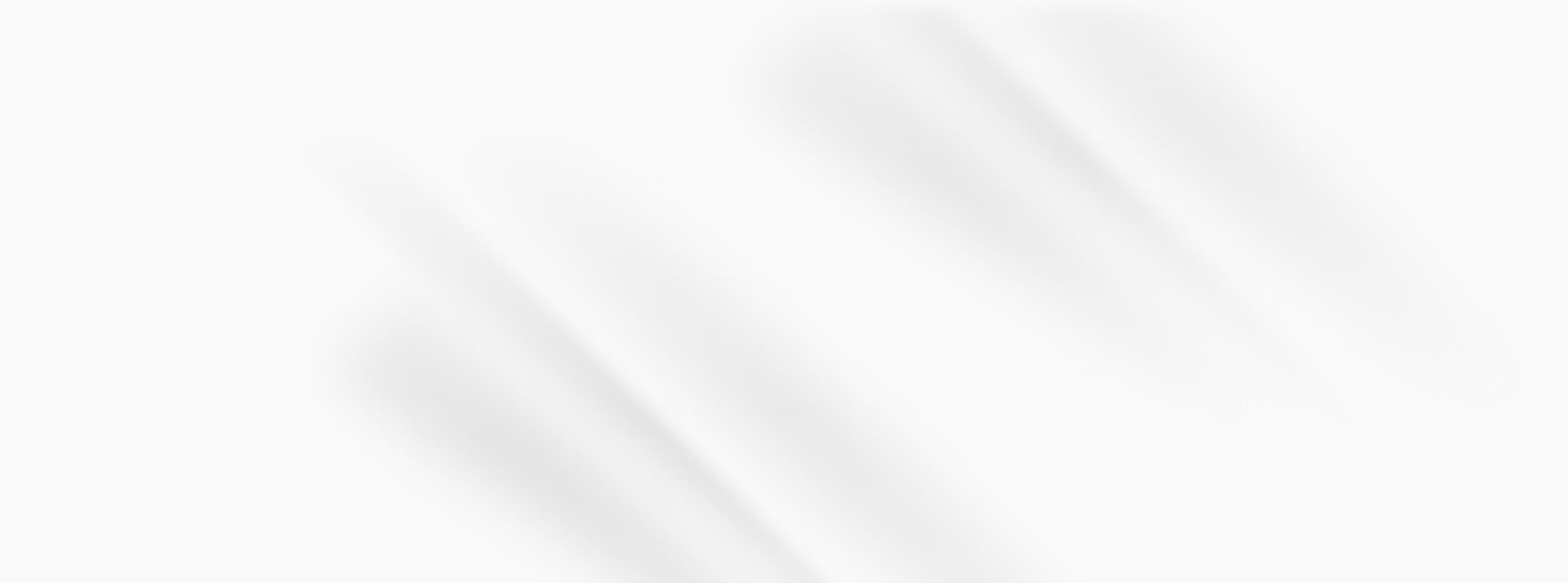
Elevate Your B2B Strategy with AI Sales Forecasting
Feb 17, 2025
Feb 17, 2025
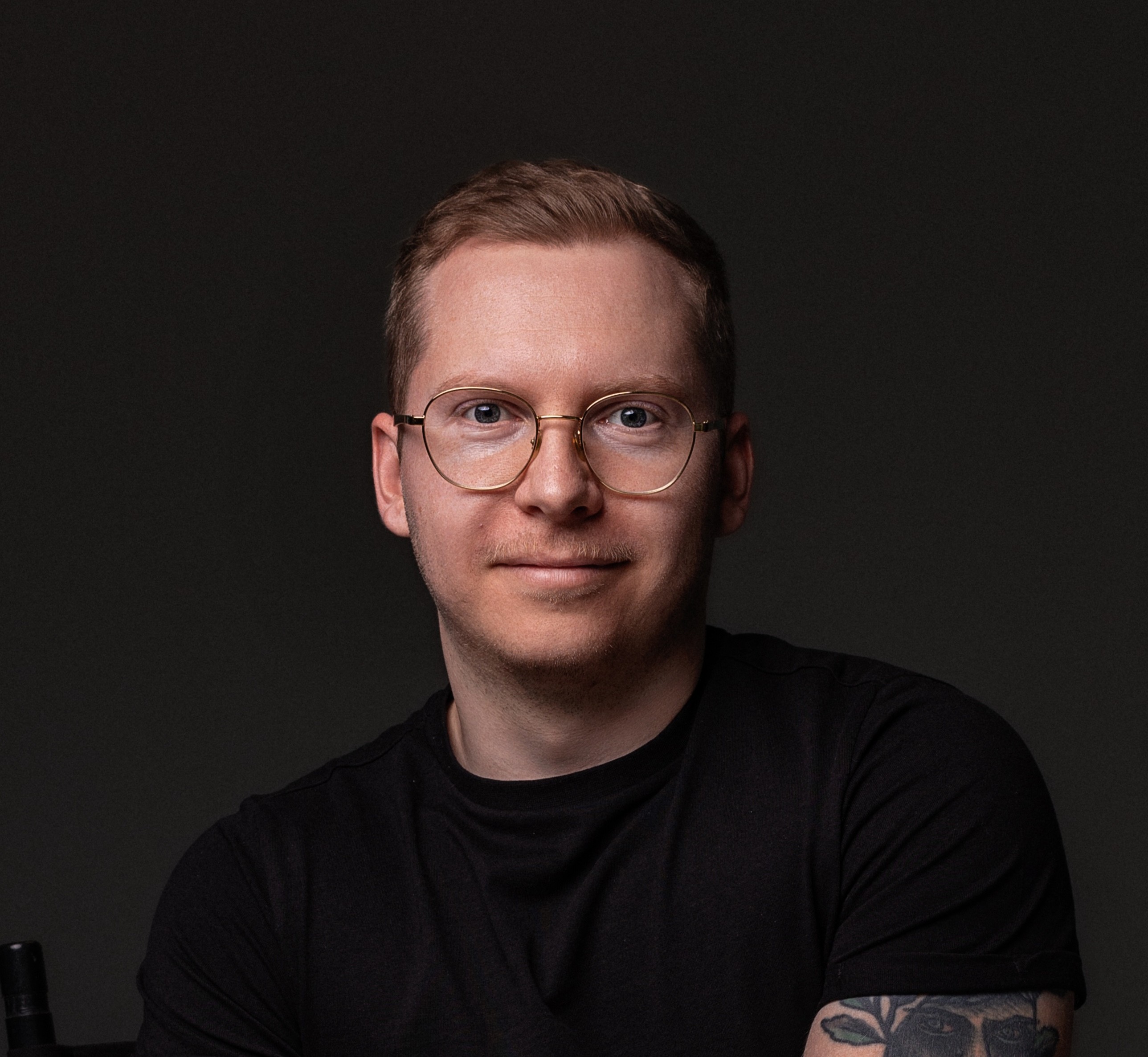
Dmytro Chervonyi
CMO at Forecastio
Last updated
Feb 17, 2025
Reading time
12 min
Share:
Share
Table of Contents




TL;DR
TL;DR
AI-powered sales forecasting cuts prediction errors by 50% and reduces forecasting time by 80% compared to traditional methods.
