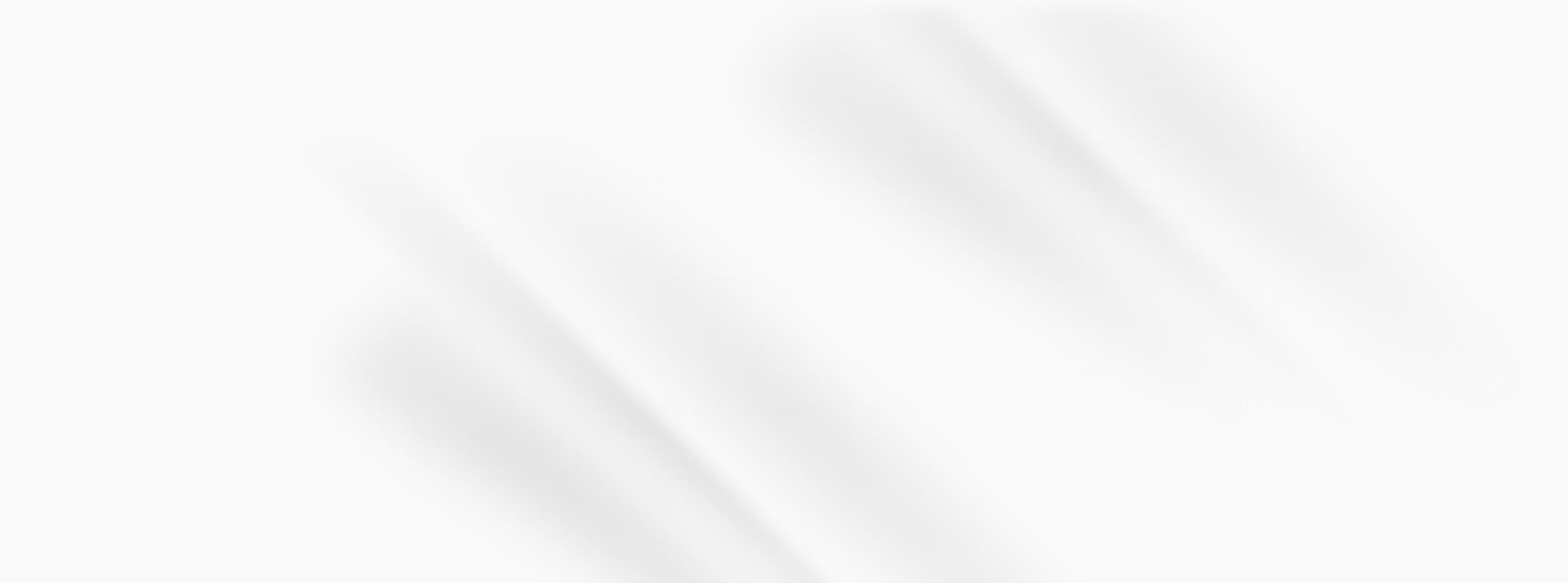
SaaS Sales Forecasting: Methods, Tips & Mistakes to Avoid
May 23, 2025
May 23, 2025
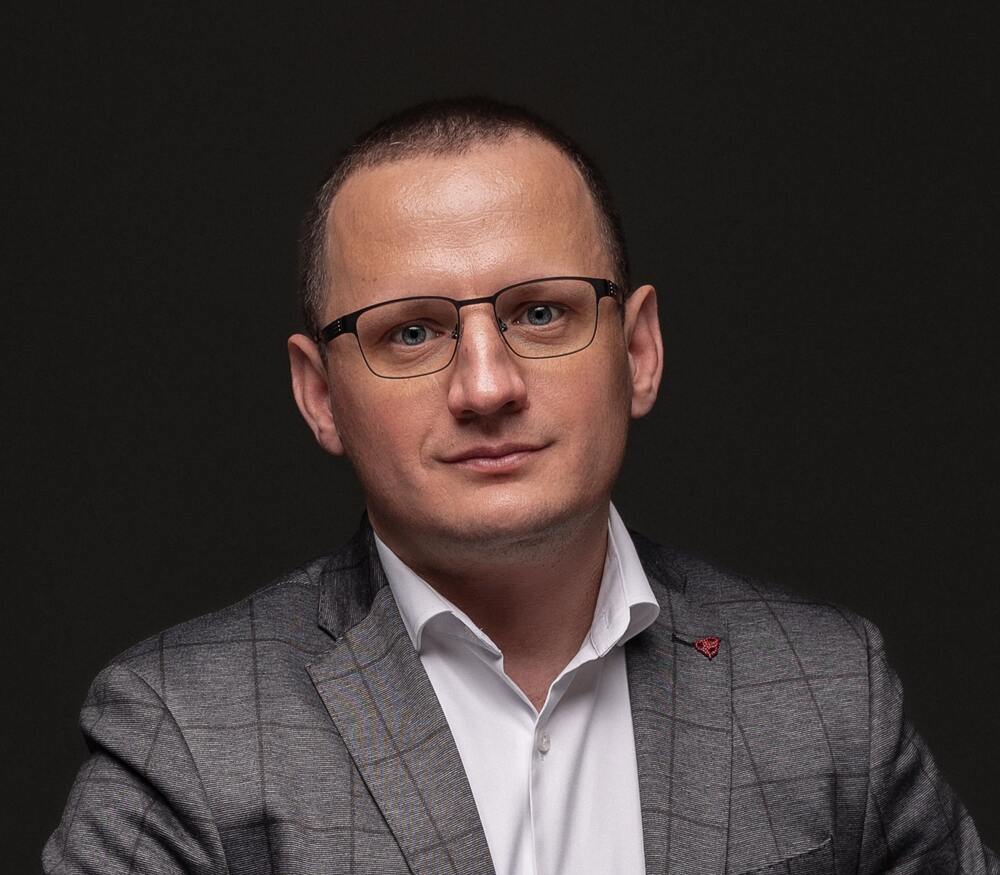
Alex Zlotko
CEO at Forecastio
Last updated
May 23, 2025
Reading time
10 min
Share:
Share
Table of Contents




TL;DR
TL;DR
SaaS sales forecasting requires tracking different metrics than traditional sales models entirely.
