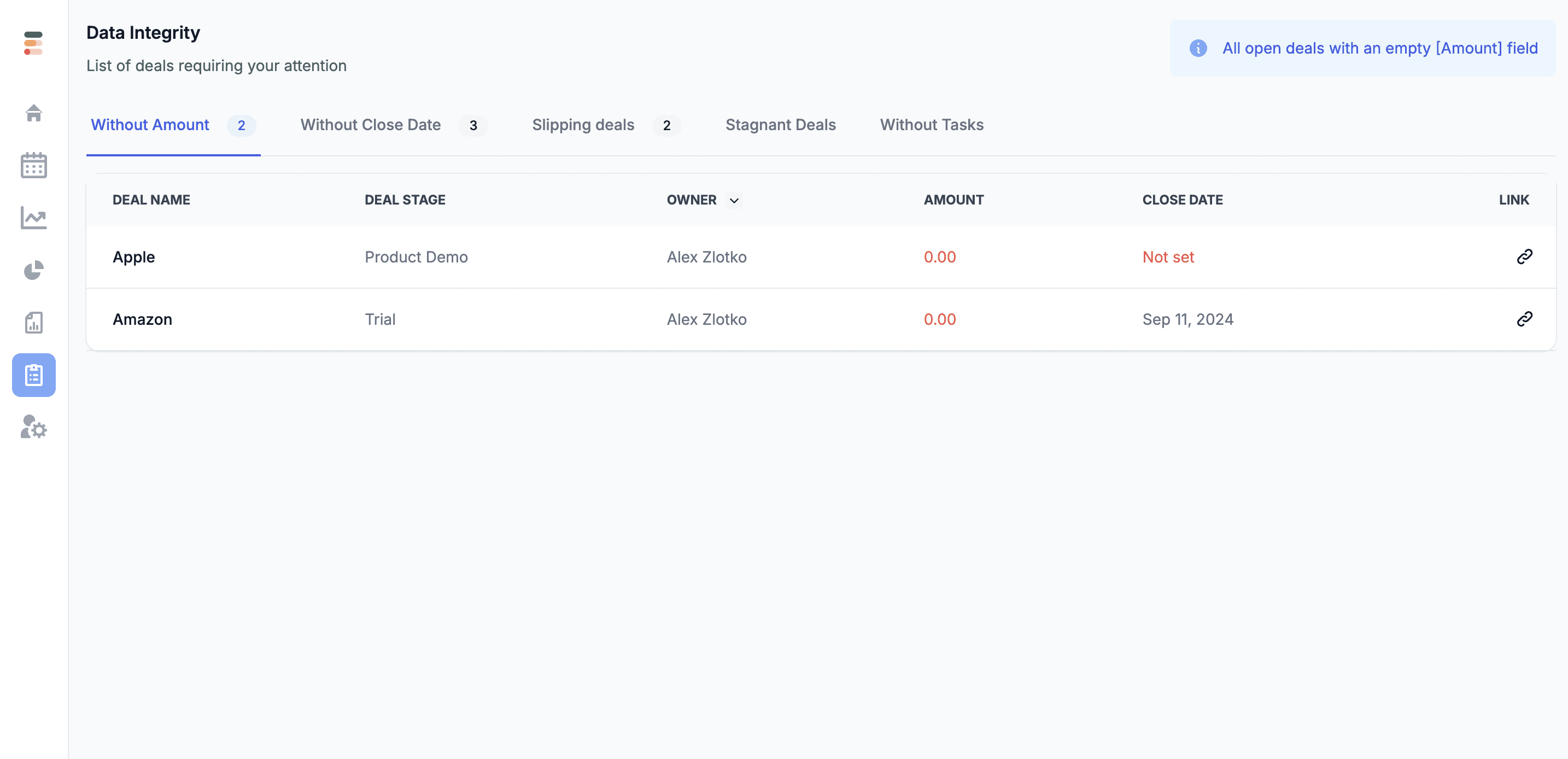
Introducing Forecastio's feature
The Data Integrity Report isn't just another dashboard – it's a diagnostic tool that scans your sales data for critical issues impacting forecast accuracy. It's like having a team of data analysts pour over your CRM, but automated and in real-time.
Drill down into the 5 critical Data Issues it finds
Deals without amounts: The report flags every opportunity without a monetary value, so you can quantify the unquantified and fill in the blanks.
Missing close dates: The report shines a light on deals floating in sales limbo, giving you clarity on your pipeline and enabling period-based forecasting.
Slipping deals: The system flags opportunities that have had their close dates pushed multiple times, so you can address sales momentum issues before it's too late.
Stagnant deals: By surfacing opportunities with no activity within a customizable time frame, it helps you revive stale deals or scrub them from your pipeline to keep it healthy.
Deals without tasks: The report surfaces opportunities without associated activities, so you can ensure every deal in your pipeline has a clear next step and is actively being pursued.