The system analyzes data from emails, calls, and CRM entries to spot which deals will close and which need attention.
Start with a pilot program in one sales team to prove the concept.
Clean your CRM data first and set clear success metrics.
The system auto-scores deals based on closing likelihood and tells you what moves deals through your pipeline fastest.
Your team gets real-time alerts when deals show risk factors, letting them fix problems early instead of relying on gut feel.
AI-powered sales forecasting cuts prediction errors by 50% and reduces forecasting time by 80% compared to traditional methods.
The system analyzes data from emails, calls, and CRM entries to spot which deals will close and which need attention.
Start with a pilot program in one sales team to prove the concept.
Clean your CRM data first and set clear success metrics.
The system auto-scores deals based on closing likelihood and tells you what moves deals through your pipeline fastest.
Your team gets real-time alerts when deals show risk factors, letting them fix problems early instead of relying on gut feel.
In the B2B world where everything moves fast and changes fast, being able to predict outcomes and navigate the sales landscape is not a nice to have, it’s a must-have. At the heart of this is sales forecasting, a process that gives you invaluable insights to make smarter data-driven decisions. But despite how important it is, many companies are still using inaccurate and inefficient forecasting methods that don’t meet their strategic needs.
This guide explores the impact of Artificial Intelligence (AI) on sales forecasting so sales leaders can transform their sales operations and stay ahead of the curve in a complex market.
Sales forecasting 101: A business imperative
Before we get into the AI bit, let’s first understand what sales forecasting is and why it’s so important to business.
What is sales forecasting?
Sales forecasting is the process of predicting future sales performance based on past sales data, historical data, market trends and other relevant factors. It’s a business function that helps companies:
Forecast revenue and budget
Allocate resources
Set targets
Decide on inventory, staffing and expansion
Why accurate sales forecasts matter
Accurate sales forecasts are more than just numbers—they’re the building blocks for many strategic decisions:
Financial Planning: Forecasts inform budgeting, investment decisions and financial projections.
Resource Management: They guide inventory levels, production schedules and workforce planning.
Risk Management: Accurate forecasts help identify market downturns or opportunities so you can adjust your strategy proactively.
Performance Management: They provide a benchmark to measure actual sales performance.
Investor Relations: Reliable forecasts build trust with stakeholders and investors.
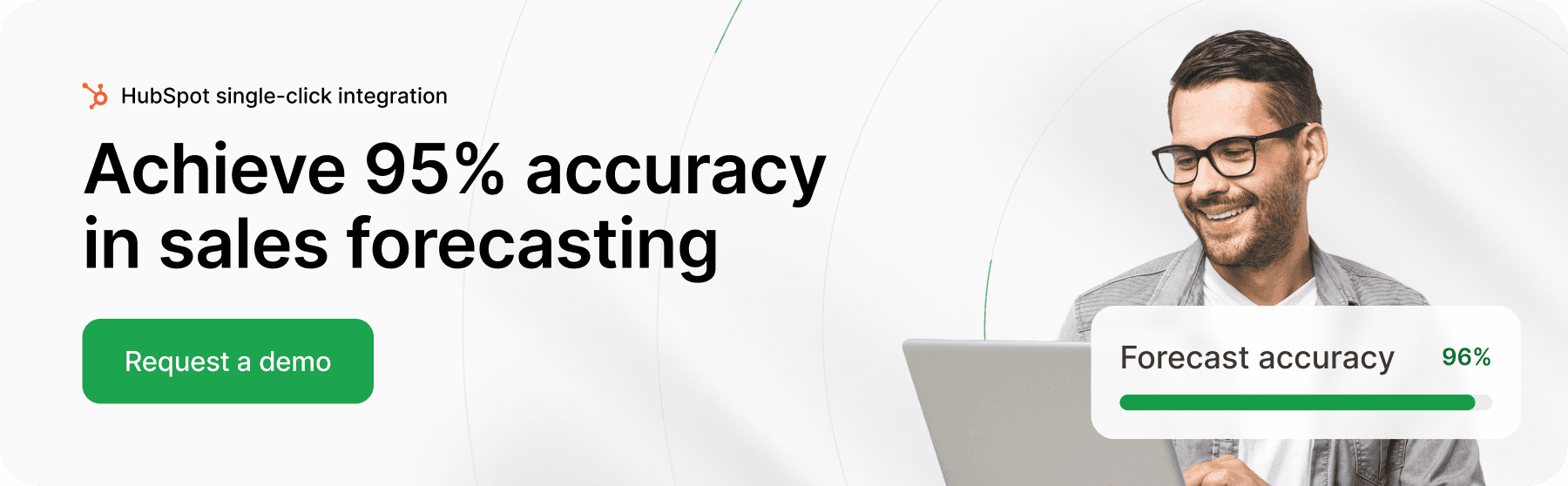
Given how important it is, you’d think sales forecasting would be a well-oiled machine in most companies. But reality is far from it.
The consequences of inaccurate forecasts on sales performance
Inaccurate sales forecasts can really hold back sales performance and create a domino effect of negative outcomes. When sales forecasts are wrong, companies end up with overstocking or understocking issues which means lost sales opportunities or wasted resources. Misallocated resources like misdirected sales teams and misaligned marketing budgets only make it worse.
Bad decisions from bad forecasts mean missed opportunities and lost revenue. Customer satisfaction suffers as sales teams struggle to meet demand accurately. This, in turn, demoralizes sales teams and leads to frustration and disappointment.
On the other hand, accurate sales forecasts enable sales teams to make informed decisions, allocate resources and meet customer demand, which means better sales performance. By using precise sales forecasts companies can optimize their operations, improve customer satisfaction, and drive revenue growth.
Sales forecasting evolution: From guesswork to data science
To understand the impact of AI on sales forecasting, let’s look at the journey from traditional methods to modern techniques.