Focus on monthly recurring revenue, churn rate, and pipeline coverage ratios.
Separate your forecasts into three distinct buckets: new business, renewals, and expansion revenue.
Each behaves differently and needs its own forecasting approach.
Update forecasts weekly, not monthly, because SaaS markets move fast.
Clean CRM data drives accuracy –– require sales reps to update deal notes and realistic close dates regularly.
Don't trust rep gut feelings alone.
Always involve sales managers for frontline insights.
Track your forecast accuracy against actual results monthly to improve performance over time consistently now.
SaaS sales forecasting requires tracking different metrics than traditional sales models entirely.
Focus on monthly recurring revenue, churn rate, and pipeline coverage ratios.
Separate your forecasts into three distinct buckets: new business, renewals, and expansion revenue.
Each behaves differently and needs its own forecasting approach.
Update forecasts weekly, not monthly, because SaaS markets move fast.
Clean CRM data drives accuracy –– require sales reps to update deal notes and realistic close dates regularly.
Don't trust rep gut feelings alone.
Always involve sales managers for frontline insights.
Track your forecast accuracy against actual results monthly to improve performance over time consistently now.
Introduction
For SaaS companies, accurate sales forecasting is far more than a “nice-to-have”, it’s the foundation for sustainable growth. Whether you're reporting on monthly recurring revenue (MRR), planning team expansion, or navigating financial planning, SaaS sales forecasting plays a critical role in keeping your SaaS business model stable, predictable, and scalable.
Unlike traditional industries, SaaS revenue forecasting is layered with complexity. Recurring revenue, long sales cycles, varied customer lifetime values, and the risk of churn demand a more tailored approach to forecasting sales. These unique dynamics make accurate sales predictions both more challenging and more vital for growth-oriented SaaS businesses.
In this article, we’ll break down what makes SaaS sales forecasting different, how to select the right forecasting models, which key metrics you need to monitor, and proven strategies to improve forecast accuracy over time. We'll also explore how to use historical data, analyze sales pipeline data, and align your marketing and sales efforts to support better decision-making.
If you’re looking to level up your SaaS revenue forecasting strategy, be sure to download our guide on Sales Forecasting Methods for a deeper dive into best practices and real-world examples.
Why SaaS Sales Forecasting Is Different
Unlike transactional business models, SaaS companies operate on a foundation of recurring revenue. That changes the game for forecasting. In a SaaS business, predicting future sales isn’t just about closing new deals, it’s about understanding the full revenue journey, including customer retention, upselling, and customer behavior over time.
Here’s what sets SaaS sales forecasting apart:
1. Recurring Revenue Focus
In SaaS revenue forecasting, you must consider both new customer acquisitions and existing account renewals. Metrics like monthly recurring revenue (MRR) and annual recurring revenue (ARR) are essential. Forecasts that ignore renewal cycles or customer lifetime value (CLV) often fall short.
2. Churn & Expansion Dynamics
Forecasting sales without factoring in churn and expansion revenue can distort your view of reality. One high-value customer lost can offset multiple new deals. That’s why accurate forecasts must account for both revenue contraction and expansion.
3. Longer Customer Lifecycles
In many SaaS businesses, deals don't deliver full value upfront. Instead, revenue builds gradually across months or even years, especially in enterprise models with long sales cycles. Your forecasting process must account for deferred revenue realization.
4. Onboarding and Ramp Time
Revenue often doesn’t activate immediately. Implementation delays, user onboarding, and internal ramp-up timelines can push real value delivery into future periods. That’s why forecasting models should align with actual sales pipeline and delivery dynamics.
A Holistic Forecasting Approach
To build accurate revenue predictions, SaaS sales teams must consider more than just pipeline deals. Factors like product usage, customer satisfaction, customer success engagement, and historical sales data all play key roles in refining your forecasts.
📈 Want to dive deeper into building a robust strategy? Read our article on how to improve sales forecasting accuracy to learn actionable techniques used by top-performing SaaS teams.
Key Metrics to Track in SaaS Forecasting
Tracking the right key performance indicators (KPIs) is essential for building accurate SaaS sales forecasts. These metrics don’t just show what your SaaS company might close, they help predict what you could retain, lose, or expand. For any data-driven sales forecasting process, these are your must-haves:
Monthly Recurring Revenue (MRR) & Annual Recurring Revenue (ARR)
Your core SaaS revenue forecasting starts here.
MRR = Total monthly subscription revenue
ARR = MRR × 12
These metrics reflect your recurring revenue stream and are vital for financial planning and reporting.
Churn Rate
Churn Rate = Lost MRR ÷ Total MRR at the start of the period
Churn directly impacts future revenue and forecast accuracy. A high churn rate can quickly erode gains from new customer acquisition.
Net Revenue Retention (NRR)
NRR = (MRR from existing customers + expansion – churn) ÷ MRR at the start
A strong NRR signals customer satisfaction and expansion revenue, both critical for sustainable growth in a SaaS business model.
Pipeline Coverage
Pipeline Coverage = Open pipeline ÷ Target quota (per period)
This metric gives insight into whether your sales team has enough sales pipeline data to meet targets. It’s a leading indicator for revenue forecasting.
Sales Cycle Length
The average time from opportunity creation to closed deal.
Understanding your average sales cycle helps forecast sales accurately and allocate resources efficiently.
Lead Velocity Rate (LVR)
LVR = (Qualified leads this month – last month) ÷ last month × 100
This measures the speed at which your pipeline is growing. It’s a forward-looking metric that ties directly to future sales and marketing efforts.