From insight to action: Using the report for immediate forecast improvement
The Data Integrity Report's greatest strength isn't just in identification – it's in action:
One-click updates let reps fix data issues directly from the report.
Bulk actions enable operations to quickly address systemic problems.
Trend analysis helps you spot recurring issues, so you can improve processes and training.
By addressing these issues quickly, sales teams can see forecast accuracy improve by 20% or more in the first quarter.
How to clean data boosts your entire sales operations
Improving data quality isn't just about fine-tuning your forecasts – though that alone is worth it. It's about creating a positive feedback loop that improves every part of your sales operation. Addressing duplicate data is critical in this process, as it can cause a host of issues like flawed analysis and inefficient workflows. Let's talk about how clean data can benefit your entire sales ecosystem.
Forecasting accuracy: Making decisions with accurate sales forecasts
When your data is clean and complete, your forecasts aren't guesses – they're predictions of future performance. This level of accuracy unlocks many benefits:
Resource allocation: With predictions of future revenue, you can make informed decisions about hiring, training, and resource deployment.
Strategic planning: Accurate forecasts let you set realistic goals and develop strategies based on data, not hope.
Investor relations: For public companies or those seeking funding, your ability to predict and hit revenue targets can impact valuation and investor confidence.
Check the accuracy of your sales forecasts with our Forecast Accuracy Calculator — ensure your predictions align with reality using Forecastio.
Sales team productivity: Focus on selling, not data management
Clean data isn't just a boon for leadership; it helps your entire sales team by:
Reduced administrative burden: Automated data integrity checks and alerts free reps from data entry drudgery to focus on what matters – selling.
Enhanced opportunity prioritization: When every deal in the pipeline is current, reps can focus on true sales priorities.
More effective coaching: Managers can coach to pipeline data, not data fantasies.
Cross-functional alignment: Finance, Sales, and Operations in Sync
Clean sales data provides a single source of truth for your entire organization:
Finance: Accurate revenue predictions help with budgeting and cash flow planning.
Marketing: Visible pipeline insights help marketing optimize programs and measure ROI.
Customer success: With visibility into incoming deals, customer success can prepare for onboarding and resource allocation.
Deal Velocity: Clean data as a fuel for faster closes
Believe it or not, clean data can actually help you close deals faster:
Improved follow-up: When every deal has tasks and up-to-date info, nothing falls through the cracks.
Enhanced buyer engagement: Reps with accurate, current information can better engage with prospects and address their needs.
Sales process optimization: By analyzing clean pipeline data, you can spot sales process bottlenecks and optimize for faster conversions.
The data quality imperative: Your path to forecasting success
As we've discussed throughout this post, the quality of your sales data isn't a technical issue – it's a strategic imperative that can make or break your organization. Bad data quality creates inaccurate forecasts, erodes trust with stakeholders, and holds your entire sales operation back from achieving its full potential.
The good news is: that you can turn data quality into a competitive advantage with the right approach and tools. Here's your roadmap to forecasting success:
Admit you have a problem: Identify the "Five Horsemen of the Data Apocalypse" infecting your sales data. Conduct a data quality audit.
Create a data culture: Make data quality a team sport. Train, incentivize, and implement processes to prioritize good data hygiene.
Take advantage of technology: Leverage tools like Forecastio's Data Integrity Report to automate data quality checks and simplify maintaining high standards for your team.
Take action: Don't just diagnose data issues – fix them. Use insights from your data quality tools to make immediate changes and implement long-term process improvements.
Monitor and adjust: Data quality isn't a one-and-done task. Regularly monitor your data health and be prepared to adjust processes as your business changes.
In the age of AI-driven sales, predictive analytics, and machine learning, organizations that master data quality will have a secret sauce. They won't just forecast better – they'll be able to maximize the benefits of advanced technologies to supercharge sales performance.
The question isn't can you afford to invest in data quality? In today's competitive market, it's: can you afford not to?
Your roadmap to forecasting success starts with one simple step: evaluate your current data quality.
Schedule a demo with Forecastio today to assess the quality of your HubSpot data. Make sure yours is conveying the right message.