Traditional sales forecasting methods: A historical perspective
Intuition-Based Forecasting
Relying on sales managers’ gut feel and experience
Subjective and biased
Not scalable and not consistent across large companies
Simple Trend Projections
Using basic mathematical models to extrapolate future sales from historical data
Assuming linear trends and stable market conditions
Not accounting for complex market dynamics and sudden changes
Evaluating opportunities at different stages of the sales funnel
Applying probability percentages to estimate likely closures
More accurate but still relying on subjective assessments
Time Series Analysis
Using more advanced statistical methods to identify patterns in historical data
Including seasonality and cyclical trends
Limited ability to factor in external variables and market changes
Drawbacks of traditional forecasting methods
While these traditional methods were the foundation of sales forecasting, they have significant limitations in today’s fast paced, data driven business world:
No Real-Time Adaptability
Traditional forecasts don’t account for sudden market changes or emerging trends
Updates are periodic which means outdated projections
Human Bias and Subjectivity
Forecasts can be influenced by individual perspectives which means overly optimistic or pessimistic projections
Inconsistency across different forecasters or departments
Limited Data Integration
Traditional methods can’t integrate multiple data sources
Siloed data means incomplete or fragmented forecasts
Time Consuming Processes
Manual data entry and analysis slows down the forecasting process
Delays in getting forecasts mean missed opportunities or delayed decisions
Scalability Issues
As companies grow, traditional methods become more cumbersome and error-prone
Can’t maintain consistency across larger and more complex sales organizations
Can’t Handle Multiple Variables
Traditional methods struggle with multi-variable analysis
Can’t account for external factors like economic conditions, competitive actions, or regulatory changes
Limited Forecasting Ability
Focusing on historical data may not capture emerging trends or disruptors
Can’t provide insights beyond basic sales projection
The consequences of this are severe: misallocated resources, missed revenue opportunities, and reduced operational efficiency. As companies are forced to make decisions in real time using data, the limitations of traditional forecasting methods are becoming more and more apparent. Sales forecasting software is the answer by allowing real-time adjustments, better data analysis, and better decision-making and operational efficiency.
AI: A game changer for sales forecasting
The combination of Artificial Intelligence with sales forecasting is a giant leap forward, addressing the limitations of traditional methods and introducing new possibilities. AI driven sales forecasting uses advanced algorithms, machine learning, and big data analytics to transform the accuracy, speed, and depth of sales predictions.
AI driven sales forecasting components
Machine Learning Algorithms
Uses complex statistical models to find patterns and relationships in data
Learns and improves predictions with new information
Natural Language Processing (NLP)
Analyzes unstructured data from emails, call logs, and social media
Extracts insights from customer interactions and market sentiment
Big Data Analytics
Processes multiple data sources in real-time
Finds correlations and trends in customer data that would be impossible to find manually
Predictive Analytics
Goes beyond historical analysis to predict future outcomes
Provides probability-based predictions for individual deals and overall sales performance
Prescriptive Analytics
Gives recommendations to improve sales outcomes
Suggests strategies based on forecasted scenarios
How AI changes the forecasting process
Real-Time Data Processing
AI systems process vast amounts of data in real-time and provide instant forecasts
Responds to changing market conditions quickly
Advanced Pattern Recognition
Machine learning algorithms find complex patterns and correlations that human analysts can’t
Uncovers hidden insights that drive better predictions
Multi-Variable Analysis
AI can process many variables: macro trends to individual customer behaviour
Gives a 360 degree view of what’s driving sales performance
Automated Data Collection and Integration
AI tools collect and integrate data from multiple sources
No manual data entry errors and complete analysis
Personalised Forecasting
Forecasts at individual, team, product, and company level
Forecasts tailored to specific sales roles, territories, or customer segments
Scenario Modelling
Allows sales leaders to simulate different scenarios and their outcomes
Better planning and risk management
Data processing, enrichment, and analysis for future sales
Data processing, enrichment, and analysis are key to accurate sales forecasting. Using advanced technologies like AI and ML, sales teams can process large amounts of data quickly and efficiently. Enriching this data with external sources like market trends and customer behavior gives a complete view of the sales landscape.
Analyzing this enriched data allows sales teams to find patterns and trends that will inform future sales. This data-driven approach allows sales teams to forecast sales more accurately and manage the sales pipeline better. By using AI and ML businesses can turn raw data into actionable insights and get more precise sales forecasts and better decision making.
AI in sales forecasting: The benefits
Integrating AI in sales forecasting brings many benefits that can impact the bottom line and competitive position.
1. Unmatched accuracy
AI eliminates human error and bias, using large datasets and complex algorithms to produce accurate forecasts. This accuracy gives more confidence in decision-making and resource allocation.
Forecast Error Reduction: AI forecasts can reduce error rates by up to 50% compared to traditional methods.
Consistency: AI is accurate across different time horizons, from short-term operational forecasts to long-term strategic projections.
Early Warning Systems: AI can detect subtle signs of forecast deviations and allow for proactive adjustments.
2. Productivity
By automating data collection, analysis, and reporting, AI reduces the time and effort required for forecasting. Sales teams can focus on high-value activities like relationship building and closing deals.
Time Savings: AI can reduce forecasting time by up to 80%, freeing up resources.
Automated Reporting: Get forecast reports with a click, no manual compilation is required.
Real-Time Updates: AI systems can update forecasts in real-time without human intervention.
3. Scalability
AI systems scale with your business and adapt to market changes. This flexibility means your forecasting stays robust and relevant regardless of your company size or market conditions.
Complexity: AI can handle thousands of SKUs or customer segments.
Market Responsiveness: Quickly add new data points or market shifts to forecasts.
Cross-Functional: Easily extend forecasting to different departments and business units.
4. Deeper insights and decision-making
AI finds hidden patterns and correlations in your sales data, providing valuable insights into customer behavior, market trends, and sales performance. This gives sales leaders the ability to make data-driven decisions that drive revenue growth.
Customer Behavior Analysis: Understand what drives buying decisions and predict future customer behavior.
Market Trend Detection: Detect trends before they become obvious, and get first mover advantage.
Performance Driver Analysis: Know what drives sales performance and focus on that.
5. Sales pipeline management
AI powered forecasting tools give you a granular view of your sales pipeline, to improve sales pipeline management by identifying bottlenecks, optimizing deal velocity, and prioritizing high-potential opportunities.
Opportunity Scoring: Auto score deals based on the likelihood of closing.
Stage Progression Analysis: What accelerates or hinders deal progression through the pipeline.
Resource Allocation: Direct sales efforts and resources to the best opportunities.
6. Collaboration and alignment
AI powered forecasting platforms can bring better communication and alignment across sales teams, management, and other departments.
Shared Visibility: One source of truth for sales projections across the company.
Cross-Functional Planning: Better coordination between sales, marketing, finance, and operations.
Goal Setting and Tracking: Set more realistic targets and track them in real-time.
7. Competitive advantage
Use AI in sales forecasting and you’ll be ahead of the competition.
Faster Market Response: Adjust strategies based on real-time forecast updates.
Better Customer Experience: Use predictive insights to anticipate and serve customer needs.
Data Driven Culture: Create an organization that values and acts on data driven insights.
How to implement AI-driven sales forecasting: A step by step approach
Implementing AI into your sales forecasting process requires planning and execution. Here’s a step-by-step guide:
1. Review your current forecasting
Pain Points: Document current issues with accuracy, timeliness, or resource allocation.
Data Quality: Assess the completeness, accuracy, and accessibility of your sales data.
Current Workflows: Map out how forecasting fits into other business processes.
KPIs: Define how you will measure forecast accuracy and impact.
2. Set clear objectives
Specific, measurable goals for your AI driven forecasting project:
Improve Forecast Accuracy: Target percentage improvement in forecast accuracy.
Reduce Forecasting Time: Cut down time spent on forecasting activities.