By monitoring these key metrics, SaaS sales leaders can better anticipate market shifts, adjust their sales strategies, and produce accurate revenue predictions.
Need help tracking these KPIs in real time? Book a Forecastio demo to get instant visibility into your pipeline, performance, and forecasts.
Forecasting Methods for SaaS Companies
Choosing the right forecasting models is a key step in building a reliable SaaS sales forecasting strategy. Because of the recurring nature of SaaS revenue, different approaches may be needed depending on your company’s size, sales process, and data quality. Below are some of the most effective sales forecasting methods tailored for SaaS companies:
1. Historical Forecasting
This method relies on historical sales data and trend analysis to predict future sales. It’s ideal for SaaS businesses experiencing steady, predictable growth without major market disruptions.
👉 Best for: Mature products, consistent growth, and minimal churn.
2. Pipeline Stage Forecasting
This model applies stage-based probabilities to open deals in your sales pipeline. While popular, it depends heavily on clean CRM data and accurate stage mapping.
👉 Best for: Sales-led SaaS companies with defined sales cycles and strong CRM discipline.
3. Weighted Pipeline Forecasting
An advanced version of stage-based forecasting that adjusts each deal’s value based on both probability and deal stage. It offers more nuanced predictions of SaaS revenue.
👉 Best for: Teams seeking to align forecasting efforts more closely with reality.
➡ Explore more in our dedicated post on the weighted pipeline.
4. Cohort-Based Forecasting
This approach analyzes revenue trends by customer cohorts (e.g., by signup month, segment, or vertical). It helps forecast churn, upsells, and customer lifetime value with greater granularity.
👉 Best for: SaaS platforms with diverse customer segments or product use cases.
5. Segmented Forecasting
Forecasts different streams like new business, expansion, and renewals separately. This allows for better revenue projection data and strategy per stream.
👉 Best for: SaaS companies with both land-and-expand and enterprise renewal motions.
6. AI/ML Forecasting
AI-powered tools like Forecastio analyze historical data, rep activity, and deal health to deliver more accurate sales forecasting than manual methods.
👉 Best for: Growth-stage and enterprise SaaS companies looking to reduce bias and improve forecast accuracy.
➡ Dive into how AI transforms forecasting in our AI in Sales Forecasting article.
Each method has its strengths, depending on your SaaS business model, sales team size, and the richness of your data. Many modern SaaS sales leaders combine these models to create a robust forecasting model that adapts to market shifts and customer behavior.

Best Practices for SaaS Sales Forecasting
Building accurate sales forecasting processes in a SaaS business isn’t just about choosing the right model, it’s about discipline, structure, and operational consistency. To improve forecast accuracy and ensure your SaaS revenue forecasting stays actionable, implement the following best practices:
1. Forecast by Revenue Type
Separate your forecasts for new business, expansion revenue, and renewals. These revenue streams behave differently and should be forecasted using distinct forecasting models. This is key for accurate monthly recurring revenue and annual recurring revenue tracking.
2. Segment by Sales Motion
Different sales cycles and close rates call for tailored forecasting. Segment your forecast by sales motion. For example, SMB vs. enterprise or inbound vs. outbound. This helps align your sales strategies with actual performance data and improves your ability to predict future sales.
3. Update Forecasts Frequently
In fast-moving SaaS companies, static monthly forecasts fall behind reality. High-growth teams update their sales forecasts weekly or bi-weekly to reflect the latest shifts in the sales pipeline and customer behavior.
4. Enforce Deal Notes & Close Dates
Healthy forecasts rely on accurate pipeline data. Require sales reps to log detailed deal notes and maintain realistic close dates. These small habits improve forecasting efforts dramatically and help sales leaders catch risk signals early.
5. Maintain Clean CRM Data
Your forecasting process is only as strong as your CRM hygiene. Use rules and automation to ensure sales pipeline data stays clean, up to date, and reflective of real opportunities. This supports data-driven financial planning and minimizes surprises.
6. Involve Sales Managers
Sales managers offer frontline insights into deal quality and rep confidence. Their input helps validate or challenge forecast assumptions, improving forecast accuracy across the board. Include them in your review cadence.
Book a demo to see how Forecastio can automate your sales forecasting process, enforce clean data practices, and support your sales leaders in making smarter decisions.
Common Mistakes in SaaS Forecasting
Even with the right forecasting models and tools in place, many SaaS companies still struggle to produce accurate sales forecasts. Why? Because success in SaaS sales forecasting relies as much on discipline as it does on data. Here are some of the most common mistakes that derail otherwise solid forecasting efforts:
1. Blind Trust in Rep Projections
Forecasting based solely on what sales reps “feel” will close—without reviewing deal notes, pipeline stages, or customer behavior—leads to overconfidence and missed targets. Always validate forecasts with sales data and frontline sales manager input.
2. Ignoring Churn and Downgrades
Many forecasts emphasize new sales while ignoring churn, downgrades, or contraction MRR. But in the SaaS business model, lost recurring revenue can easily offset gains. Your SaaS revenue forecasting should always account for both growth and loss.
3. Using Stale Pipeline Data
Outdated close dates, inactive deals, and untouched records distort your forecasts. Clean, timely sales pipeline data is the backbone of forecast accuracy. Automate CRM hygiene where possible.
4. Treating New and Expansion Revenue the Same
New business and expansion revenue behave differently, they have distinct sales cycles, conversion rates, and average deal sizes. Lumping them together in forecasts reduces forecast precision and undermines resource planning.
5. Not Analyzing Forecast vs. Actual
Many teams miss the opportunity to improve by failing to review forecast accuracy post-mortem. Regularly examine past sales performance and measure how forecasts aligned with reality. This helps refine assumptions and build better models over time.
Ultimately, a SaaS sales forecast is only as strong as the operational discipline behind it. Avoiding these pitfalls can significantly improve your ability to predict future sales, manage financial planning, and guide marketing and sales efforts.