Share:
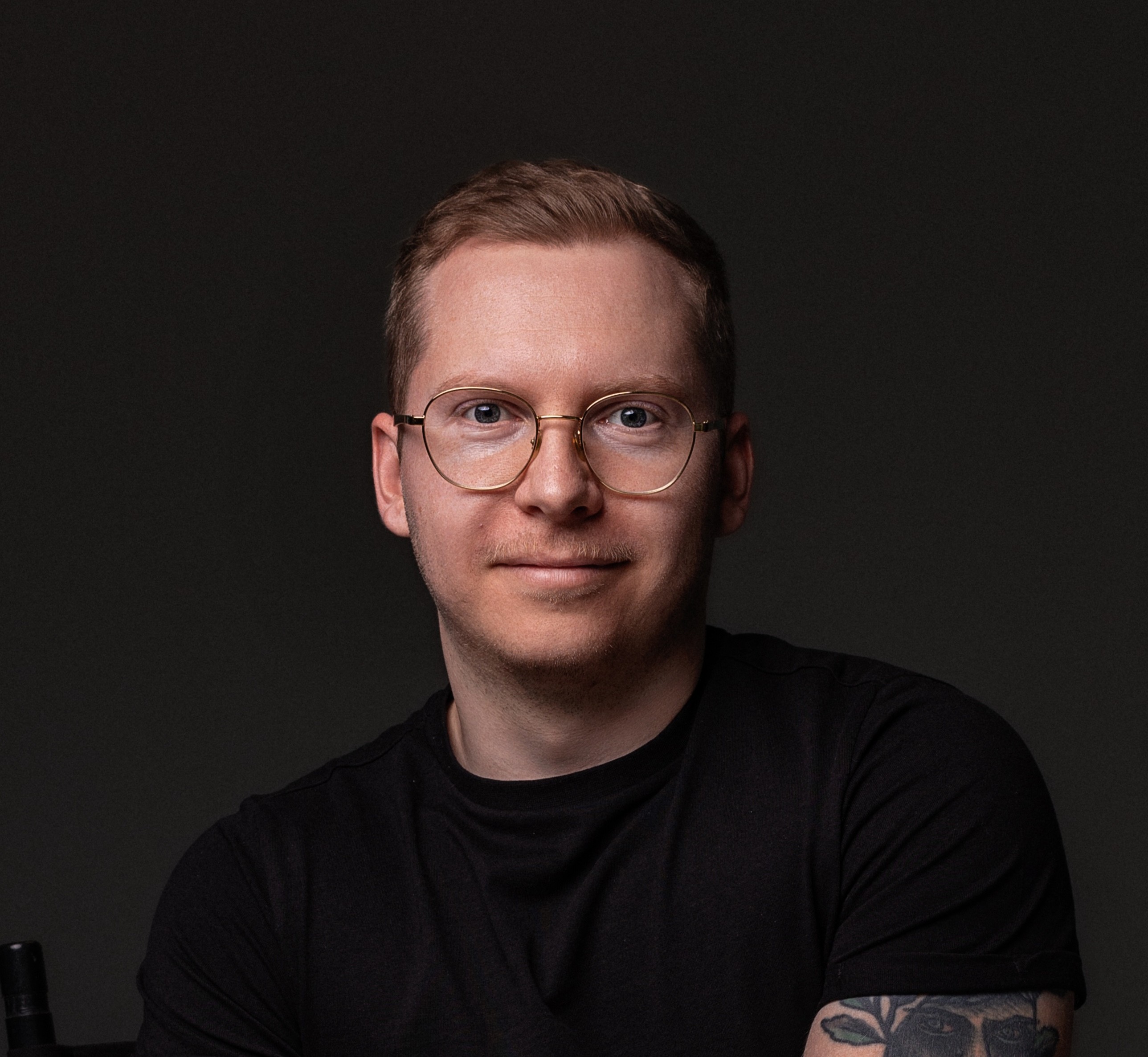
Dmytro is a seasoned marketing professional with over 10 years in the B2B and startup ecosystem. He is passionate about helping companies better plan their revenue goals, improve forecast accuracy, and proactively address performance bottlenecks or seize growth opportunities.
Dmytro is a seasoned marketing professional with over 10 years in the B2B and startup ecosystem. He is passionate about helping companies better plan their revenue goals, improve forecast accuracy, and proactively address performance bottlenecks or seize growth opportunities.
Related articles
Pipeline Management
Feb 17, 2025
9 min
Pipeline Management
Feb 17, 2025
9 min
Sales Performance
Feb 17, 2025
9 min
Sales Performance
Feb 17, 2025
9 min
Sales Strategies
Feb 17, 2025
15 min
Sales Strategies
Feb 17, 2025
15 min
Pipeline Management
Feb 17, 2025
9 min
Sales Performance
Feb 17, 2025
9 min
Pipeline Management
Feb 17, 2025
9 min
Sales Performance
Feb 17, 2025
9 min
Sales Planning
Sales Forecasting
Sales Performance Insights
Sales Planning
Sales Forecasting
Sales Performance Insights
Sales Planning
Sales Forecasting
Sales Performance Insights
© 2025 Forecastio, All rights reserved.
Sales Planning
Sales Forecasting
Sales Performance Insights
Sales Planning
Sales Forecasting
Sales Performance Insights
Sales Planning
Sales Forecasting
Sales Performance Insights
© 2025 Forecastio, All rights reserved.
Sales Planning
Sales Forecasting
Sales Performance Insights
Sales Planning
Sales Forecasting
Sales Performance Insights
Sales Planning
Sales Forecasting
Sales Performance Insights
© 2025 Forecastio, All rights reserved.
Sales Planning
Sales Forecasting
Sales Performance Insights
Sales Planning
Sales Forecasting
Sales Performance Insights
Sales Planning
Sales Forecasting
Sales Performance Insights
© 2025 Forecastio, All rights reserved.