Better Decision Making: How will improved forecasts inform business decisions.
Increase Win Rates: Target improvement in deal close rates through better opportunity prioritization.
3. Select the right AI forecasting tool
Choose an AI forecasting tool that fits your business and technical landscape:
Integration: Ensure seamless integration with your existing CRM and sales tools.
Scalability: Think about future growth and how the solution will scale with your business.
Customization: Look for platforms that can be configured to your sales process.
User Experience: Prioritize solutions with simple interfaces for broad adoption.
Vendor Expertise: What’s the vendor’s experience in your industry and support options.
4. Data infrastructure
Get your data ready for AI forecasting:
Data Centralization: Consolidate sales data from multiple sources into one place.
Data Cleansing: Put processes in place to clean and standardize historical and incoming data.
Data Governance: Define data management and quality control policies.
Real-Time Data Pipelines: Set up secure data flows for continuous forecast updates.
5. Pilot
Pilot Group: Choose a specific sales team or product line to start with.
Timelines: Define a timeline for the pilot including key milestones and review points.
Metrics: Monitor forecast accuracy, user adoption, and sales performance.
Feedback: Gather feedback from users and stakeholders to identify areas to improve.
Iterative Refining: Use pilot insights to refine the AI models and processes before full rollout.
6. Training and change management
Get your sales team ready for AI forecasting:
Training Programs: Develop role-specific training on the new tools and processes.
Executive Sponsorship: Ensure leadership visibility to drive adoption.
Change Champions: Identify and empower influential team members to promote the new way.
Communication: Address concerns and highlight benefits through ongoing communication.
Incentive Alignment: Adjust metrics and incentives to encourage AI adoption.
7. Monitor and refine
Performance Reviews: Regularly review forecast accuracy and business impact.
Model Retraining: Continuously update AI models with new data.
Stay Up To Date: Keep current with AI forecasting technology and best practices.
User Feedback Loop: Establish a feedback mechanism to drive continuous improvement.
Cross-Functional Collaboration: Regular dialogue between sales, IT, and data science teams to optimize the system.
AI Forecasting implementation challenges
Bringing AI into the sales process can make it more efficient and accurate by automating the mundane, so sales teams can focus on data analysis and market trends.
1. Data quality and format issues
Problem: Poor data quality or incompatible data formats can kill AI forecast accuracy.
Solutions:
Do a data audit to find the issues.
Implement data validation rules in your CRM and other data sources.
Invest in data integration tools to get the data flowing.
Monitor data quality ongoing.
2. Change resistance
Problem: Sales teams may not want to adopt new AI forecasting methods and stick to what they know.
Solutions:
Get key stakeholders involved early in the implementation to get buy-in.
Show clear ROI and benefits through pilot programs and case studies.
Provide ongoing support and celebrate early wins to build momentum.
Address concerns openly and highlight how AI complements not replaces human expertise.
Offer role-specific training to show how AI can make each sales rep more successful.
3. Existing systems
Problem: Integrating AI forecasting tools with existing CRM and sales systems can be tricky.
Solutions:
Choose AI solutions with strong APIs and pre-built integrations.
Work with your IT department to get the integration and data flowing.
Consider a phased rollout to minimize disruption.
Test integrations thoroughly before going live.
Develop a data architecture plan to show how AI forecasting will sit in your tech stack.
4. AI vs human
Problem: Balancing AI forecasts with human judgment can be hard.
Solutions:
Train sales leaders to interpret and act on AI insights.
Encourage data-driven decision-making while valuing human expertise and market knowledge.
Create a feedback loop for sales teams to input into the AI models.
Use AI forecasts as a conversation starter, not a final answer.
Compare AI forecasts with human forecasts regularly to see where both can improve.
5. Cost and ROI
Problem: Justifying the investment in AI forecasting technology can be tough, especially for smaller businesses.
Solutions:
Start with a pilot to show tangible ROI before going full scale.
Calculate the cost savings from better forecast accuracy and efficiency gains.
Consider cloud-based AI forecasting solutions with more flexible pricing models.
Quantify the strategic value of better decision-making enabled by AI insights.
Build a business case that shows both the short and long-term benefits of AI forecasting.
AI in B2B sales forecasting in the future
As AI gets better we can expect even more advanced capabilities in sales forecasting. Here’s a sneak peek into the future of AI driven B2B sales forecasting:
1. Hyper personalized forecasting
AI will enable forecasting at the individual customer level, predicting specific product needs and optimal engagement strategies.
Micro-Segmentation: AI will create and analyze super-specific customer segments for more accurate forecasting.
Dynamic Pricing Optimization: Real-time price adjustments based on individual customer behavior and market conditions.
Predictive Lead Scoring: Advanced algorithms will find high-value leads with unprecedented accuracy.
2. Natural language processing (NLP)
AI will analyze customer communications, social media, and market sentiment in real-time and incorporate this into forecasts.
Sentiment Analysis: Real-time tracking of customer and market sentiment to adjust forecasts.
Voice Activated Forecasting: Natural language interfaces to query and interact with AI forecasting systems.
Automated Meeting Analysis: AI transcription and analysis of sales calls to extract insights and update forecasts.
3. Autonomous sales optimization
AI will not only forecast outcomes but also autonomously adjust sales strategy, optimize pricing, and allocate resources in real time.
AI Sales Playbooks: Automatically generated and updated sales strategies based on forecasted scenarios.
Intelligent Resource Allocation: Dynamic assignment of sales resources based on AI-predicted opportunity value.
Automated Nurture Campaigns: AI-optimized, personalized customer journeys that adapt based on forecasted behavior.
5. Predictive market intelligence
AI will provide forward-looking insights into market trends, competitor activity, and economic factors that impact sales.
Trend Detection: Identifying trends before they become apparent to human analysts.
Competitive Intelligence Forecasting: Forecasting competitor moves and their impact on your sales pipeline.
Economic Impact Modeling: Real-time adjustments to forecasts based on macroeconomic indicators and predictions.
6. Quantum computing
As quantum computing becomes more mainstream it will change the speed and complexity of AI-driven sales forecasting.
Complex Scenario Modeling: Ability to process hundreds of variables and scenarios at once.
Real Time Global Forecasting: Instant updates to global sales forecasts for hundreds of variables.
Cryptographic Security: Enhanced data protection for sensitive sales and customer data.
Conclusion: AI in sales forecasting
The integration of AI into sales forecasting is a big moment for B2B companies. By using AI, businesses can get unprecedented accuracy, operational efficiency, and strategic agility. As we’ve seen in this guide, AI-driven sales forecasting means:
Better sales outcomes
Automation and real-time visibility
Scalability to changing business and market needs
Deeper insights for better decision-making
Sales pipeline management and resource allocation
Data-driven strategy
But to successfully implement AI in sales forecasting you need to plan, change, and optimize. Follow the strategy in this guide and tackle the challenges head-on.
When you review your current forecasting methods and how they align with your strategic goals, think about the potential of AI to take your sales to the next level. Review your processes, assess your readiness for AI, and get started with a more data-driven, intelligent approach to sales forecasting.