Forecasting Tools for SaaS Sales Teams
While manual spreadsheets might work for early-stage SaaS businesses, they quickly become unmanageable as your sales pipeline grows and revenue streams diversify. For scalable, accurate sales forecasting, adopting dedicated forecasting tools is essential.
Here are some popular options SaaS companies should consider:
✅ Forecastio
A purpose-built sales forecasting platform designed specifically for HubSpot users. Forecastio offers forecasting by pipeline stage and revenue stream. It also leverages time series analysis, which is ideal for SaaS companies with short sales cycles and steady growth. This makes it especially effective for mid-sized SaaS teams looking to improve forecast accuracy and track recurring revenue with precision.

🏢 Clari
An enterprise-grade revenue intelligence platform used by larger SaaS companies. It integrates deeply with multiple CRMs and uses AI to track sales rep behavior, deal health, and pipeline trends. Best suited for high-volume, complex sales cycles.
📊 InsightSquared
A strong player for pipeline analytics and sales performance visibility. Offers robust reporting, visual dashboards, and deal-level intelligence to support your forecasting process.
🔧 Salesforce Forecasting
Built into Salesforce CRM, this tool provides basic sales forecasting capabilities. While useful for teams already using Salesforce, it often requires significant customization to match the needs of fast-moving SaaS sales teams.
🧮 Spreadsheets
Still widely used for their flexibility but prone to errors, data decay, and version control issues. Fine for very early-stage teams, but not recommended for mature SaaS revenue operations.
Choosing the right tool depends on your CRM integration, sales team size, data quality, and reporting needs. A modern forecasting model should support recurring revenue, track key metrics, and help allocate resources efficiently based on live sales data.
Real-World Forecasting Example
Let’s bring SaaS sales forecasting to life with a simple example.
Imagine you’re running a growing SaaS startup. Your current monthly recurring revenue (MRR) is $100K. You’re projecting some churn, expecting new deals to close, and your Customer Success team is working on upsell opportunities. Here’s how a real-world forecasting model might look:
📈 Forecast Breakdown
Starting MRR: $100,000
Churn (3%): –$3,000
New Business Expected: +$20,000
Expansion Revenue (CS Pipeline): +$10,000
✅ Forecasted MRR for Next Month: $127,000
This combined view of new, churned, and expansion revenue offers a more realistic picture of your company’s future revenue. It allows you to forecast sales accurately, align marketing and sales efforts, and track the health of your SaaS business model.
This is exactly why separating revenue streams and forecasting each based on actual sales pipeline data and historical trends is a best practice in modern SaaS revenue forecasting.
How to Improve Forecast Accuracy Over Time
Accurate sales forecasting isn’t something you get right on day one, it’s a skill that improves with structure, feedback, and team-wide discipline. For SaaS companies, the key to better forecast accuracy lies in refining both the process and the data that power your forecasts.
Here are proven ways to improve your SaaS sales forecasting over time:
🧹 Invest in CRM Hygiene
Your forecasting model is only as good as your sales pipeline data. Make CRM hygiene a shared responsibility, not just a sales ops task. Ensure sales reps update stages, close dates, and deal notes regularly to avoid forecasting blind spots.
📊 Analyze Forecast vs. Actual
Schedule time each month to compare your forecasted revenue against actual results. This helps your team learn where assumptions were off and adjust future sales forecasting efforts based on real-world performance.
🤖 Use Deal Scoring or AI
Modern SaaS sales forecasting tools offer deal scoring and AI-driven insights to identify at-risk deals and surface hidden trends in your pipeline. These systems use historical data and rep behavior to enhance forecast accuracy.
📅 Hold Weekly Forecast Reviews
Run short, consistent forecast check-ins with your sales managers. These sessions allow leaders to validate assumptions, update timelines, and challenge overconfident projections based on frontline knowledge.
📈 Track Forecast Accuracy as a KPI
Make forecasting accuracy a team-level key performance indicator. When everyone is accountable, your forecasting process becomes more data-driven and trustworthy over time.
The most successful SaaS companies don’t just forecast, they learn from every forecast. Continuous improvement is what transforms your SaaS revenue forecasting from guesswork into a growth asset.
Conclusion
SaaS sales forecasting isn’t about predicting the future with perfect precision, it’s about strengthening your ability to plan, adapt, and scale with confidence.
The best forecasts are built over time, not overnight. Start by choosing the right forecasting method for your stage, ensuring clean sales data, and aligning your forecast with your SaaS business model. With each cycle, you’ll gain more insight, reduce uncertainty, and make smarter, faster decisions for your team and customers.
✅ Keep it simple at the start.
✅ Focus on forecast accuracy, not perfection.
✅ Build a feedback loop and let your forecasting process evolve with your growth.
Done is better than perfect, especially in a fast-moving SaaS company. The sooner you begin, the faster you’ll build forecasting into a powerful asset for financial planning, resource allocation, and revenue predictability.
🚀 Ready to improve your forecasts starting today? Book a demo and let Forecastio help you build a more accurate, scalable, and insightful forecasting engine.
FAQs
How to forecast SaaS sales?
To forecast SaaS sales, start by analyzing your historical sales data, including monthly recurring revenue (MRR), churn rate, and average sales cycle. Choose a forecasting model that fits your SaaS business stage, such as pipeline stage forecasting, cohort-based forecasting, or AI-driven models. Use clean sales pipeline data from your CRM and segment revenue by type (new, expansion, renewals) for better forecast accuracy. Accurate SaaS sales forecasting helps you plan resources, track growth, and make data-driven decisions.
What is the sales cycle in SaaS?
The sales cycle in SaaS refers to the total time it takes to move a prospect from initial contact to becoming a paying customer. It typically includes stages like lead qualification, product demo, proposal, negotiation, and closing. The average sales cycle can vary depending on your customer segment. SMB sales cycles are usually shorter, while enterprise SaaS deals take longer. Understanding your sales cycle is crucial for accurate sales forecasting, resource planning, and optimizing your SaaS business model.
What are the 5 steps of the sales forecasting process?
The sales forecasting process in SaaS typically follows these five steps:
Collect historical sales data. Analyze MRR, ARR, and past sales pipeline data to identify trends.
Choose a forecasting model. Select methods like time series analysis, pipeline forecasting, or AI-based models depending on your stage and data.
Segment your forecast. Break forecasts down by revenue type (new, renewal, expansion) and sales motion (SMB vs enterprise).
Run and review forecasts. Generate projections using your chosen model and compare with real-time pipeline updates.
Refine and track accuracy. Measure forecast accuracy, learn from discrepancies, and continuously improve your forecasting efforts.
What are the 4 principles of forecasting?
The four core principles of forecasting are:
Accuracy depends on data quality. Reliable forecasts require clean, up-to-date sales data and CRM hygiene.
All forecasts are estimates. No forecast is perfect; the goal is to improve forecast accuracy over time through feedback loops.
Shorter time frames = higher accuracy. Forecasts over shorter periods (like monthly or quarterly) tend to be more precise, especially in fast-moving SaaS companies.
Use the right model for your stage. Different forecasting models suit different growth stages and sales motions; choose based on your current business needs and customer behavior.
Introduction
For SaaS companies, accurate sales forecasting is far more than a “nice-to-have”, it’s the foundation for sustainable growth. Whether you're reporting on monthly recurring revenue (MRR), planning team expansion, or navigating financial planning, SaaS sales forecasting plays a critical role in keeping your SaaS business model stable, predictable, and scalable.
Unlike traditional industries, SaaS revenue forecasting is layered with complexity. Recurring revenue, long sales cycles, varied customer lifetime values, and the risk of churn demand a more tailored approach to forecasting sales. These unique dynamics make accurate sales predictions both more challenging and more vital for growth-oriented SaaS businesses.
In this article, we’ll break down what makes SaaS sales forecasting different, how to select the right forecasting models, which key metrics you need to monitor, and proven strategies to improve forecast accuracy over time. We'll also explore how to use historical data, analyze sales pipeline data, and align your marketing and sales efforts to support better decision-making.
If you’re looking to level up your SaaS revenue forecasting strategy, be sure to download our guide on Sales Forecasting Methods for a deeper dive into best practices and real-world examples.
Why SaaS Sales Forecasting Is Different
Unlike transactional business models, SaaS companies operate on a foundation of recurring revenue. That changes the game for forecasting. In a SaaS business, predicting future sales isn’t just about closing new deals, it’s about understanding the full revenue journey, including customer retention, upselling, and customer behavior over time.
Here’s what sets SaaS sales forecasting apart:
1. Recurring Revenue Focus
In SaaS revenue forecasting, you must consider both new customer acquisitions and existing account renewals. Metrics like monthly recurring revenue (MRR) and annual recurring revenue (ARR) are essential. Forecasts that ignore renewal cycles or customer lifetime value (CLV) often fall short.
2. Churn & Expansion Dynamics
Forecasting sales without factoring in churn and expansion revenue can distort your view of reality. One high-value customer lost can offset multiple new deals. That’s why accurate forecasts must account for both revenue contraction and expansion.
3. Longer Customer Lifecycles
In many SaaS businesses, deals don't deliver full value upfront. Instead, revenue builds gradually across months or even years, especially in enterprise models with long sales cycles. Your forecasting process must account for deferred revenue realization.
4. Onboarding and Ramp Time
Revenue often doesn’t activate immediately. Implementation delays, user onboarding, and internal ramp-up timelines can push real value delivery into future periods. That’s why forecasting models should align with actual sales pipeline and delivery dynamics.
A Holistic Forecasting Approach
To build accurate revenue predictions, SaaS sales teams must consider more than just pipeline deals. Factors like product usage, customer satisfaction, customer success engagement, and historical sales data all play key roles in refining your forecasts.
📈 Want to dive deeper into building a robust strategy? Read our article on how to improve sales forecasting accuracy to learn actionable techniques used by top-performing SaaS teams.
Key Metrics to Track in SaaS Forecasting
Tracking the right key performance indicators (KPIs) is essential for building accurate SaaS sales forecasts. These metrics don’t just show what your SaaS company might close, they help predict what you could retain, lose, or expand. For any data-driven sales forecasting process, these are your must-haves:
Monthly Recurring Revenue (MRR) & Annual Recurring Revenue (ARR)
Your core SaaS revenue forecasting starts here.
MRR = Total monthly subscription revenue
ARR = MRR × 12
These metrics reflect your recurring revenue stream and are vital for financial planning and reporting.
Churn Rate
Churn Rate = Lost MRR ÷ Total MRR at the start of the period
Churn directly impacts future revenue and forecast accuracy. A high churn rate can quickly erode gains from new customer acquisition.
Net Revenue Retention (NRR)
NRR = (MRR from existing customers + expansion – churn) ÷ MRR at the start
A strong NRR signals customer satisfaction and expansion revenue, both critical for sustainable growth in a SaaS business model.
Pipeline Coverage
Pipeline Coverage = Open pipeline ÷ Target quota (per period)
This metric gives insight into whether your sales team has enough sales pipeline data to meet targets. It’s a leading indicator for revenue forecasting.
Sales Cycle Length
The average time from opportunity creation to closed deal.
Understanding your average sales cycle helps forecast sales accurately and allocate resources efficiently.
Lead Velocity Rate (LVR)
LVR = (Qualified leads this month – last month) ÷ last month × 100
This measures the speed at which your pipeline is growing. It’s a forward-looking metric that ties directly to future sales and marketing efforts.
By monitoring these key metrics, SaaS sales leaders can better anticipate market shifts, adjust their sales strategies, and produce accurate revenue predictions.
Need help tracking these KPIs in real time? Book a Forecastio demo to get instant visibility into your pipeline, performance, and forecasts.