AI is no longer just about analysis — it's about anticipation. It’s transforming not only sales, but also how companies engage with their customers through marketing. If you're interested in how these technologies will evolve beyond forecasting and shape marketing strategies as well, check out this article from M1 Project on the best AI marketing solutions for 2025. It explores how AI is shifting from being a tool to becoming a strategic partner — one that predicts customer behavior, adjusts content in real time, and helps brands personalize at scale.
Remember the future of sales is for those who can predict it and shape it. By using AI-driven sales forecasting today you’ll put your business at the forefront of the B2B sales revolution and be able to:
Make better data-driven decisions
Respond to market and customer changes
Optimize your sales strategies and resource allocation
Grow sustainable revenue in a competitive world
Implementing AI in sales forecasting may seem overwhelming but the rewards are huge. Start small, learn fast, and scale as you see the impact on your sales.
Ready to get started with AI in sales forecasting? Book a demo with Forecastio for a consultation on how to integrate the latest AI forecasting tools into your sales strategy. Let’s build a better, more profitable tomorrow.
In the B2B world where everything moves fast and changes fast, being able to predict outcomes and navigate the sales landscape is not a nice to have, it’s a must-have. At the heart of this is sales forecasting, a process that gives you invaluable insights to make smarter data-driven decisions. But despite how important it is, many companies are still using inaccurate and inefficient forecasting methods that don’t meet their strategic needs.
This guide explores the impact of Artificial Intelligence (AI) on sales forecasting so sales leaders can transform their sales operations and stay ahead of the curve in a complex market.
Sales forecasting 101: A business imperative
Before we get into the AI bit, let’s first understand what sales forecasting is and why it’s so important to business.
What is sales forecasting?
Sales forecasting is the process of predicting future sales performance based on past sales data, historical data, market trends and other relevant factors. It’s a business function that helps companies:
Forecast revenue and budget
Allocate resources
Set targets
Decide on inventory, staffing and expansion
Why accurate sales forecasts matter
Accurate sales forecasts are more than just numbers—they’re the building blocks for many strategic decisions:
Financial Planning: Forecasts inform budgeting, investment decisions and financial projections.
Resource Management: They guide inventory levels, production schedules and workforce planning.
Risk Management: Accurate forecasts help identify market downturns or opportunities so you can adjust your strategy proactively.
Performance Management: They provide a benchmark to measure actual sales performance.
Investor Relations: Reliable forecasts build trust with stakeholders and investors.
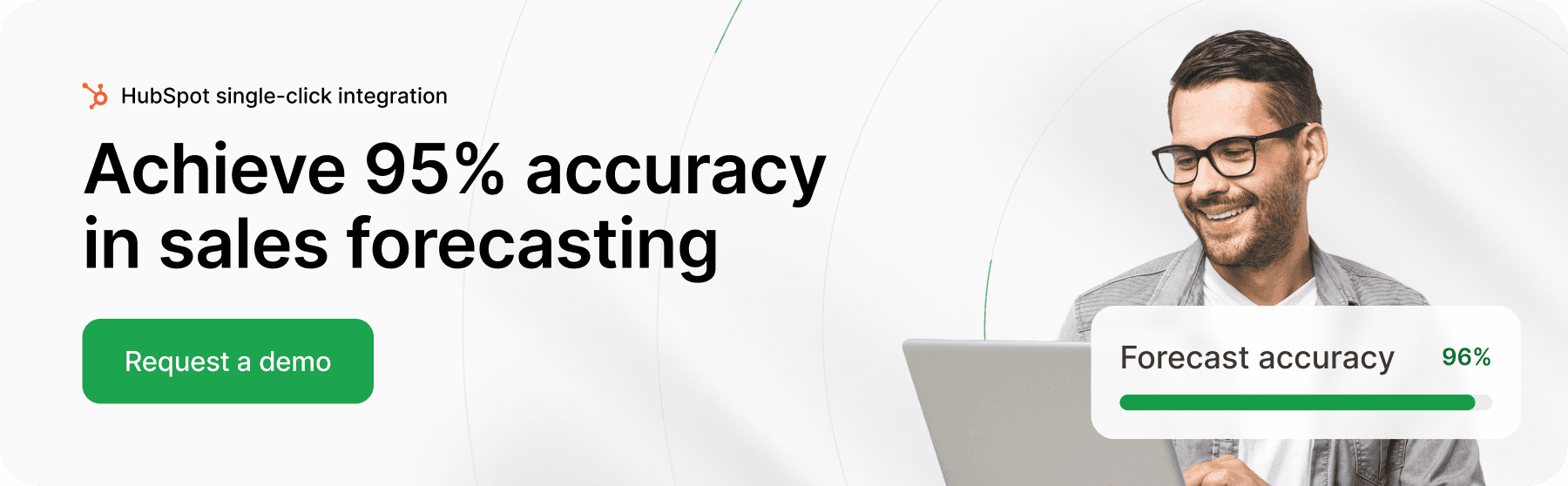
Given how important it is, you’d think sales forecasting would be a well-oiled machine in most companies. But reality is far from it.
The consequences of inaccurate forecasts on sales performance
Inaccurate sales forecasts can really hold back sales performance and create a domino effect of negative outcomes. When sales forecasts are wrong, companies end up with overstocking or understocking issues which means lost sales opportunities or wasted resources. Misallocated resources like misdirected sales teams and misaligned marketing budgets only make it worse.
Bad decisions from bad forecasts mean missed opportunities and lost revenue. Customer satisfaction suffers as sales teams struggle to meet demand accurately. This, in turn, demoralizes sales teams and leads to frustration and disappointment.
On the other hand, accurate sales forecasts enable sales teams to make informed decisions, allocate resources and meet customer demand, which means better sales performance. By using precise sales forecasts companies can optimize their operations, improve customer satisfaction, and drive revenue growth.
Sales forecasting evolution: From guesswork to data science
To understand the impact of AI on sales forecasting, let’s look at the journey from traditional methods to modern techniques.