Forecasting Methods for SaaS Companies
Choosing the right forecasting models is a key step in building a reliable SaaS sales forecasting strategy. Because of the recurring nature of SaaS revenue, different approaches may be needed depending on your company’s size, sales process, and data quality. Below are some of the most effective sales forecasting methods tailored for SaaS companies:
1. Historical Forecasting
This method relies on historical sales data and trend analysis to predict future sales. It’s ideal for SaaS businesses experiencing steady, predictable growth without major market disruptions.
👉 Best for: Mature products, consistent growth, and minimal churn.
2. Pipeline Stage Forecasting
This model applies stage-based probabilities to open deals in your sales pipeline. While popular, it depends heavily on clean CRM data and accurate stage mapping.
👉 Best for: Sales-led SaaS companies with defined sales cycles and strong CRM discipline.
3. Weighted Pipeline Forecasting
An advanced version of stage-based forecasting that adjusts each deal’s value based on both probability and deal stage. It offers more nuanced predictions of SaaS revenue.
👉 Best for: Teams seeking to align forecasting efforts more closely with reality.
➡ Explore more in our dedicated post on the weighted pipeline.
4. Cohort-Based Forecasting
This approach analyzes revenue trends by customer cohorts (e.g., by signup month, segment, or vertical). It helps forecast churn, upsells, and customer lifetime value with greater granularity.
👉 Best for: SaaS platforms with diverse customer segments or product use cases.
5. Segmented Forecasting
Forecasts different streams like new business, expansion, and renewals separately. This allows for better revenue projection data and strategy per stream.
👉 Best for: SaaS companies with both land-and-expand and enterprise renewal motions.
6. AI/ML Forecasting
AI-powered tools like Forecastio analyze historical data, rep activity, and deal health to deliver more accurate sales forecasting than manual methods.
👉 Best for: Growth-stage and enterprise SaaS companies looking to reduce bias and improve forecast accuracy.
➡ Dive into how AI transforms forecasting in our AI in Sales Forecasting article.
Each method has its strengths, depending on your SaaS business model, sales team size, and the richness of your data. Many modern SaaS sales leaders combine these models to create a robust forecasting model that adapts to market shifts and customer behavior.

Best Practices for SaaS Sales Forecasting
Building accurate sales forecasting processes in a SaaS business isn’t just about choosing the right model, it’s about discipline, structure, and operational consistency. To improve forecast accuracy and ensure your SaaS revenue forecasting stays actionable, implement the following best practices:
1. Forecast by Revenue Type
Separate your forecasts for new business, expansion revenue, and renewals. These revenue streams behave differently and should be forecasted using distinct forecasting models. This is key for accurate monthly recurring revenue and annual recurring revenue tracking.
2. Segment by Sales Motion
Different sales cycles and close rates call for tailored forecasting. Segment your forecast by sales motion. For example, SMB vs. enterprise or inbound vs. outbound. This helps align your sales strategies with actual performance data and improves your ability to predict future sales.
3. Update Forecasts Frequently
In fast-moving SaaS companies, static monthly forecasts fall behind reality. High-growth teams update their sales forecasts weekly or bi-weekly to reflect the latest shifts in the sales pipeline and customer behavior.
4. Enforce Deal Notes & Close Dates
Healthy forecasts rely on accurate pipeline data. Require sales reps to log detailed deal notes and maintain realistic close dates. These small habits improve forecasting efforts dramatically and help sales leaders catch risk signals early.
5. Maintain Clean CRM Data
Your forecasting process is only as strong as your CRM hygiene. Use rules and automation to ensure sales pipeline data stays clean, up to date, and reflective of real opportunities. This supports data-driven financial planning and minimizes surprises.
6. Involve Sales Managers
Sales managers offer frontline insights into deal quality and rep confidence. Their input helps validate or challenge forecast assumptions, improving forecast accuracy across the board. Include them in your review cadence.
Book a demo to see how Forecastio can automate your sales forecasting process, enforce clean data practices, and support your sales leaders in making smarter decisions.
Common Mistakes in SaaS Forecasting
Even with the right forecasting models and tools in place, many SaaS companies still struggle to produce accurate sales forecasts. Why? Because success in SaaS sales forecasting relies as much on discipline as it does on data. Here are some of the most common mistakes that derail otherwise solid forecasting efforts:
1. Blind Trust in Rep Projections
Forecasting based solely on what sales reps “feel” will close—without reviewing deal notes, pipeline stages, or customer behavior—leads to overconfidence and missed targets. Always validate forecasts with sales data and frontline sales manager input.
2. Ignoring Churn and Downgrades
Many forecasts emphasize new sales while ignoring churn, downgrades, or contraction MRR. But in the SaaS business model, lost recurring revenue can easily offset gains. Your SaaS revenue forecasting should always account for both growth and loss.
3. Using Stale Pipeline Data
Outdated close dates, inactive deals, and untouched records distort your forecasts. Clean, timely sales pipeline data is the backbone of forecast accuracy. Automate CRM hygiene where possible.
4. Treating New and Expansion Revenue the Same
New business and expansion revenue behave differently, they have distinct sales cycles, conversion rates, and average deal sizes. Lumping them together in forecasts reduces forecast precision and undermines resource planning.
5. Not Analyzing Forecast vs. Actual
Many teams miss the opportunity to improve by failing to review forecast accuracy post-mortem. Regularly examine past sales performance and measure how forecasts aligned with reality. This helps refine assumptions and build better models over time.
Ultimately, a SaaS sales forecast is only as strong as the operational discipline behind it. Avoiding these pitfalls can significantly improve your ability to predict future sales, manage financial planning, and guide marketing and sales efforts.
Forecasting Tools for SaaS Sales Teams
While manual spreadsheets might work for early-stage SaaS businesses, they quickly become unmanageable as your sales pipeline grows and revenue streams diversify. For scalable, accurate sales forecasting, adopting dedicated forecasting tools is essential.
Here are some popular options SaaS companies should consider:
✅ Forecastio
A purpose-built sales forecasting platform designed specifically for HubSpot users. Forecastio offers forecasting by pipeline stage and revenue stream. It also leverages time series analysis, which is ideal for SaaS companies with short sales cycles and steady growth. This makes it especially effective for mid-sized SaaS teams looking to improve forecast accuracy and track recurring revenue with precision.

🏢 Clari
An enterprise-grade revenue intelligence platform used by larger SaaS companies. It integrates deeply with multiple CRMs and uses AI to track sales rep behavior, deal health, and pipeline trends. Best suited for high-volume, complex sales cycles.
📊 InsightSquared
A strong player for pipeline analytics and sales performance visibility. Offers robust reporting, visual dashboards, and deal-level intelligence to support your forecasting process.
🔧 Salesforce Forecasting
Built into Salesforce CRM, this tool provides basic sales forecasting capabilities. While useful for teams already using Salesforce, it often requires significant customization to match the needs of fast-moving SaaS sales teams.
🧮 Spreadsheets
Still widely used for their flexibility but prone to errors, data decay, and version control issues. Fine for very early-stage teams, but not recommended for mature SaaS revenue operations.
Choosing the right tool depends on your CRM integration, sales team size, data quality, and reporting needs. A modern forecasting model should support recurring revenue, track key metrics, and help allocate resources efficiently based on live sales data.
Real-World Forecasting Example
Let’s bring SaaS sales forecasting to life with a simple example.
Imagine you’re running a growing SaaS startup. Your current monthly recurring revenue (MRR) is $100K. You’re projecting some churn, expecting new deals to close, and your Customer Success team is working on upsell opportunities. Here’s how a real-world forecasting model might look:
📈 Forecast Breakdown
Starting MRR: $100,000
Churn (3%): –$3,000
New Business Expected: +$20,000
Expansion Revenue (CS Pipeline): +$10,000
✅ Forecasted MRR for Next Month: $127,000
This combined view of new, churned, and expansion revenue offers a more realistic picture of your company’s future revenue. It allows you to forecast sales accurately, align marketing and sales efforts, and track the health of your SaaS business model.
This is exactly why separating revenue streams and forecasting each based on actual sales pipeline data and historical trends is a best practice in modern SaaS revenue forecasting.
How to Improve Forecast Accuracy Over Time
Accurate sales forecasting isn’t something you get right on day one, it’s a skill that improves with structure, feedback, and team-wide discipline. For SaaS companies, the key to better forecast accuracy lies in refining both the process and the data that power your forecasts.
Here are proven ways to improve your SaaS sales forecasting over time:
🧹 Invest in CRM Hygiene
Your forecasting model is only as good as your sales pipeline data. Make CRM hygiene a shared responsibility, not just a sales ops task. Ensure sales reps update stages, close dates, and deal notes regularly to avoid forecasting blind spots.
📊 Analyze Forecast vs. Actual
Schedule time each month to compare your forecasted revenue against actual results. This helps your team learn where assumptions were off and adjust future sales forecasting efforts based on real-world performance.
🤖 Use Deal Scoring or AI
Modern SaaS sales forecasting tools offer deal scoring and AI-driven insights to identify at-risk deals and surface hidden trends in your pipeline. These systems use historical data and rep behavior to enhance forecast accuracy.
📅 Hold Weekly Forecast Reviews
Run short, consistent forecast check-ins with your sales managers. These sessions allow leaders to validate assumptions, update timelines, and challenge overconfident projections based on frontline knowledge.
📈 Track Forecast Accuracy as a KPI
Make forecasting accuracy a team-level key performance indicator. When everyone is accountable, your forecasting process becomes more data-driven and trustworthy over time.
The most successful SaaS companies don’t just forecast, they learn from every forecast. Continuous improvement is what transforms your SaaS revenue forecasting from guesswork into a growth asset.
Conclusion
SaaS sales forecasting isn’t about predicting the future with perfect precision, it’s about strengthening your ability to plan, adapt, and scale with confidence.
The best forecasts are built over time, not overnight. Start by choosing the right forecasting method for your stage, ensuring clean sales data, and aligning your forecast with your SaaS business model. With each cycle, you’ll gain more insight, reduce uncertainty, and make smarter, faster decisions for your team and customers.
✅ Keep it simple at the start.
✅ Focus on forecast accuracy, not perfection.
✅ Build a feedback loop and let your forecasting process evolve with your growth.
Done is better than perfect, especially in a fast-moving SaaS company. The sooner you begin, the faster you’ll build forecasting into a powerful asset for financial planning, resource allocation, and revenue predictability.
🚀 Ready to improve your forecasts starting today? Book a demo and let Forecastio help you build a more accurate, scalable, and insightful forecasting engine.
FAQs
How to forecast SaaS sales?
To forecast SaaS sales, start by analyzing your historical sales data, including monthly recurring revenue (MRR), churn rate, and average sales cycle. Choose a forecasting model that fits your SaaS business stage, such as pipeline stage forecasting, cohort-based forecasting, or AI-driven models. Use clean sales pipeline data from your CRM and segment revenue by type (new, expansion, renewals) for better forecast accuracy. Accurate SaaS sales forecasting helps you plan resources, track growth, and make data-driven decisions.
What is the sales cycle in SaaS?
The sales cycle in SaaS refers to the total time it takes to move a prospect from initial contact to becoming a paying customer. It typically includes stages like lead qualification, product demo, proposal, negotiation, and closing. The average sales cycle can vary depending on your customer segment. SMB sales cycles are usually shorter, while enterprise SaaS deals take longer. Understanding your sales cycle is crucial for accurate sales forecasting, resource planning, and optimizing your SaaS business model.
What are the 5 steps of the sales forecasting process?
The sales forecasting process in SaaS typically follows these five steps:
Collect historical sales data. Analyze MRR, ARR, and past sales pipeline data to identify trends.