Traditional sales forecasting methods: A historical perspective
Intuition-Based Forecasting
Relying on sales managers’ gut feel and experience
Subjective and biased
Not scalable and not consistent across large companies
Simple Trend Projections
Using basic mathematical models to extrapolate future sales from historical data
Assuming linear trends and stable market conditions
Not accounting for complex market dynamics and sudden changes
Evaluating opportunities at different stages of the sales funnel
Applying probability percentages to estimate likely closures
More accurate but still relying on subjective assessments
Time Series Analysis
Using more advanced statistical methods to identify patterns in historical data
Including seasonality and cyclical trends
Limited ability to factor in external variables and market changes
Drawbacks of traditional forecasting methods
While these traditional methods were the foundation of sales forecasting, they have significant limitations in today’s fast paced, data driven business world:
No Real-Time Adaptability
Traditional forecasts don’t account for sudden market changes or emerging trends
Updates are periodic which means outdated projections
Human Bias and Subjectivity
Forecasts can be influenced by individual perspectives which means overly optimistic or pessimistic projections
Inconsistency across different forecasters or departments
Limited Data Integration
Traditional methods can’t integrate multiple data sources
Siloed data means incomplete or fragmented forecasts
Time Consuming Processes
Manual data entry and analysis slows down the forecasting process
Delays in getting forecasts mean missed opportunities or delayed decisions
Scalability Issues
As companies grow, traditional methods become more cumbersome and error-prone
Can’t maintain consistency across larger and more complex sales organizations
Can’t Handle Multiple Variables
Traditional methods struggle with multi-variable analysis
Can’t account for external factors like economic conditions, competitive actions, or regulatory changes
Limited Forecasting Ability
Focusing on historical data may not capture emerging trends or disruptors
Can’t provide insights beyond basic sales projection
The consequences of this are severe: misallocated resources, missed revenue opportunities, and reduced operational efficiency. As companies are forced to make decisions in real time using data, the limitations of traditional forecasting methods are becoming more and more apparent. Sales forecasting software is the answer by allowing real-time adjustments, better data analysis, and better decision-making and operational efficiency.
AI: A game changer for sales forecasting
The combination of Artificial Intelligence with sales forecasting is a giant leap forward, addressing the limitations of traditional methods and introducing new possibilities. AI driven sales forecasting uses advanced algorithms, machine learning, and big data analytics to transform the accuracy, speed, and depth of sales predictions.
AI driven sales forecasting components
Machine Learning Algorithms
Uses complex statistical models to find patterns and relationships in data
Learns and improves predictions with new information
Natural Language Processing (NLP)
Analyzes unstructured data from emails, call logs, and social media
Extracts insights from customer interactions and market sentiment
Big Data Analytics
Processes multiple data sources in real-time
Finds correlations and trends in customer data that would be impossible to find manually
Predictive Analytics
Goes beyond historical analysis to predict future outcomes
Provides probability-based predictions for individual deals and overall sales performance
Prescriptive Analytics
Gives recommendations to improve sales outcomes
Suggests strategies based on forecasted scenarios
How AI changes the forecasting process
Real-Time Data Processing
AI systems process vast amounts of data in real-time and provide instant forecasts
Responds to changing market conditions quickly
Advanced Pattern Recognition
Machine learning algorithms find complex patterns and correlations that human analysts can’t
Uncovers hidden insights that drive better predictions
Multi-Variable Analysis
AI can process many variables: macro trends to individual customer behaviour
Gives a 360 degree view of what’s driving sales performance
Automated Data Collection and Integration
AI tools collect and integrate data from multiple sources
No manual data entry errors and complete analysis
Personalised Forecasting
Forecasts at individual, team, product, and company level
Forecasts tailored to specific sales roles, territories, or customer segments
Scenario Modelling
Allows sales leaders to simulate different scenarios and their outcomes
Better planning and risk management
Data processing, enrichment, and analysis for future sales
Data processing, enrichment, and analysis are key to accurate sales forecasting. Using advanced technologies like AI and ML, sales teams can process large amounts of data quickly and efficiently. Enriching this data with external sources like market trends and customer behavior gives a complete view of the sales landscape.
Analyzing this enriched data allows sales teams to find patterns and trends that will inform future sales. This data-driven approach allows sales teams to forecast sales more accurately and manage the sales pipeline better. By using AI and ML businesses can turn raw data into actionable insights and get more precise sales forecasts and better decision making.
AI in sales forecasting: The benefits
Integrating AI in sales forecasting brings many benefits that can impact the bottom line and competitive position.
1. Unmatched accuracy
AI eliminates human error and bias, using large datasets and complex algorithms to produce accurate forecasts. This accuracy gives more confidence in decision-making and resource allocation.
Forecast Error Reduction: AI forecasts can reduce error rates by up to 50% compared to traditional methods.
Consistency: AI is accurate across different time horizons, from short-term operational forecasts to long-term strategic projections.
Early Warning Systems: AI can detect subtle signs of forecast deviations and allow for proactive adjustments.
2. Productivity
By automating data collection, analysis, and reporting, AI reduces the time and effort required for forecasting. Sales teams can focus on high-value activities like relationship building and closing deals.
Time Savings: AI can reduce forecasting time by up to 80%, freeing up resources.
Automated Reporting: Get forecast reports with a click, no manual compilation is required.
Real-Time Updates: AI systems can update forecasts in real-time without human intervention.
3. Scalability
AI systems scale with your business and adapt to market changes. This flexibility means your forecasting stays robust and relevant regardless of your company size or market conditions.
Complexity: AI can handle thousands of SKUs or customer segments.
Market Responsiveness: Quickly add new data points or market shifts to forecasts.
Cross-Functional: Easily extend forecasting to different departments and business units.
4. Deeper insights and decision-making
AI finds hidden patterns and correlations in your sales data, providing valuable insights into customer behavior, market trends, and sales performance. This gives sales leaders the ability to make data-driven decisions that drive revenue growth.
Customer Behavior Analysis: Understand what drives buying decisions and predict future customer behavior.
Market Trend Detection: Detect trends before they become obvious, and get first mover advantage.
Performance Driver Analysis: Know what drives sales performance and focus on that.
5. Sales pipeline management
AI powered forecasting tools give you a granular view of your sales pipeline, to improve sales pipeline management by identifying bottlenecks, optimizing deal velocity, and prioritizing high-potential opportunities.
Opportunity Scoring: Auto score deals based on the likelihood of closing.
Stage Progression Analysis: What accelerates or hinders deal progression through the pipeline.
Resource Allocation: Direct sales efforts and resources to the best opportunities.
6. Collaboration and alignment
AI powered forecasting platforms can bring better communication and alignment across sales teams, management, and other departments.
Shared Visibility: One source of truth for sales projections across the company.
Cross-Functional Planning: Better coordination between sales, marketing, finance, and operations.
Goal Setting and Tracking: Set more realistic targets and track them in real-time.
7. Competitive advantage
Use AI in sales forecasting and you’ll be ahead of the competition.
Faster Market Response: Adjust strategies based on real-time forecast updates.
Better Customer Experience: Use predictive insights to anticipate and serve customer needs.
Data Driven Culture: Create an organization that values and acts on data driven insights.
How to implement AI-driven sales forecasting: A step by step approach
Implementing AI into your sales forecasting process requires planning and execution. Here’s a step-by-step guide:
1. Review your current forecasting
Pain Points: Document current issues with accuracy, timeliness, or resource allocation.
Data Quality: Assess the completeness, accuracy, and accessibility of your sales data.
Current Workflows: Map out how forecasting fits into other business processes.
KPIs: Define how you will measure forecast accuracy and impact.
2. Set clear objectives
Specific, measurable goals for your AI driven forecasting project:
Improve Forecast Accuracy: Target percentage improvement in forecast accuracy.
Reduce Forecasting Time: Cut down time spent on forecasting activities.