Choose a forecasting model. Select methods like time series analysis, pipeline forecasting, or AI-based models depending on your stage and data.
Segment your forecast. Break forecasts down by revenue type (new, renewal, expansion) and sales motion (SMB vs enterprise).
Run and review forecasts. Generate projections using your chosen model and compare with real-time pipeline updates.
Refine and track accuracy. Measure forecast accuracy, learn from discrepancies, and continuously improve your forecasting efforts.
What are the 4 principles of forecasting?
The four core principles of forecasting are:
Accuracy depends on data quality. Reliable forecasts require clean, up-to-date sales data and CRM hygiene.
All forecasts are estimates. No forecast is perfect; the goal is to improve forecast accuracy over time through feedback loops.
Shorter time frames = higher accuracy. Forecasts over shorter periods (like monthly or quarterly) tend to be more precise, especially in fast-moving SaaS companies.
Use the right model for your stage. Different forecasting models suit different growth stages and sales motions; choose based on your current business needs and customer behavior.
Share:
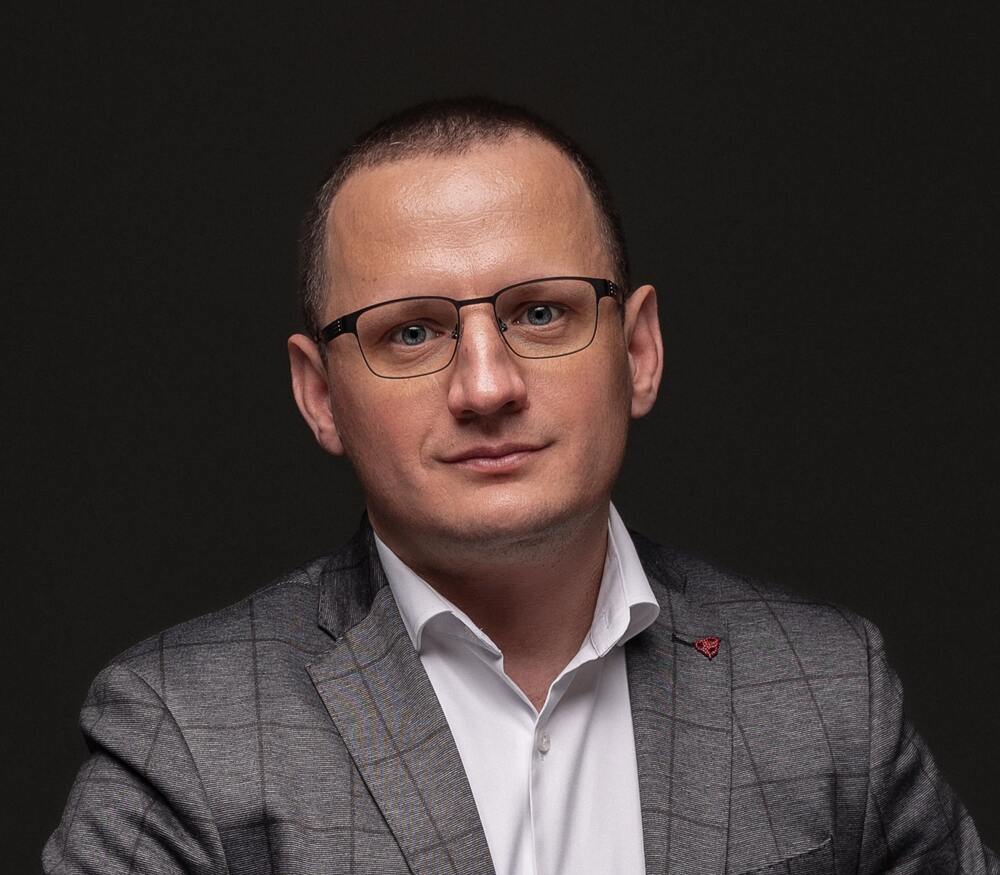
Alex is the CEO at Forecastio, bringing over 15 years of experience as a seasoned B2B sales expert and leader in the tech industry. His expertise lies in streamlining sales operations, developing robust go-to-market strategies, enhancing sales planning and forecasting, and refining sales processes.
Alex is the CEO at Forecastio, bringing over 15 years of experience as a seasoned B2B sales expert and leader in the tech industry. His expertise lies in streamlining sales operations, developing robust go-to-market strategies, enhancing sales planning and forecasting, and refining sales processes.
Related articles
Sales Forecasting
May 13, 2025
11 min
Sales Forecasting
May 13, 2025
11 min
Sales Performance
May 8, 2025
12 min
Sales Performance
May 8, 2025
12 min
Sales Performance
May 2, 2025
13 min
Sales Performance
May 2, 2025
13 min
Sales Forecasting
May 13, 2025
11 min
Sales Performance
May 8, 2025
12 min
Sales Forecasting
May 13, 2025
11 min
Sales Performance
May 8, 2025
12 min
Sales Planning
Sales Forecasting
Sales Performance Insights
Sales Planning
Sales Forecasting
Sales Performance Insights
Sales Planning
Sales Forecasting
Sales Performance Insights
© 2025 Forecastio, All rights reserved.
Sales Planning
Sales Forecasting
Sales Performance Insights
Sales Planning
Sales Forecasting
Sales Performance Insights
Sales Planning
Sales Forecasting
Sales Performance Insights
© 2025 Forecastio, All rights reserved.
Sales Planning
Sales Forecasting
Sales Performance Insights
Sales Planning
Sales Forecasting
Sales Performance Insights
Sales Planning
Sales Forecasting
Sales Performance Insights
© 2025 Forecastio, All rights reserved.
Sales Planning
Sales Forecasting
Sales Performance Insights
Sales Planning
Sales Forecasting
Sales Performance Insights
Sales Planning
Sales Forecasting
Sales Performance Insights
© 2025 Forecastio, All rights reserved.