Better Decision Making: How will improved forecasts inform business decisions.
Increase Win Rates: Target improvement in deal close rates through better opportunity prioritization.
3. Select the right AI forecasting tool
Choose an AI forecasting tool that fits your business and technical landscape:
Integration: Ensure seamless integration with your existing CRM and sales tools.
Scalability: Think about future growth and how the solution will scale with your business.
Customization: Look for platforms that can be configured to your sales process.
User Experience: Prioritize solutions with simple interfaces for broad adoption.
Vendor Expertise: What’s the vendor’s experience in your industry and support options.
4. Data infrastructure
Get your data ready for AI forecasting:
Data Centralization: Consolidate sales data from multiple sources into one place.
Data Cleansing: Put processes in place to clean and standardize historical and incoming data.
Data Governance: Define data management and quality control policies.
Real-Time Data Pipelines: Set up secure data flows for continuous forecast updates.
5. Pilot
Pilot Group: Choose a specific sales team or product line to start with.
Timelines: Define a timeline for the pilot including key milestones and review points.
Metrics: Monitor forecast accuracy, user adoption, and sales performance.
Feedback: Gather feedback from users and stakeholders to identify areas to improve.
Iterative Refining: Use pilot insights to refine the AI models and processes before full rollout.
6. Training and change management
Get your sales team ready for AI forecasting:
Training Programs: Develop role-specific training on the new tools and processes.
Executive Sponsorship: Ensure leadership visibility to drive adoption.
Change Champions: Identify and empower influential team members to promote the new way.
Communication: Address concerns and highlight benefits through ongoing communication.
Incentive Alignment: Adjust metrics and incentives to encourage AI adoption.
7. Monitor and refine
Performance Reviews: Regularly review forecast accuracy and business impact.
Model Retraining: Continuously update AI models with new data.
Stay Up To Date: Keep current with AI forecasting technology and best practices.
User Feedback Loop: Establish a feedback mechanism to drive continuous improvement.
Cross-Functional Collaboration: Regular dialogue between sales, IT, and data science teams to optimize the system.
AI Forecasting implementation challenges
Bringing AI into the sales process can make it more efficient and accurate by automating the mundane, so sales teams can focus on data analysis and market trends.
1. Data quality and format issues
Problem: Poor data quality or incompatible data formats can kill AI forecast accuracy.
Solutions:
Do a data audit to find the issues.
Implement data validation rules in your CRM and other data sources.
Invest in data integration tools to get the data flowing.
Monitor data quality ongoing.
2. Change resistance
Problem: Sales teams may not want to adopt new AI forecasting methods and stick to what they know.
Solutions:
Get key stakeholders involved early in the implementation to get buy-in.
Show clear ROI and benefits through pilot programs and case studies.
Provide ongoing support and celebrate early wins to build momentum.
Address concerns openly and highlight how AI complements not replaces human expertise.
Offer role-specific training to show how AI can make each sales rep more successful.
3. Existing systems
Problem: Integrating AI forecasting tools with existing CRM and sales systems can be tricky.
Solutions:
Choose AI solutions with strong APIs and pre-built integrations.
Work with your IT department to get the integration and data flowing.
Consider a phased rollout to minimize disruption.
Test integrations thoroughly before going live.
Develop a data architecture plan to show how AI forecasting will sit in your tech stack.
4. AI vs human
Problem: Balancing AI forecasts with human judgment can be hard.
Solutions:
Train sales leaders to interpret and act on AI insights.
Encourage data-driven decision-making while valuing human expertise and market knowledge.
Create a feedback loop for sales teams to input into the AI models.
Use AI forecasts as a conversation starter, not a final answer.
Compare AI forecasts with human forecasts regularly to see where both can improve.
5. Cost and ROI
Problem: Justifying the investment in AI forecasting technology can be tough, especially for smaller businesses.
Solutions:
Start with a pilot to show tangible ROI before going full scale.
Calculate the cost savings from better forecast accuracy and efficiency gains.
Consider cloud-based AI forecasting solutions with more flexible pricing models.
Quantify the strategic value of better decision-making enabled by AI insights.
Build a business case that shows both the short and long-term benefits of AI forecasting.
AI in B2B sales forecasting in the future
As AI gets better we can expect even more advanced capabilities in sales forecasting. Here’s a sneak peek into the future of AI driven B2B sales forecasting:
1. Hyper personalized forecasting
AI will enable forecasting at the individual customer level, predicting specific product needs and optimal engagement strategies.
Micro-Segmentation: AI will create and analyze super-specific customer segments for more accurate forecasting.
Dynamic Pricing Optimization: Real-time price adjustments based on individual customer behavior and market conditions.
Predictive Lead Scoring: Advanced algorithms will find high-value leads with unprecedented accuracy.
2. Natural language processing (NLP)
AI will analyze customer communications, social media, and market sentiment in real-time and incorporate this into forecasts.
Sentiment Analysis: Real-time tracking of customer and market sentiment to adjust forecasts.
Voice Activated Forecasting: Natural language interfaces to query and interact with AI forecasting systems.
Automated Meeting Analysis: AI transcription and analysis of sales calls to extract insights and update forecasts.
3. Autonomous sales optimization
AI will not only forecast outcomes but also autonomously adjust sales strategy, optimize pricing, and allocate resources in real time.
AI Sales Playbooks: Automatically generated and updated sales strategies based on forecasted scenarios.
Intelligent Resource Allocation: Dynamic assignment of sales resources based on AI-predicted opportunity value.
Automated Nurture Campaigns: AI-optimized, personalized customer journeys that adapt based on forecasted behavior.
5. Predictive market intelligence
AI will provide forward-looking insights into market trends, competitor activity, and economic factors that impact sales.
Trend Detection: Identifying trends before they become apparent to human analysts.
Competitive Intelligence Forecasting: Forecasting competitor moves and their impact on your sales pipeline.
Economic Impact Modeling: Real-time adjustments to forecasts based on macroeconomic indicators and predictions.
6. Quantum computing
As quantum computing becomes more mainstream it will change the speed and complexity of AI-driven sales forecasting.
Complex Scenario Modeling: Ability to process hundreds of variables and scenarios at once.
Real Time Global Forecasting: Instant updates to global sales forecasts for hundreds of variables.
Cryptographic Security: Enhanced data protection for sensitive sales and customer data.
Conclusion: AI in sales forecasting
The integration of AI into sales forecasting is a big moment for B2B companies. By using AI, businesses can get unprecedented accuracy, operational efficiency, and strategic agility. As we’ve seen in this guide, AI-driven sales forecasting means:
Better sales outcomes
Automation and real-time visibility
Scalability to changing business and market needs
Deeper insights for better decision-making
Sales pipeline management and resource allocation
Data-driven strategy
But to successfully implement AI in sales forecasting you need to plan, change, and optimize. Follow the strategy in this guide and tackle the challenges head-on.
When you review your current forecasting methods and how they align with your strategic goals, think about the potential of AI to take your sales to the next level. Review your processes, assess your readiness for AI, and get started with a more data-driven, intelligent approach to sales forecasting.
AI is no longer just about analysis — it's about anticipation. It’s transforming not only sales, but also how companies engage with their customers through marketing. If you're interested in how these technologies will evolve beyond forecasting and shape marketing strategies as well, check out this article from M1 Project on the best AI marketing solutions for 2025. It explores how AI is shifting from being a tool to becoming a strategic partner — one that predicts customer behavior, adjusts content in real time, and helps brands personalize at scale.
Remember the future of sales is for those who can predict it and shape it. By using AI-driven sales forecasting today you’ll put your business at the forefront of the B2B sales revolution and be able to:
Make better data-driven decisions
Respond to market and customer changes
Optimize your sales strategies and resource allocation
Grow sustainable revenue in a competitive world
Implementing AI in sales forecasting may seem overwhelming but the rewards are huge. Start small, learn fast, and scale as you see the impact on your sales.
Ready to get started with AI in sales forecasting? Book a demo with Forecastio for a consultation on how to integrate the latest AI forecasting tools into your sales strategy. Let’s build a better, more profitable tomorrow.
Share:
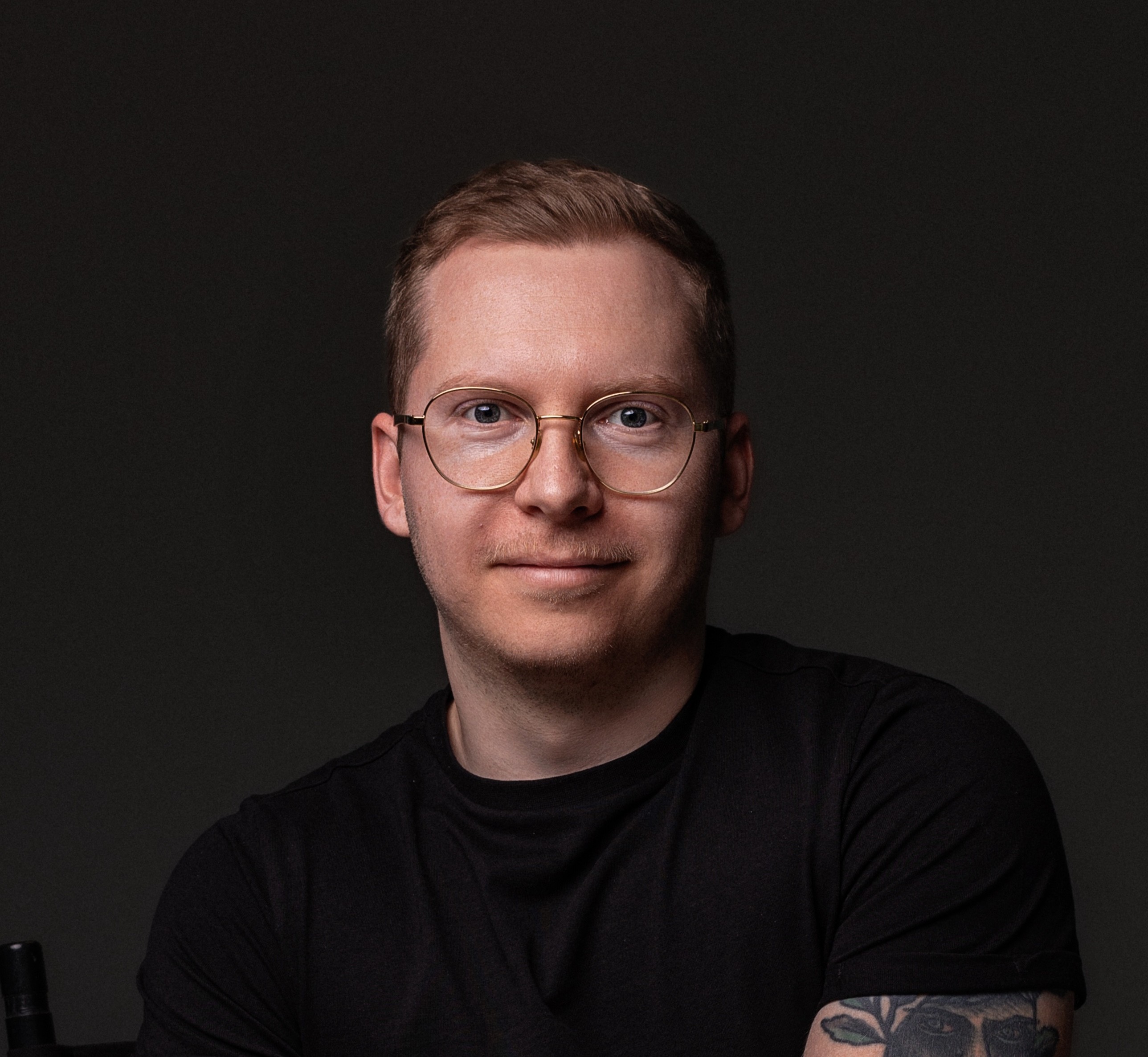
Dmytro is a seasoned marketing professional with over 10 years in the B2B and startup ecosystem. He is passionate about helping companies better plan their revenue goals, improve forecast accuracy, and proactively address performance bottlenecks or seize growth opportunities.
Dmytro is a seasoned marketing professional with over 10 years in the B2B and startup ecosystem. He is passionate about helping companies better plan their revenue goals, improve forecast accuracy, and proactively address performance bottlenecks or seize growth opportunities.
Related articles
Pipeline Management
Feb 17, 2025
9 min
Pipeline Management
Feb 17, 2025
9 min
Sales Performance
Feb 17, 2025
9 min
Sales Performance
Feb 17, 2025
9 min
Sales Forecasting
Feb 17, 2025
10 min
Sales Forecasting
Feb 17, 2025
10 min
Pipeline Management
Feb 17, 2025
9 min
Sales Performance
Feb 17, 2025
9 min
Pipeline Management
Feb 17, 2025
9 min
Sales Performance
Feb 17, 2025
9 min
Sales Planning
Sales Forecasting
Sales Performance Insights
Sales Planning
Sales Forecasting
Sales Performance Insights
Sales Planning
Sales Forecasting
Sales Performance Insights
© 2025 Forecastio, All rights reserved.
Sales Planning
Sales Forecasting
Sales Performance Insights
Sales Planning
Sales Forecasting
Sales Performance Insights
Sales Planning
Sales Forecasting
Sales Performance Insights
© 2025 Forecastio, All rights reserved.
Sales Planning
Sales Forecasting
Sales Performance Insights
Sales Planning
Sales Forecasting
Sales Performance Insights
Sales Planning
Sales Forecasting
Sales Performance Insights
© 2025 Forecastio, All rights reserved.
Sales Planning
Sales Forecasting
Sales Performance Insights
Sales Planning
Sales Forecasting
Sales Performance Insights
Sales Planning
Sales Forecasting
Sales Performance Insights
© 2025 Forecastio, All rights reserved.